Artificial intelligence and privacy engineering: Why it matters NOW
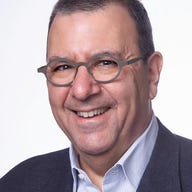
As artificial intelligence proliferates, companies and governments are aggregating enormous data sets to feed their AI initiatives.
Although privacy is not a new concept in computing, the growth of aggregated data magnifies privacy challenges and leads to extreme ethical risks such as unintentionally building biased AI systems, among many others.
Privacy and artificial intelligence are both complex topics. There are no easy or simple answers because solutions lie at the shifting and conflicted intersection of technology, commercial profit, public policy, and even individual and cultural attitudes.
Given this complexity, I invited two brilliant people to share their thoughts in a CXOTALK conversation on privacy and AI. Watch the video embedded above to participate in the entire discussion, which was Episode 229 of CXOTALK.
Michelle Dennedy is the Chief Privacy Officer at Cisco. She is an attorney, author of the book The Privacy Engineer's Manifesto, and one of the world's most respected experts on privacy engineering.
David Bray is Chief Ventures Officer at the National Geospatial-Intelligence Agency. Previously, he was an Eisenhower Fellow and Chief Information Officer at the Federal Communications Commission. David is one of the foremost change agents in the US federal government.
Here are edited excerpts from the conversation. You can read the entire transcript at the CXOTALK site.
What is privacy engineering?
Michelle Dennedy: Privacy by Design is a policy concept that was hanging around for ten years in the networks and coming out of Ontario, Canada with a woman named Ann Cavoukian, who was the commissioner at the time of Ontario.
But in 2010, we introduced the concept at the Data Commissioner's Conference in Jerusalem, and over 120 different countries agreed we should contemplate privacy in the build, in the design. That means not just the technical tools you buy and consume, [but] how you operationalize, how you run your business; how you organize around your business.
And, getting down to business on my side of the world, privacy engineering is using the techniques of the technical, the social, the procedural, the training tools that we have available, and in the most basic sense of engineering to say, "What are the routinized systems? What are the frameworks? What are the techniques that we use to mobilize privacy-enhancing technologies that exist today, and look across the processing lifecycle to build in and solve for privacy challenges?"
And I'll double-click on the word "privacy." Privacy, in the functional sense, is the authorized processing of personally-identifiable data using fair, moral, legal, and ethical standards. So, we bring down each one of those things and say, "What are the functionalized tools that we can use to promote that whole panoply and complicated movement of personally-identifiable information across networks with all of these other factors built in?" [It's] if I can change the fabric down here, and our teams can build this in and make it as routinized and invisible, then the rest of the world can work on the more nuanced layers that are also difficult and challenging.
Where does privacy intersect with AI?
David Bray: What Michelle said about building beyond and thinking about networks gets to where we're at today, now in 2017. It's not just about individual machines making correlations; it's about different data feeds streaming in from different networks where you might make a correlation that the individual has not given consent to with [...] personally identifiable information.
For AI, it is just sort of the next layer of that. We've gone from individual machines, networks, to now we have something that is looking for patterns at an unprecedented capability, that at the end of the day, it still goes back to what is coming from what the individual has given consent to? What is being handed off by those machines? What are those data streams?
One of the things I learned when I was in Australia as well as in Taiwan as an Eisenhower Fellow; it's a question about, "What can we do to separate this setting of our privacy permissions and what we want to be done with our data, from where the data is stored?" Because right now, we have this more simplistic model of, "We co-locate on the same platform," and then maybe you get an end-user agreement that's thirty or forty pages long, and you don't read it. Either accept, or you don't accept; if you don't accept, you won't get the service, and there's no opportunity to say, "I'm willing to have it used in this context, but not these contexts." And I think that means Ai is going to raise questions about the context of when we need to start using these data streams.
How does "context" fit into this?
Michelle Dennedy: We wrote a book a couple of years ago called "The Privacy Engineer's Manifesto," and in the manifesto, the techniques that we used are based on really foundational computer science.
