Tech
What happened to Turing's thinking machines?
Artificial intelligence is still a long way from delivering the human intelligence in robot form that has long been common in science fiction.
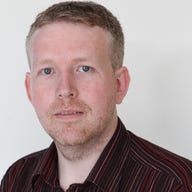

Alan Turing Image credit: Wikipedia
Why what robots find hard, we find easy – and vice versa
Since the field of AI got underway in the 1950s researchers have realised there is a gulf between what humans and what computers find difficult, says Dr Peter Norvig, director of research at Google and co-author of one of the standard works on AI. ”What we thought was hard, things like playing chess, turned out to be easy, and what we thought was easy, things like recognising faces or objects, which a child can do, that turned out to be much harder,” he says. Computers excel at working out the best move in a game like chess because it has well-defined rules and established patterns of play that can be rapidly checked by a machine. The problem with getting computers to emulate human intelligence is that they need to be capable of interacting with the world, of tasks such as recognising people or spoken language, which requires them to handle variables that are constantly changing and hard to predict. In the 1980s AI researchers realised they needed to take a different approach if machines were going to understand that real world complexity, he says. “Part of that shift was from [focusing on] the abstract and formal rules of a game like chess to the messy real world, and going along with that is a shift from formal logic where everything is boolean, true or false, to probability where everything is uncertain,” Norvig says. Judea Pearl, professor in the computer science department at University of California, Los Angeles says that computers are now able to handle aspects of real-world uncertainty through mathematical models that apply Bayesian probability theory to as many as 100,000 variables. ”Now we understand how to cope with uncertainty, because we are armed with all the guarantees and the warning signs that mathematics gives you,” he says. Perhaps the most high profile example of how well modern computers can handle the messy environment of the real-world is how Google's driverless cars have safely navigated more than 20,000 miles of rural and city roads or the wide-ranging speech and language recognition capabilities of Apple's virtual assistant Siri. Future breakthroughs in handling uncertainty, says Pearl, will afford AI routines a far greater understanding of context, for instance providing with the next generation of virtual assistants with the ability to recognise speech in noisy environments and to understand how the position of a phrase in a sentence can change its meaning. But it is true that progress in the field of AI has been more reliant on advances in theory than increases in computer processing power and storage. Norvig says: “There's a minimum floor that if you don't have the computing power you're not going to succeed, but just having more doesn't mean you're going to get somewhere. ”There are a couple of billion computers in the world and we do have enough computing power today if we pooled all of our resources to far outstrip a brain, but we don't know how to organise it to do anything with it. It's not just having the power, it's knowing what to do with it.” And without insightful mathematical modelling, Pearl says, certain tasks would be impossible for AI to carry out, as the amount of data generated would rapidly scale to a point where it became unmanageable for any forseeable computing technology. The importance of theoretical breakthroughs, to some extent, undermines the theory put forward by Ray Kurzweil and others that mankind is decades away from creating a technological singularity, an AI whose general intelligence surpasses our own. Exponents of the theory use the exponential growth of computer processing power as an indicator of the rate of progress towards human-level AI. Norvig is sceptical about predictions that a technological singularity will be created before 2050: “I really object to the precision of nailing it down to a decade or two. I'd be hard pressed to nail it down to a century or two. I think it's farther off.”AI and robots
While we may not be on the cusp of developing intelligences greater than our own, we are likely not far off an explosion in robotics driven by advances in AI, similar to way home PCs suddenly took off in the 1980s. ”Look at progress in speech recognition, machine translation, computer vision, computer planning and operations," says Norvig, adding that the error rate in these areas is roughly halving every decade. Thanks to progress in these sub-fields of AI, autonomous systems are being built that can interact with and learn about their environment, as well as making decisions that aid both themselves and humans. Professor Nick Jennings is a chief scientific advisor to the UK government and heads the Agents, Interaction and Complexity Group in the computer science department of Southampton University. “It [the field of AI] has really come back again to the idea of constructing whole intelligent things. Not in the general intelligence area, as in constructing something of human-like intelligence, but as in autonomous systems,” says Jennings, pointing to the Google Driverless Car, which brings together research in many areas such as sensors, information processing, reasoning and planning. Norvig predicts a boom in the popularity of robots and portable virtual assistants that utilise new UIs, such as the speech recognition of Siri and the augmented reality display of Google's Project Glass. “We will see a lot happen in the next couple of decades. Now everybody is carrying a computer with them that has a phone, has a camera and interacts with the real world. People are going to want to have more of a partnership where they tell the phone something and the phone tells them something back, and they treat it more of a personality. ”In terms of robotics we're probably where the world of PCs were in the early 1970s, where you could buy a PC kit and if you were an enthusiast you could have a lot of fun with that. But it wasn't a worthwhile investment for the average person. There wasn't enough you could do that was useful. Within a decade that changed, your grandmother needed word processing or email and we rapidly went from a very small number of hobbyists to pervasive technology throughout society in one or two decades. ”I expect a similar sort of timescale for robotic technology to take off, starting roughly now.”AI and humans – working together?
The collaboration between humans and intelligent computer systems, which can interact with the real-world and make decisions for themselves, is also what Jennings sees as the most likely future for AI. At Southampton he is working on a research project called Orchid, where networked AI systems called agents work with humans to help make decisions. Researchers working on the project, which runs until 2015, are examining how this approach could be used in a number of scenarios, including disaster response. Orchid will test how AI agents capable of planning, reasoning and acting can help emergency services and others on the ground to react to a rapidly changing situation. The AI agents will scrutinise data from a number of sources and negotiate with other agents and humans to decide the best response, for instance which fire should be extinguished first. Jennings feels this collaborative approach is the best way forward for AI, as while machines already outperform humans in certain areas, such as their ability to parse a trillion web pages in a blink of an eye, he says they will never surpass humans in every field of endeavour. ”As to constructing a system that is generally better than humans in all dimensions, I don't think that it's going to happen. I just think that there are things that humans are innately good at, like creativity. As a human being I feel reassured by that,” he says.Barriers to the creation of thinking machines?
There could also be limitations to the abilities of AI that people are unaware of due to our limited understanding of how the human brain works. For instance, as we discover more about the brain we could discover that some of its critical faculties are tied to its structure and size, limiting our ability to create a superior artificial alternative. “We don't know what level of understanding is going to come out and what are the limitations,” says Norvig. ”If the human brain could have 1,000 times more brain cells, would it work at all? Would it work completely differently? Or work the same but have more?”- How Alan Turing ushered in modern computing (photos)
- Sign the e-petition for Alan Turing to replace Darwin on £10 note
- Breaking the Nazi's Enigma codes at Bletchley Park (photos)
- ZDNet UK: Alan Turing: The computing pioneer's life and works, in photos
- Alan Turing: 10 ideas beyond Enigma
- All aboard computing's 'learning age'?
- SmartPlanet: Turning artificial intelligence on itself
Nick Heath is chief reporter for TechRepublic UK. He writes about the technology that IT-decision makers need to know about, and the latest happenings in the European tech scene.