How to build a data science team
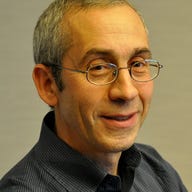
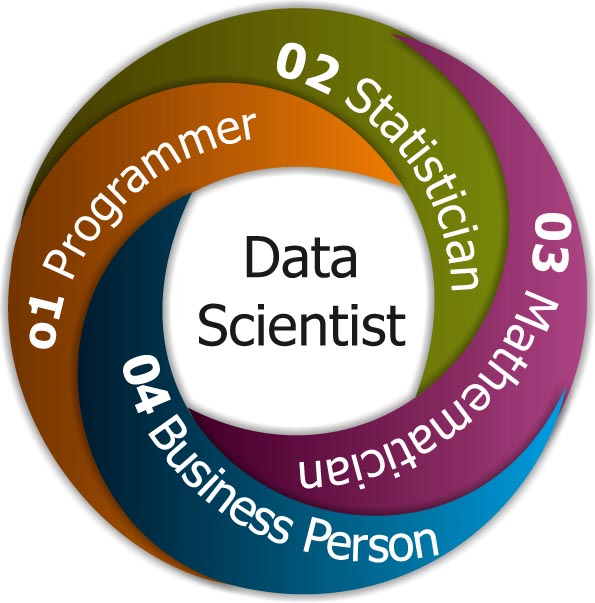
There is good reason that Googling the phrase "Data science is a team sport" brings up so many entries. The skills that are required of a data scientist are so varied that it would be practically impossible to find them in a single person.
And even if you could find somebody that embodies all those qualities, you will likely have to pay top dollar for them. But let's assume that you are not in a large market like North America, and your search grows even more impossible. That was the challenge faced by Vodafone NZ (New Zealand) when analytics and data strategy manager David Bloch had to build a data science team. He presented his saga before the Teradata Partners conference this week.
In a country of less than 5 million people, it shouldn't be surprising that the national telecom provider would likely be the one with the largest Hadoop cluster and most ambitious big data analytics program in town. According to Bloch, the traditional HR approach for finding specialists with years of experience just wouldn't work when you're in a small market. "They would probably filter out the people you want to talk to," Bloch said.
Instead, he called for adopting a startup mentality, combing events like meetups and hackathons for people whose interest and enthusiasm outweighed their actual experience. In place of traditional interviews, a more informal process was the best way to find these people. Bloch had a good idea of what he was talking about given his experience with several data-related startups prior to joining Vodafone.
Bloch defined a series of roles for populating his data science team, encompassing engineers, hackers, analyst, statistician, story teller, and change agent. The roles were not necessarily mapped to individual positions; for instance, the analyst and change agent, or the hacker and engineer, could be the same person.
More specifically, the engineer is the team's "automation magician." As someone who comes with a DBA or ETL background, this is the person who works with the hacker to build data flows, and ensures that technically, the trains run on time. On many teams, this would be called the data engineer. The hacker is the R or Python developer who builds the rough model, even if he or she does not necessarily understand the science behind the model. The latter is the job of the statistician, the deep thinker who owns the scientific method for identifying and validating models. This is probably the person most likely to have "data scientist" on his or her business card.
Then you need someone who is the subject matter expert and data explorer: that is the analyst, who performs as "the Indiana Jones' of the team. This is the person who is comfortable writing SQL and would most closely resemble the business analyst. Finally, there is the story teller, who has the creative streak (and probably a talent for working with visualization tools like Tableau), and the change agent. The person holding the change agent role acts as the influencer who builds the business cases, liaises with executives, and ensures that the models connect and impact business processes. According to Bloch, the change agent is the role that many data science teams often overlook.
Making it all work requires consistent process that, at different stages, involves different members of the team. First, the business challenge must be identified, a task involving the change agent and analyst. Then comes exploration and ideation, where the strategy for getting the insight is formed; that is where the analyst and hacker put their heads together. That is followed by prospecting for data, where the engineer, analyst, and statistician get involved. Now it's time to test and develop the model itself, where the statistician, analyst, and change agent collaborate. The home stretch comes with telling the story, involving (not surprisingly) the story teller and analyst, followed by making the results actionable. Here, the change agent and hacker collaborate to ensure that the results of the model actually get absorbed, and hopefully change the business.
While the team has multiple roles, you don't want it to grow too large. From his startup days, he's found that a dozen people becomes the practical upper limit, beyond which collaboration efforts get unwieldy. But in a pinch, he's seen this model work with as few as two or three people. Taking a Moneyball-like approach, don't get hung up by paper qualifications; aptitudes and enthusiasm go much farther in a labor market where you may have to improvise.