How do we get to a possible future with AI? Meet the AI Adoption Maturity Curve
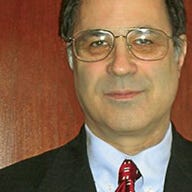
I've known Michael Wu for many years. Many years. He's been a thought leader, an influencer, a true scientist, an architect and always a dear friend, a non-blood member of the family. When I met him, he was chief scientist at Lithium and he was on the verge of becoming an influencer on the nature of influence. He wrote a number of seminal pieces on it and at the same time, starting traveling the world to speak at conferences ranging from the technology industry to the scientific. He and his wife Margie are two of the most delightful and good-hearted people I've had the pleasure of knowing.

For the last several years, Michael has been a thought leader and innovator in the world of AI; in fact, he is currently the Chief AI Strategist at PROS, a company that not surprisingly has a world class AI platform.
Normally, I'm a critic of maturity models that come from vendors because they typically represent the vendor's self interested version of a maturity model. I can go into a long dissertation of why I think it's normally bogus. But this is Michael's show today. His maturity model meets the standard for a universal model that applies to all areas and is not biased to PROS's AI platform. It's a credit to Michael's and to PROS cultural honesty - both are impeccable. So you can treat this maturity model as something you can trust to be what a true AI maturity model looks like. Argue with me if you want. I'm down with that. :-) But I'm publishing it because I trust Michael and PROS and this is a model that needs to be seen - especially now that people are wrestling with AI as a necessity to grow their engagement with their customers and thus their business.
You'll see that in Michael's case the AI has a component not just for business but for good. Make that Capital G Good.
So, with that said...take it away, Dr. Michael Wu!
Most of you probably know me as a data scientist who develops machine learning (ML) algorithms and builds artificial intelligence (AI) solutions. But most of you probably don't know that I am also half a behavior scientist. I wasn't formally trained in behavioral economics or psychology, but I've applied my data science expertise specifically to analyze consumer behaviors on social media for over a decade in my previous roles. And I've discovered many interesting business insights from the digital footprints left behind by digital consumers. Although this doesn't make me a full-blown behavior scientist, with all the writings, lectures, and workshops I contributed to this discipline (some at renowned universities), I think I qualified for half a behavior scientist.
Emerging from this tumultuous 2020, we as a society have learned, adapted, evolved, and continue doing so to better cope with the disruption of the past year. An increasing number of companies are supporting remote, virtual work. Meanwhile, more and more businesses are going digital, and turning their storefront into an eCommerce channel.
This makes me wonder how will the future unfold as we adopt more contactless digital technologies. Certainly, this will greatly accelerate the adoption of AI for two reasons. First, AI is absolutely required to help scale the global nature of your eCommerce channel, since digital is borderless. More importantly, AI is a key differentiator against the steep competition online. Being online greatly increases your total addressable market, but it also elevates your competition significantly, since you'll be competing with every single eCommerce on the planet. So, every digital business must and will eventually adopt some form of AI.
Since many companies are still in the early stage of this AI adoption journey, it is helpful to understand the AI adoption journey. However, all companies are comprised of humans, and therefore subject equally to principles that govern human behaviors. This prompted me to explore whether it is possible to use principles of psychology and behavior economics to predict the future adoption trajectory of AI. It is, and I have developed a vendor-agnostic AI adoption maturity model as a result of this exploration.
This AI adoption maturity model has six stages, and moving from one stage to the next is a direct consequence of human behaviors (i.e. their reactions to the competitive pressures in the market). It is not driven by the use of any particular solution from any vendor. Such a maturity model is extremely useful because it allows you to see where you stand compared to other companies regardless of your choice of vendors or even the specific AI solutions. And if you are one of the early adopters who have already embarked on this journey, this maturity model can also serve as an adoption roadmap that shows you what you can expect in the roads ahead.
AI Maturity Model
Stage 1: Digital Transformation (in Greater Detail)
I call this first stage the digitization of work, because it's literally turning our work in the physical world into digital processes that can be tracked and recorded digitally as data. In the grand scheme of things, this stage is all about getting the data, which is the raw material for AI. Evidently, digitization is usually the first step of digital transformation So if you are on the digital transformation bandwagon, good for you. You are already in Stage 1 of this maturity curve.
By digitizing our work, we would have the data to train AI to mimic how we work. However, the critical mass of data required to train AI is very high, so it typically takes a long time for companies to reach this data volume. While data accumulation takes time, companies can glean value from the data they've captured before reaching the critical mass required by AI. This is accomplished by performing analytics on the existing data to help leaders make better decisions.
