AI ethics toolkit updated to include more assessment components
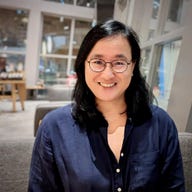

A software toolkit has been updated to help financial institutions cover more areas in evaluating their "responsible" use of artificial intelligence (AI).
First launched in February last year, the assessment toolkit focuses on four key principles around fairness, ethics, accountability, and transparency -- collectively called FEAT. It offers a checklist and methodologies for businesses in the financial sector to define the objectives of their AI and data analytics use and identify potential bias.
Also: These 3 AI tools made my two-minute how-to video more fun and engaging
The toolkit was developed by a consortium led by the Monetary Authority of Singapore (MAS) that compromises 31 industry players, including Bank of China, BNY Mellon, Google Cloud, Microsoft, Goldman Sachs, Visa, OCBC Bank, Amazon Web Services, IBM, and Citibank.
The first release of the toolkit had focused on the assessment methodology for the "fairness" component in the FEAT principles, which included automating the metrics assessment and visualization of this principle.
The second iteration has been updated to include review methodologies for the other three principles, as well as an improved "fairness" assessment methodology, MAS said. Several banks in the consortium had tested the toolkit.
Available on GitHub, the open-source toolkit allows for plugins to enable integration with the financial institution's IT systems.
Also: Six skills you need to become an AI prompt engineer
The consortium, called Veritas, also developed new use cases to demonstrate how the methodology can be applied and offer key implementation lessons. These included a case study involving Swiss Reinsurance, which ran a transparency assessment for its predictive AI-based underwriting function. Google also shared its experience applying the FEAT methodologies to its fraud detection payment systems in India and to map its AI principles and processes.
Veritas also released a whitepaper outlining lessons shared by seven financial institutions, including Standard Chartered Bank and HSBC, on the integration of the AI assessment methodology with their internal governance framework. These include the need for a "responsible AI framework" that spans geographies and a risk-based model to determine the governance required for the AI use cases. The document also details responsible AI practices and training for a new generation of AI professionals in the financial sector.
MAS Chief Fintech Officer Sopnendu Mohanty said: "Given the rapid pace of developments in AI, it is critical financial institutions have in place robust frameworks for the responsible use of AI. The Veritas Toolkit version 2.0 will enable financial institutions and fintech firms to effectively assess their AI use cases for fairness, ethics, accountability, and transparency. This will help promote a responsible AI ecosystem."
Also: AI has the potential to automate 40% of the average work day
The Singapore government has identified six top risks associated with generative AI and proposed a framework on how these issues can be addressed. It also established a foundation that looks to tap the open-source community to develop test toolkits that mitigate the risks of adopting AI.
During his visit to Singapore earlier this month, OpenAI CEO Sam Altman urged the development of generative AI alongside public consult, with humans remaining in control. He said this was essential to mitigate potential risks or harm that might be associated with the adoption of AI.
Altman said it also was critical to address challenges related to bias and data localization, as AI gained traction and the interest of nations. For OpenAI, the brainchild behind ChatGPT, it meant figuring out how to train its generative AI platform on datasets that were "as diverse as possible" and that cut across multiple cultures, languages, and values, among others.