High energy: Facebook's AI guru LeCun imagines AI's next frontier
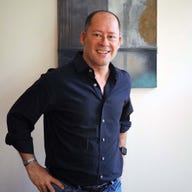
It's been said that engineers -- and some scientists, but mostly engineers -- can visualize in their mind's eye that which does not yet exist long before they sit down at the bench to construct something.
Facebook's head of artificial intelligence, Yann LeCun, seems to fit that profile to a T.
"I work mostly by intuition," he writes in When the Machine Learns, a new book that is part biography, part science lecture, part AI history, published Wednesday in French as Quand la machine apprend.
"I project in my head the borderline cases, that which Einstein called the 'thought experiments'," writes LeCun.
Also: LeCun, Hinton, Bengio: AI conspirators awarded prestigious Turing prize
LeCun is animated on stage, clearly energized by trying to convey things at the edges of AI that have come from his thought experiments.
That ability to imagine something that doesn't exist, perhaps at the limit of what's generally thought feasible, is the mark of engineers and innovators. LeCun is something of a rarity among the AI crowd, a scientist who is at home in algorithm design but also has one foot firmly in computer engineering.
LeCun, who this year won the ACM Turing Award for contributions to computer science, is best known for advancing and refining and making practical the convolutional neural network, or CNN, in the 1990s. He didn't invent the thing from scratch, but he made CNNs practical, workable. They formed the foundation of the revolution in machine learning that brought LeCun to prominence in the past decade along with his compatriots, fellow award winners Geoffrey Hinton and Yoshua Bengio.
LeCun the engineer was on stage Wednesday at the Institute for Advanced Study in Princeton, NJ, to explain what sounded like intuition, but a well fleshed-out intuition. The setting was a three-day workshop on deep learning -- specifically, the theory of deep learning. Organized by Institute mathematics professor Sanjeev Arora, the event drew plenty of AI luminaries, including Nvidia's head of AI research, Anima Anandkumar, and LeCun's fellow Facebook scholar Léon Bottou.
LeCun's slide presentation was about a theme he's lately taken on the road to many lectures: How to get beyond the labeled training examples of conventional deep learning. "We are not going to get intelligence as general as humans' with supervision or with multi-task learning," he told the audience. "We are going to have to have something else."
Primers
That something else, LeCun believes, is unsupervised learning. And to make possible unsupervised learning, the entire field may need to work more on an approach known as energy-based learning.
Energy functions have been around in AI for decades. The biologist John Hopfield first popularized the approach in the 1980s with what came to be known as the "Hopfield Network." It was a breakthrough in machine learning at the time, and it led to other kinds of learning algorithms that deal with notions of an energy field to be optimized, such as the "Boltzmann Machine" pursued by Hinton.
Also: Facebook's Yann LeCun reflects on the enduring appeal of convolutions
"Energy-based learning has been around a while," observed LeCun, "and it's come back recently to my consciousness because of the need to do less supervision."
The details become abstruse quickly, but the basic idea is that instead of creating tons of labeled data sets, such as pictures of cats and dogs, or spending thousands of hours playing games of chess like DeepMind's AlphaZero, just take some abundant raw data, such as lots of YouTube clips, and feed it to the machine.
"Make the machine really big and have it watch YouTube or Facebook Live all day," said LeCun.
The machine can be trained to predict what comes next after each frame of video. The compatibility between a prediction and the reality is what's called an energy level. Lower energy is better, more compatible, meaning, more accurate, so a neural net tries to reach an ideal low-energy state.
Also: Facebook's Yann LeCun says 'internal activity' proceeds on AI chips
LeCun has great energy on stage and an evident delight with the nuances of the subject. He demonstrated uncertainty for the audience, staring straightforward, moving his arms back and forth. "You're looking at me right now, you're shooting a video of me, and the background doesn't change, the camera doesn't move," said LeCun.
"The only thing that happens is that I can move my head in one direction or the other, I can move my muscles in different ways, and the manifold of all pictures of my head during this talk right now is a low-dimensional manifold that is bounded above by the number of muscles in my head."
LeCun's message was fairly radical in the context of machine learning. Energy functions do away with probabilistic prediction, in LeCun's version. "I think the right framework for this is to actually throw away the probabilistic framework because it's wrong." The typical neural net would have to have "infinite weights," he said, which is "just wrong, it's also useless."
Clearly, LeCun is in his element imagining what's not yet been created and trying to communicate it. But some of that would have to wait for another day. LeCun was getting on a plane later to go to Paris to meet with journalists to discuss the book. He is on the cover this week of a glossy French magazine, l'Obs (the Nouvel Observateur) talking about the promise and peril of AI. He is the pied piper of a movement everyone wants to be excited about or frightened about. For decades, LeCun and others could see it, but the stuff didn't work. Now it seems to work too well. Someone's got to be its ombudsmen. That's LeCun.