How our brain sees natural scenes
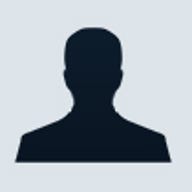
Carnegie Mellon University (CMU) researchers have developed a new computational model explaining how the brain processes images to interpret natural scenes. According to ACM TechNews, the team 'used an algorithm to analyze the patterns that compose natural scenes and determine which patterns are most likely associated with each other.' And the team leader said that they 'were astonished that the model reproduced so many of the properties of these cells just as a result of solving this computational problem.' He added that it's still a theory, so they need to test it further.' Read more...
You can see above how "statistical patterns distinguish local regions of natural scenes: a, A natural scene with four distinct regions outlined (image courtesy of E. Doi). b, The scatter plot shows the joint output of a pair of linear feature detectors (oriented Gabor filters) for 20 20-image patches sampled from the four regions. The outputs from different regions are highly overlapping, indicating that linear features provide no means to distinguish between the regions." Credit: CMU/Nature) Here is a link to a larger version of this figure.
This research project has been led by Michael Lewicki, associate professor in Carnegie Mellon's Computer Science Department and the Center for the Neural Basis of Cognition (CNBC). He worked with his graduate student, Yan Karklin. Here is a page describing the current CNBC research projects.
Let's start with a simple example. "The bark of a tree, for instance, is composed of a multitude of different local image patterns, but the computational model can determine that all these local images represent bark and are all part of the same tree, as well as determining that those same patches are not part of a bush in the foreground or the hill behind it. 'Our model takes a statistical approach to making these generalizations about each patch in the image,' said Lewicki, who currently is on sabbatical at the Institute for Advanced Study in Berlin. 'We don't know if the visual system computes exactly in this way, but it is behaving as if it is.'"
And there are still huge gaps between human brain and computers about vision skills. "The human brain makes these interpretations of visual stimuli effortlessly, but computer scientists have long struggled to program computers to do the same. 'We don't have computer vision algorithms that function as well as the brain,' Lewicki said, so computers often have trouble recognizing objects, understanding their three-dimensional nature and appreciating how the objects they see are juxtaposed across a landscape. A deeper understanding of how the brain perceives the world could translate into improved computer vision systems."
This research work has been reported by Nature as an advance online publication under the title "Emergence of complex cell properties by learning to generalize in natural scenes" (November 19, 2008). Here is the beginning of the abstract. "A fundamental function of the visual system is to encode the building blocks of natural scenes -- edges, textures and shapes -- that subserve visual tasks such as object recognition and scene understanding. Essential to this process is the formation of abstract representations that generalize from specific instances of visual input. A common view holds that neurons in the early visual system signal conjunctions of image features, but how these produce invariant representations is poorly understood. Here we propose that to generalize over similar images, higher-level visual neurons encode statistical variations that characterize local image regions. We present a model in which neural activity encodes the probability distribution most consistent with a given image. Trained on natural images, the model generalizes by learning a compact set of dictionary elements for image distributions typically encountered in natural scenes."
You'll have to pay to read the full paper, but you can freely access several figures excerpted from it and to some supplementary information (PDF format, 12 pages, 275 KB). Previous papers about the same subject are avilable from the researchers, here and there.
Sources: Carnegie Mellon University news release, November 19, 2008; and various websites
You'll find related stories by following the links below.