Computers mimicking the brain
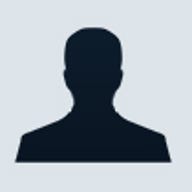
Researchers at the MIT McGovern Institute for Brain Research have used a biological model to train a computer model to recognize objects in busy street scenes, such as cars or people. Their very innovative approach, which combines neuroscience and artificial intelligence with computer science, mimics how the brain functions to recognize objects in the real world. This versatile model could soon be used for automobile driver's assistance, visual search engines, biomedical imaging analysis or robots with realistic vision. It also have many potential applications for neuroscientists, to design augmented sensory prostheses for example. And the researchers are thinking about a commercial implementation of their technology.
This computer model has been built in Tomaso Poggio's laboratory at the McGovern Institute. Poggio also is co-director of the Center for Biological & Computational Learning (CBCL) at MIT where he worked with Thomas Serre. Here is how this biologically-inspired computer model works.
The team "showed" the model randomly selected images so that it could "learn" to identify commonly occurring features in real-word objects, such as trees, cars, and people. In so-called supervised training sessions, the model used those features to label by category the varied examples of objects found in digital photographs of street scenes: buildings, cars, motorcycles, airplanes, faces, pedestrians, roads, skies, trees, and leaves.
Compared to traditional computer-vision systems, the biological model was surprisingly versatile. Traditional systems are engineered for specific object classes. For instance, systems engineered to detect faces or recognize textures are poor at detecting cars. In the biological model, the same algorithm can learn to detect widely different types of objects.
Below are several images which show how this computer model works. The top row contains examples taken from the Street Scene database of the CBCL. The middle row shows the results of true hand-labeling: "color overlay indicates texture-based objects and bounding rectangles indicate shape-based objects. Note that pixels may have multiple labels due to overlapping objects or no label at all (indicated in white)." Finally, the bottom row shows the "results obtained with a system trained on examples like (but not including) those in the second row." (Credit: McGovern Institute at MIT, via IEEE)
Teaching a computer how to recognize objects has always been difficult even if children can easily do it. This is why this new computer model is really innovative because it mimics the brain's own hierarchy.
Specifically, the "layers" within the model replicate the way neurons process input and output stimuli -- according to neural recordings in physiological labs. Like the brain, the model alternates several times between computations that help build an object representation that is increasingly invariant to changes in appearances of an object in the visual field and computations that help build an object representation that is increasingly complex and specific to a given object.
While the team is working on a commercial implementation, it also has ideas to go further.
The lab is now elaborating the model to include the brain's feedback loops from the cognitive centers. This slower form of object recognition provides time for context and reflection, such as: if I see a car, it must be on the road not in the sky. Giving the model the ability to recognize such semantic features will empower it for broader applications, including managing seemingly insurmountable amounts of data, work tasks, or even email.
This research work has been published by the IEEE Transactions on Pattern Analysis and Machine Intelligence under the name "Robust Object Recognition with Cortex-Like Mechanisms" (Volume 29, Number 3, Pages 411-426, March 2007). Here are two links to the abstract and to the full paper (PDF format, 31 pages, 3.48 MB), from which the above images have been extracted.
Finally, the Street Scene database and other ones are directly available as well as various pieces of software from this CBCL page.
Sources: The McGovern Institute at MIT, February 7, 2007; and various other websites
You'll find related stories by following the links below.