Most companies will begin with descriptive analytics that merely summarizes the data they've collected. This is because the data volume required to perform descriptive analytics is relatively low. Yet, this could and already have produced thousands of reports and dashboards powered by business intelligence (BI) to facilitate business decision-making.
As the volume and diversity of your data assets grow, you may be ready to perform predictive analytics. This is where we use existing data assets to make inferences about data we don't have via a model. A classic application is forecasting, where companies use existing historical data to infer future data, which they don't have. But in general, you can use any data asset to infer some other data you want. For example, a manufacturing plant may use the mechanical operation data (assuming this is collected) to infer the failure time of certain machinery (data they don't have) to do predictive maintenance.
Eventually, you would have gathered enough data to perform prescriptive analytics. This is where prescriptive models are developed to prescribe actions that optimize some outcomes. For example, a pricing recommendation system could prescribe the price you should set for a product to optimize revenue. Marketing automation could prescribe the engagement frequency for a prospect to optimize conversion probability. As you progressed from doing descriptive to predictive, and then prescriptive analytics, you would have enough data to move onto the next stage of the maturity curve.
We have gone through this stage in greater detail so you can see how companies naturally progress through this journey in a completely vendor-agnostic way. Regardless of which vendors you've chosen to help you perform the various types of analytics, the progression from descriptive, to predictive, to prescriptive will happen in this order because it's only limited by the data assets accumulated by the company. It's independent of the vendor that provides the analytics. In fact, it's independent of the technologies, and even the use cases. That means it doesn't even matter what you do with these analytics in your business, the progression of will still happen as described.
Although the journey through this first stage of the maturity model is vendor-agnostic, technology-agnostic, and use-case-agnostic, I must emphasize that it is derived from social science principles. Yet these principles are not like the fundamental laws in math, physics, or chemistry that are practically absolute. This means there will be exceptions, albeit rare.
For example, a company can skip doing predictive analytics and go from descriptive analytics to prescriptive directly. Yet, another company may be so focused on using prescriptive analytics to address a specific problem that they've chosen not to do descriptive and predictive analytics even when they had enough data to do so. As I said before, these are the exceptions, not the norm. And companies would have to invest more resources and potentially forgo more value in the short-term to deviate from the norm.
Similarly, the remaining stages of the AI adoption maturity model are also derived from those same principles in behavioral economics and psychology. So they are agnostic to vendors, technologies, use cases, industries, business models, etc. And this maturity model illustrates the normative journey that most businesses will go through as they adopt AI technologies to help their business operate more efficiently and compete more effectively in the market. However, in the interest of time, I will go through the rest of the AI adoption maturity model a bit faster.
Stage 2: Exchange Data for Automation
If you made it here with me, you should have built up a sizable data asset already. So it's time to make the most of your data assets by exchanging this data for automation. And prescriptive analytics provide the natural transition into this stage. After all, if a machine can already prescribe actions that optimize some outcome, why not just automate those actions? If the prescribed actions are indeed "optimal," which means there is nothing else you could've done to achieve a better outcome, why wouldn't you let the machine automate these actions?
If you are in this stage of the AI maturity journey, you would be providing machines with data on how you (humans) make decisions in a certain aspect of your work. This data is used to train the AI (via supervised learning) to mimic how you work. From the AI's perspective, we can equivalently say the AI is learning from you through the data you've provided. Once the AI is trained, it would be able to help us automate that aspect of our work by mimicking our decisions and actions. So essentially, you are exchanging your data for automation.
Here is an illustrative example. Amazon used to have a large team of fraud investigators to examine potentially fraudulent transactions and determine if frauds were committed. Over the years, Amazon has collected huge amounts of data on transactions that were determined by their investigators to be fraudulent, as well as those that are not. This data on what the fraud investigators decide to do for different transactions in different scenarios are used to train a model to mimic the human experts' decisions and actions. When the AI has learned to mimic the human investigators, it would be able to help automate their decisions and actions in the future.
I must clarify the distinction between the prescriptive analytics in Stage 1 and the AI automation in Stage 2 here. In Stage 1, the machine would tell you what to do, but defer the final decision to humans, in case the prescriptive model doesn't have all the data to ensure the optimality of its prescribed actions. In Stage 2, we must be comfortable with letting machines make the call (at least when it's sufficiently confident) under human supervision.
