A mathematical theory of surprise
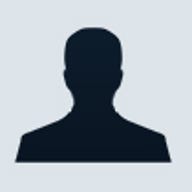
Computer scientists in California have built a mathematical theory of surprise, working from first principles of probability theory applied to a digital environment, according to this news release from the University of Southern California (USC). And the results of experiments recording eye movements of volunteers watching video seem to confirm it. Beyond vision applications, this new Bayesian theory of surprise could lead to new developments in data mining, as it can in principle be applied to any type of data, including visual, auditory or text."
This new mathematical theory of surprise has been developed by Laurent Itti, of the USC's Viterbi School of Engineering, his colleagues at his lab, and by Pierre Baldi, of the University of California Irvine's Institute for Genomics and Bioinformatics.
Before looking at their theory, here are some key definitions given by the computer engineers.
[By analyzing streams of electronic data making up a video image,] researchers can isolate stimuli with visual attributes that are unique in the mix by breaking down the signal into "feature channels," each describing a particular attribute (i.e,, color) in the mix. Such features are called "salient."
A parallel analysis performs similar operations, but does so over time, not space, looking for new elements suddenly appearing. This approach is said to model "novelty."
Finally, an analysis can be done purely in terms of Shannon's original equations, which can measure the level of organization or detail found in the data flow, its entropy.
And now, let's look at their theory.
Their theory boldly proposes to make just such predictions, working from probability theory as well as digital principles. The probability theory involved is that known as "Bayesian," which amounts to a way of structuring events observed over time in the past into predictions about the future.
And they put their theory at work.
The next step is to use this theory to analyze a video stream to describe what are the streams most "surprising," features. Finally, having performed this analysis, they checked it by watching the eye movements observers watching the images, to see if the eyes followed the measure of surprise.
On the figure below, you can see: "(a) Sample eye movement traces from four observers (squares denote saccade endpoints): (b) Our data exhibits high inter-individual overlap, shown here with the locations where one human saccade endpoint was nearby one (white squares), two (cyan squares), or all three (black squares) other humans; (c) A metric where the master map was created from the three eye movement traces other than that being tested yields an upper-bound KL score, computed by comparing the histograms of metric values at human (narrow blue bars) and random (wider green bars) saccade targets. (Credit for image and caption: USC).
The results of this research will be presented at the Nineteenth Annual Conference on Neural Information Processing Systems (NIPS 2005) under the title "Bayesian Surprise Attracts Human Attention."
Here are two links to the abstract and to the full text of this presentation (PDF format, 8 pages, 586 KB), from which the above illustration was picked.
And the U.S. National Science Foundation (NSF) has decided to fund future efforts on this theory, as tells us this other USC news release.
But here are the preliminary conclusions of the authors of this new theory of surprise.
At the foundation of our model is a simple theory which describes a principled approach to computing surprise in data streams. While surprise is not a new concept it had lacked a formal definition, broad enough to capture the intuitive meaning of the term, yet quantitative and computable…. Beyond vision, computable surprise could guide the development of data mining, as it can in principle be applied to any type of data, including visual, auditory or text.
Will we ever see some practical results coming from this theory? Maybe, but in a few years.
Sources: University of Southern California news release, via EurekAlert!November 28, 2005; and various web sites
You'll find related stories by following the links below.