Machine learning adds punch to predictive analytics
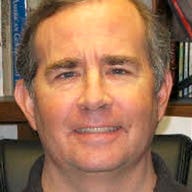

Machine learning techniques generally produce more accurate predictions.
Predictive analytics has become an increasingly important tool for businesses as they look to make better use of all the data they're gathering. Machine learning can provide even more punch to analytics, giving enterprises an even more powerful data resource.
Data analysts are increasingly using machine learning techniques for predictive analytics because they "tend to outperform statistical techniques for prediction problems," said Thomas Dinsmore, an independent consultant and author of Disruptive Analytics.
"When organizations deploy machine learning broadly, they improve the efficiency and effectiveness of business processes," Dinsmore said. "Business gains depend on how and where the organization deploys predictions."
For example, better predictions deployed in an insurance claims process can reduce the rate of fraudulent claims and improve customer satisfaction. In a marketing process, better predictions improve ad targeting, audience selection, and offer optimization. And, in retail store operations, better predictions of in-store foot traffic help the retailer optimize staffing.
"The list of potential applications for predictions in business operations is broad and deep," Dinsmore said.
Machine learning makes it possible to do things that would be impossible otherwise, Dinsmore said. For instance, machine learning makes it possible to estimate storm damage from images of properties or regions, diagnose cancer, or detect the unique "signature" of a computer user.
Machine learning techniques generally produce more accurate predictions, especially when the behavior you're looking to predict is rare, Dinsmore said. They "tend to work better with dirty data and 'wide' data sets -- sets with a very large number of features -- and with unlabeled data," he said.
Machine learning algorithms tend to scale well to large volumes of data, and they are more easily incorporated into large-scale applications.
This year, Dinsmore expects to see more machine learning vendors claiming to offer some degree of automation. "There is a crowded market for 'desktop' predictive analytics accessible to the business user; some existing startups will likely be acquired," he said. "New startups will likely focus on targeted business solutions" in marketing, financial service, healthcare, and security.
"Over the next several years, we will see more widespread use of machine learning, as enterprises pursue digital transformation," Dinsmore said. "Analog-to-digital conversion produces huge volumes of new data, and cheap storage means we can keep data that we used to discard. Machine learning provides a way to identify pattern and structure in this data."
Expanding the number of people in an organization who can apply and use machine learning expedites the process of finding value in the data.
Open-source machine learning engines will become increasingly pervasive, Dinsmore said. "Commercial software vendors are already responding to this challenge in different ways," he said. They will typically focus "on the top of the stack, the end user experience, while the middle and bottom of the analytics stack will increasingly become open source," he said.
Whether in the public cloud, private cloud, virtual private cloud, or through on-premises data center virtualization, predictive analytics and machine learning are a natural fit for an "elastic" computing model, Dinsmore said. Much more so, for example, than data warehousing or business intelligence.
"That means vendors like AWS, Microsoft, and Google will carve out a presence in machine learning alongside the traditional analytics leaders," Dinsmore said.
VIDEO: Tesla's Elon Musk launches startup to plug computers into the brain