The hallmark of Stage 2 is that machines are allowed to make some decisions and execute the actions it thinks are best. This cuts humans out of the loop, so we typically see a dramatic increase in efficiency. Since this is the first time when machines are allowed to make the final decision, it is the beginning of true AI automation.
Stage 3: Learning from Humans
Despite the huge efficiency gain, however, the AI algorithms in Stage 2 typically can't quite perform at human level yet. So we may need to sacrifice quality temporarily as a tradeoff. Therefore, constant human supervision is still required, and human intervention is still necessary when the AI isn't confident enough about its decision. Now, you may wonder, is there a way to reap the efficiency gain without sacrificing quality?
For this, I have good news for you! This is precisely the issue we will address in Stage 3 of the AI adoption maturity model. In this stage, we (humans) will actively teach the AI to refine its model of how we work. The goal here is to improve the performance of the AI to match human quality work. Essentially, we become a supervisor and a coach of AI. From the perspective of AI, it would be learning from humans.
But wait, didn't we just talked about AI learning from human through the data we provided in Stage 2? What is the difference here? The distinction is subtle, so let me clarify. In the previous stage (Stage 2), the focus is in automating human decisions to gain efficiency. So the learning is focused on dealing with the most common situations (i.e. the most typical 80%). But here (Stage 3), the focus is on closing the performance gap between machine and human. So the learning is focused on dealing with the outliers (i.e. the 20% edge cases).
In this stage, every time the AI helps us automate our work, we would review and rate it. We would need to provide explicit feedback on whether the AI is doing a good job or not. If the AI isn't doing a good enough job, we can take over and perform the task ourselves. Since we can already digitize our work in Stage 1, while we take over the task, the AI will be collecting data on what we do differently, how we do it, and why.
I want to stress that an important characteristic of this stage is the collection and utilization of explicit human feedback data. The AI must learn from this new data to refine and improve its model of how we work through reinforcement learning. Although the AI here is learning to handle a much smaller number of cases (~20% as oppose to ~80%), since these are edge cases that occur rarely, this model refinement is a long-tail process. That is, collecting enough data to cover ~20% of the edge cases may take more time than what's required to collect enough data to cover ~80% of the common cases.
But if we do this long enough, the AI will eventually cover all the outliers with sufficient data. At this time, the AI would have a very fine-tuned model of how we work and can perform at near-human level. This means AI would be able to automate a large part of our work and mimic how we work under nearly all plausible circumstances. The result is that businesses would get improved efficiency and scalability from AI automation without sacrificing quality and personalization.
Stage 4: Full Automation and Job Shift
If you have an AI that can perform human quality work, and in some instances, even surpass humans, what would you do? This where we enter Stage 4 of the maturity model. And I must tell you that the natural thing to do is to let AI take over our task completely with full automation. Since AI is able to automate a large part of our work and do an equally good or better job than us, why wouldn't we let it take over?
You may wonder, for selfish reasons, what do we do then? This is a legitimate concern. However, this situation is really no different from when we have trained an intern or a new employee to do our work.
If we've trained an intern well, and they can perform all our tasks equally well, then we should hire them and let them take over our work. What happens then is that we become managers. We take on more work, different kinds of work, and we take on more responsibilities. The very nature of our job will change dramatically, but our work is shifting to something more strategic and important. That's why I called this stage "full automation and job shift."
If you are one of the few companies that reach Stage 4, you've come a long way. Your AI solutions are pretty autonomous and your use cases are very advanced. It's conceivable that companies in this stage would have human managers, each managing multiple AI-based technologies responsible for many different tasks. Consequently, you would have achieved unparalleled efficiency and unmatched competitive advantage in the market. But this raises a whole new set of challenges relating to HR, accountability, and even legal.
Since your AI can operate with a fair amount of autonomy, who would be accountable if a wrong decision is made. You can't fire an AI as you do with employees. Since these AI help you operate so efficiently, you may not need so many employees? Do you just lay off the extra employees? How can we retrain and reskill these workers, and who is responsible for doing this? You must work through these challenges before you can get through this stage.
So far, the first four stages of the AI adoption journey are generally more focused on the internal operations within a company. There are, however, some external use cases in customer service using conversational AI or chatbots that can start as early as Stage 3. But I cannot recommend letting an AI directly engage with external entities (e.g., customers, partners, or companies) without human supervision when it hasn't achieved human-level performance yet. This is because customer experience is crucially important today. Before AI can replicate or surpass human quality work, it has the potential to create an irreparable customer experience that contributes to the long-term or permanent loss of customers. Trust me, you don't want to play dice on this one.
