Databricks announces data integration partner program, touting "data lakehouse" model
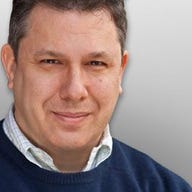
Databricks, the company founded by the creators of Apache Spark, is today announcing its new Data Ingestion Network program. The (perhaps awkwardly-named) partner program brings third party data integration, DataOps, integration Platform as a Service (iPaaS) and change data capture providers onto the company's Unified Data Analytics Platform.
Also read: Is Databricks vying for a full analytics stack?
A well-honed guest list
Bharath Gowda, Databricks' VP of product marketing, briefed ZDNet on the news by phone. He said Data Ingestion Network charter members include Fivetran, Qlik (with its Data Integration product, formerly Attunity), Infoworks, StreamSets and Syncsort. Databricks says Informatica, customer data platform Segment and Talend's Stitch Data Loader will join the program soon. And Gowda pointed out that Microsoft, which offers Azure Databricks as a first-party service, has already integrated it with Azure Data Factory.
Apache Spark, on which Databricks' platform is based, excels at streaming and batch analytics, as well as machine learning and more code-oriented data engineering work. But neither open source Spark nor the commercial Databricks platform are focused on visual data pipeline authoring or the full range of connectors necessary to move data from enterprise SaaS applications. Competing data warehouse platforms like Snowflake and Amazon's Redshift, meanwhile, have been forging prolific partnerships with data integration providers. The Data Ingestion Network will let Databricks compete robustly with those warehouse platforms.
A warehouse with a lakefront view?
And speaking of data warehouses, Databricks' CEO, Ali Ghodsi, feels strongly that running those separately from data lake platforms leads to "siloed data...slow processing and partial results that are too delayed or too incomplete to be effectively utilized." That's why Databricks is heavily pushing the (perhaps better-named) "data lakehouse," its concept for a converged data lake/data warehouse platform.
The suitability of Spark as a data lake is rather undisputed. But Databricks believes the Delta Lake component of its platform, with its ACID transactions and strong consistency, makes it great for warehouse workloads as well. Combine that with its machine learning capabilities, including Spark MLlib and MLflow, and Databricks see itself as a comprehensive platform for analytics and AI, with the Data Ingestion Network clinching it.
Also read: AI gets rigorous: Databricks announces MLflow 1.0
Also read: Databricks introduces MLflow Model Registry, brings Delta Lake to Linux Foundation
Individual, indivisible
Data lakes and data warehouses are distinct constructs, each with great merit. The lake is a great place for exploratory analytics on less-processed data, while the warehouse works well for the kind of operational analytics on highly structured data that enterprises have done there for decades. But just because the constructs are distinct doesn't necessarily mean the platforms needs to be.
Data warehouses combine columnar storage, in-memory operation and parallel processing across clusters of servers to get the job done. Spark and Databricks -- through a combination of the Parquet file format, core Spark and Spark SQL -- replicate much of this, even if implementations differ. Adding third party data integration platforms may check off the last box.