Nvidia boosts its 'superchip' Grace-Hopper with faster memory for AI
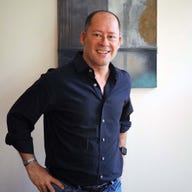
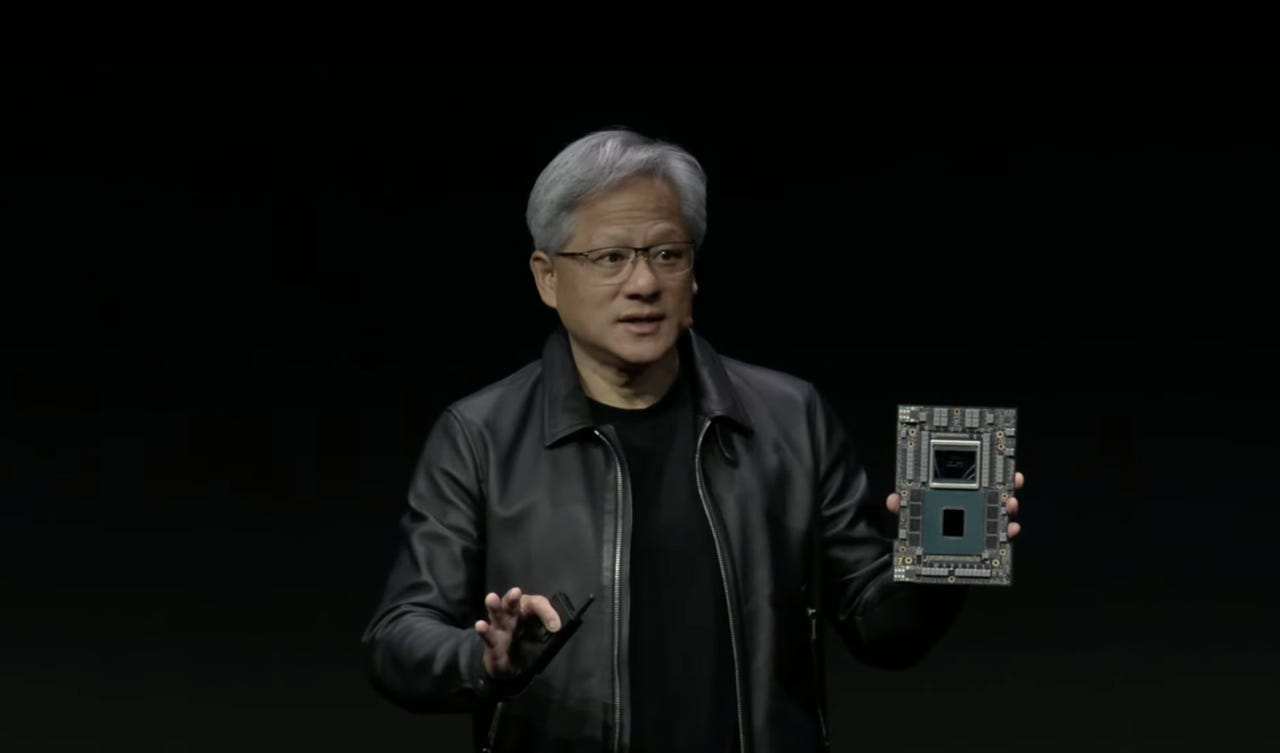
Nvidia CEO Jensen Huang on Tuesday showed off his company's next iteration of the combination CPU and GPU, the "GH200" Grace Hopper "superchip." The part boosts the memory capacity to 5 terabytes per second to handle increasing size of AI models.
Nvidia plans to ship next year an enhanced version of what it calls a "superchip" that combines CPU and GPU, with faster memory, to move more data into and out of the chip's circuitry. Nvidia CEO Jensen Huang made the announcement Tuesday during his keynote address at the SIGGRAPH computer graphics show in Los Angeles.
The GH200 chip is the next version of the Grace Hopper combo chip, announced earlier this year, which is already shipping in its initial version in computers from Dell and others.
Also: Nvidia unveils new kind of Ethernet for AI, Grace Hopper 'Superchip' in full production
Whereas the initial Grace Hopper contains 96 gigabytes of HBM memory to feed the Hopper GPU, the new version contains 140 gigabytes of HBM3e, the next version of the high-bandwidth-memory standard. HBM3e boosts the data rate feeding the GPU to 5 terabytes (trillion bytes) per second from 4 terabytes in the original Grace Hopper.
The GH200 will follow by one year the original Grace Hopper, which Huang said in May was in full production.
"The chips are in production, we'll sample it at the end of the year, or so, and be in production by the end of second-quarter [2024]," he said Tuesday.
The GH200, like the original, features 72 ARM-based CPU cores in the Grace chip, and 144 GPU cores in the Hopper GPU. The two chips are connected across the circuit board by a high-speed, cache-coherent memory interface, NVLink, which allows the Hopper GPU to access the CPU's DRAM memory
Huang described how the GH200 can be connected to a second GH200 in a dual-configuration server, for a total of 10 terabytes of HBM3e memory bandwidth.
GH200 is the next version of the Grace Hopper superchip, which is designed to share the work of AI programs via a tight coupling of CPU and GPU.
Upgrading the memory speed of GPU parts is fairly standard for Nvidia. For example, the prior generation of GPU -- A100 "Ampere" -- moved from HBM2 to HBM2e.
HBM began to replace the prior GPU memory standard, GDDR, in 2015, driven by the increased memory demands of 4K displays for video game graphics. HBM is a "stacked" memory configuration, with the individual memory die stacked vertically on top of one another, and connected to each other by way of a "through-silicon via" that runs through each chip to a "micro-bump" soldered onto the surface between each chip.
AI programs, especially the generative AI type such as ChatGPT, are very memory-intensive. They must store an enormous number of neural weights, or parameters, the matrices that are the main functional units of a neural network. Those weights increase with each new version of a generative AI program such as a large language model, and they are trending toward a trillion parameters.
Also: Nvidia sweeps AI benchmarks, but Intel brings meaningful competition
Also during the show, Nvidia announced several other products and partnerships.
AI Workbench is a program running on a local workstation that makes it easy to upload neural net models for the cloud in containerized fashion. AI Workbench is currently signing up users for early access.
New workstation configurations for generative AI, from Dell, HP, Lenovo, and others, under the "RTX' brand, will combine as many as four of the company's "RTX 6000 Ada GPUs," each of which has 48 gigabytes of memory. Each desktop workstation can provide up to 5,828 trillion floating point operations per second (TFLOPs) of AI performance and 192 gigabytes of GPU memory, said Nvidia.
You can watch the replay of Huang's full keynote on the Nvidia website.