However, when a company reaches Stage 4, their AI would be good enough to face the external world. So the next two stages are more focused externally, engaging with customers, partners, and other companies.
Stage 5: 'Last-Mile' Delivery
As we move into the fifth stage, this is where we will be delivering the "last mile" of AI automation. This is going to involve robotics and other mechanical machinery powered by autonomous AI. The pervasive use of robotics with autonomous AI is a clear indicator of Stage 5 of the AI adoption maturity model.
Most of the AIs we've dealt with up to Stage 4 are digital in nature. Despite the utility offered by digital platforms, there are a lot of things we can't do digitally yet. I seriously doubt that we will eat a meal digitally in the foreseeable future.
Today, we can find everything we will ever need and configure every aspect of those products to our liking on the web. We can even make the purchase online via eCommerce, but not every product can be downloaded to our laptop or phone. A lot of products still need to be delivered physically, and many services still need to be performed by humans. So, how could companies deliver that "last mile," where the product reaches you and the service is performed?
I want to stress that for companies to enter Stage 5, they would need to start bridging the digital-physical gap and deliver the "last mile" of AI automation. Whether it uses an autonomous drone, robots, other machines, or vehicles powered by autonomous AI, they must be able to execute the delivery of the product or service they provide in the physical world. This could be something as simple as delivering a meal or other physical product. Or it could be the execution of complex physical tasks, like cleaning the windows at your home.
Stage 6: AI-Augmented Economy
If most companies are able to achieve the first five stages, then we will move into the final stage where we'll have an AI-augmented economy. This will be a very different world where most of the repetitive work of our business may be performed by AI and robots. You may ask, is there anything left for humans? Do we still need to work in that world?
The bad news is that humans will still need to work because there are always new problems that need to be solved and new situations that need to be dealt with. Since new problems are never seen before, there is no data on what to do in these situations. So we, humans, will still need to solve these problems a few times, perhaps under different situations and contexts to generate enough data. We may even need to solve them in several different ways to generate sufficient data for the AI to learn to address these problems. In this future, we will be doing more problem-solving that is inherently non-repetitive.
The good news, however, is that these types of work are likely more interesting, more motivating, and they are probably more critical. This will likely have significant societal and economic impacts, as we will likely work less and be able to have more time to focus on things that are more important in our lives.
Humans have dramatically reduced the amount of time they spend working ever since the Industrial Revolution. This is due to the efficiency we gained through the employment of mechanical machines. Now if we can enter Stage 6 of the AI adoption maturity curve, intelligent machines could help businesses operate so efficiently that we can work even less.
Our government may even support a universal basic income, so people may no longer need to work for survival. This allows people to choose what they want to do and work on things they are truly passionate about, leading to greater job satisfaction. I believe this will ultimately drive more innovation, which culminates in greater economic growth.
Conclusion
We are clearly a long way from reaching an AI-augmented economy today, but it all starts with digital transformation, which is already happening. This AI adoption maturity curve provides companies a roadmap for getting to this future. This future won't be a utopia free of problems, but it's a world that could unleash more passionate people than ever to solve some of the most difficult problems in our society. Together with AI, I believe we can tackle the biggest challenges facing humanity.
Thank you, Doc. AKA Michael.
An announcement or two:
CRM Playaz is very happy to announce that we have partnered with MyCustomer.com for content distribution. We have admired them for a long time (one of my favorite venues in the world - along with this one of course) and they us, so we took the mutual admiration to another level and will work together when we can to create and distribute content that has some real value - and, of course, at times, is funny. (At least we'd like to think so and now, MyCustomer.com would like to think so too! One small step...)
My new show, "Engaged...with Paul Greenberg" is set for launch in what looks to be early August. I would appreciate your help in something though. Weirdly (if I'm reading it right) YouTube requires 1,000 subscribers to unlock some of their content creation tools - and even though I'm pre-launch, I'd ask you to subscribe to my show (Not to me, to the show). Here's the link. As of this writing, the only video on the site is my 39-second intro to the actual show. There will be a longer teaser/trailer up possibly by the time you read this. Please watch them and please, please, please subscribe. If you subscribe pre-launch - I will buy you a bagel and lox when I see you. And coffee or tea. And a drink. Or two. And a car. Okay. not a car.
Have a great week.