To master artificial intelligence, don't forget people and process
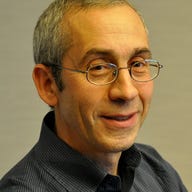
Video: The ethics that AI will need to succeed
Depending on your perspective, the world of artificial intelligence (AI) is blessed or cursed by an embarrassment of riches.
Unlike the bad old days of the AI winter, we not only have the algorithms, but also the data and the infrastructure to make AI and ML happen. And we've lowered the barriers to entry. With the cloud, you no longer need to work for an organization that can afford its own high-performance computing (HPC) cluster, and thanks to open source, you no longer need to reinvent the wheel when it comes to grinding out the algorithms. And thanks to emergence of curated cloud services, incorporating machine learning can be as easy as building an app in Visual Studio.
Read also: Moneyball for movies: Data science and AI in Hollywood
So, what can go wrong? There's been no lack of warnings, from the sobering to the sublime.
Like any technology, you can always get lucky once. You can run an ad hoc project and score some breakthrough insight. That new next-best offer algorithm just increased conversions by 10%. Repeating that feat means paying attention to the people and process side of it.
It starts with building the team. That will clearly be easier said than done. PwC forecasts that there are 3 million practitioners with analytics skills in the US talent pool, but that's a broad superset that extends to data engineering and visualization. Yes, there are millions of analytics specialists out there. But demand is going to outstrip supply for a long time to come, no matter how fast colleges and universities turn out data science grads.
What's interesting is how PwC characterizes the skills mix; it assumes that data scientists must be all-around superstars with advanced skills in everything from domain knowledge to visualization, data governance, engineering, data sourcing, analytics, and machine learning. Let's be realistic -- in most cases, you'll need a team with complementary mixes of those skills as it's unlikely you'll find them embodied in a single person.
If your organization has managed to assemble the right mix of people, that's where process comes in. Chances are, most organizations that are seeking to benefit from AI and ML already have experience working with analytics; hopefully there is some process for identifying analytic problems and operationalizing answers there, although in most organizations it's probably informal if anything exists at all,
But AI and ML add new variables to a process that must begin with data science. The science comes first, and in scenarios where the goal is identifying patterns, such as identifying case and effect, AI might not necessarily be needed.
There are common threads between successful implementation of data science and that for AI. Both are team sports.
But for AI, the stakes (and the risks) are arguably higher. With data science, the decisions rest on people. AI adds another set of moving parts: the system. Through models and algorithms, the system scales the ability of people to spot signals in data that are then used for deriving insights and making decisions that are supposed to drive business benefits. With AI, more of the learning, and in many cases, decision-making load falls to machines. Regardless of whether the machine or deep learning approach is supervised or unsupervised, humans must be part of the equation.
There are numerous variables when choosing the approach. It starts with an embarrassment of riches of open source machine learning and deep learning frameworks to choose from, which makes choosing the right tool for the job challenging to say the least. Often the choice may rely on the types of skillsets that your organization has onboard; for instance, if R is the lingua franca, there will likely be a bias toward the CRAN libraries; if your team prefers Python, the Scikit-Learn will likely be the choice. If your analytics use Spark, there will be a tendency to fold in MLlib to your data processing pipelines.
Then there is the logical side of the model and the purpose (or intent). Do we know what the problem is that we're looking for, or do we need the machine to help us spot it? What are the criteria that we use for assigning features and parameters to the model, and are we inadvertently building in some faulty preconceptions or biases? Are we creating more Minority Reports? Documenting the rationale and assumptions of machine learning and deep learning models is still new ground..
Then there's the decision point for how to train the models; should it be supervised (where humans provide the assumptions) or unsupervised (where the machine is left to sort through and figure out what to learn), or something in between? And what is the criteria for determining when the model is adequately trained?
Agility is key. The team must be prepared to fail fast; data science and AI are both about constant validation of hypotheses. Because of the iterative nature of machine learning, models must be constantly scored and compared. Disruptions or outlier events may either prove or throw off the model. Attention must also be drawn to selection of the data sets. And even once the best algorithms and data sets are identified, there is the phenomenon of drift: data sets, coming from diverse sources, are not always predictable. The characteristics of data (like log files) can readily mutate. And perturbations in data can easily set machine learning and deep learning models off course. Teams should have a codified way for detecting and responding to data and model drift.
Read also: Death and data science: How machine learning can improve end-of-life care
A lot of attention has been directed toward the process of transitioning from model development to deployment; there are numerous tools that help guide the technology side of the process. But what about ingraining the model into the business? Recall when we spoke of documenting intent before? This is where the rubber meets the road. Machines may help make assumptions, spot patterns, or even make decisions. It's not inconceivable that in the future, there will be some new regulatory requirement to document the assumptions or intents behind a model, and how and why the model evolved.
At this point, AI and its manifestations in machine learning and deep learning are novelties; they are the shiny new objects of technology. The first part of the challenge is operationalizing AI; having the right people and having the processes in place are essential if AI is to get ingrained into the business. Repeatability is a big hurdle. But the next step is avoiding becoming a victim of your own success, and that's where accountability comes in. That's where AI becomes a people and process challenge, where natural intelligence becomes ultimately accountable for the results coming from the artificial side.
Previous and related coverage
Alexa, shut up: Amazon tries to stifle spontaneous laughter
Amazon on Wednesday said it's making some changes to its AI-powered virtual assistant after customers reported unprompted laughter from Alexa-enabled devices.
IBM's new launch: PAIRS Geoscope aims to be search for geospatial big data
IBM wants enterprises to use its PAIRS Geoscope datasets to develop better geospatial services.