Before we called it "computer science" we used to call it "statistics and math." But even thinking about geometric proof, nothing happens without context. And so, the thought that you have one tool that is appropriate for everything has simply never worked in engineering. You wouldn't build a bridge with just nails and not use hammers. You wouldn't think about putting something in the jungle that was built the same way as a structure that you would build in Arizona.
So, thinking about use-cases and contexts with human data, and creating human experiences, is everything. And it makes a lot of sense. If you think about how we're regulated primarily in the U.S., we'll leave the bankers off for a moment because they're different agencies, but the Federal Communications Commission, the Federal Trade Commission; so, we're thinking about commercial interests; we're thinking about communication. And communication is wildly imperfect why? Because it's humans doing all the communicating!
So, any time you talk about something that is as human and humane as processing information that impacts the lives and cultures and commerce of people, you're going to have to really over-rotate on context. That doesn't mean everyone gets a specialty thing, but it doesn't mean that everyone gets a car in any color that they want so long as it's black.
David Bray: And I want to amplify what Michelle is saying. When I arrived at the FCC in late 2013, we were paying for people to volunteer what their broadband speeds were in certain, select areas because we wanted to see that they were getting the broadband speed that they were promised. And that cost the government money, and it took a lot of work, and so we effectively wanted to roll up an app that could allow people to crowdsource and if they wanted to, see what their score was and share it voluntarily with the FCC. Recognizing that if I stood up and said, "Hi! I'm with the U.S. government! Would you like to have an app [...] for your broadband connection?" Maybe not that successful.
But using the principles that you said about privacy engineering and privacy design, one, we made the app open source so people could look at the code. Two, we made it so that, when we designed the code, it didn't capture your IP address, and it didn't know who you were in a five-mile-radius. So, it gave some fuzziness to your actual, specific location, but it was still good enough for informing whether or not broadband speed is as desired.
And once we did that; also, our terms and conditions were only two pages long; which, again, we dropped the gauntlet and said, "When was the last time you agreed to anything on the internet that was only two pages long?" Rolling that out, as a result, ended up being the fourth most-downloaded app behind Google Chrome because there were people that looked at the code and said, "Yea, verily, they have privacy by design."
And so, I think that this principle of privacy by design is making the recognition that one, it's not just encryption but then two, it's not just the legalese. Can you show something that gives people trust; that what you're doing with their data is explicitly what they have given consent to? That, to me, is what's needed for AI [which] is, can we do that same thing which shows you what's being done with your data, and gives you an opportunity to weigh in on whether you want it or not?
Does AI require a new level of information security?
David Bray: So, I'll give the simple answer which is "Yes." And now I'll go beyond that.
So, shifting back to first what Michelle said, I think it is great to unpack that AI is many different things. It's not a monolithic thing, and it's worth deciding are we talking about simply machine learning at speed? Are we talking about neural networks? This matters because five years ago, ten years ago, fifteen years ago, the sheer amount of data that was available to you was nowhere near what it is right now, and let alone what it will be in five years.
If we're right now at about 20 billion networked devices on the face of the planet relative to 7.3 billion human beings, estimates are at between 75 and 300 billion devices in less than five years. And so, I think we're beginning to have these heightened concerns about ethics and the security of data. To Scott's question: because it's just simply we are instrumenting ourselves, we are instrumenting our cars, our bodies, our homes, and this raises huge amounts of questions about what the machines might make of this data stream. It's also just the sheer processing capability. I mean, the ability to do petaflops and now exaflops and beyond, I mean, that was just not present ten years ago.
So, with that said, the question of security. It's security, but also we may need a new word. I heard in Scandinavia, they talk about integrity and being integral. It's really about the integrity of that data: Have you given consent to having it used for a particular purpose? So, I think AI could play a role in making sense of whether data is processed securely.
Because the whole challenge is right now, for most of the processing we have to decrypt it at some point to start to make sense of it and re-encrypt it again. But also, is it being treated with integrity and integral to the individual? Has the individual given consent?
And so, one of the things raised when I was in conversations in Taiwan is the question, "Well, couldn't we simply have an open-source AI, where we give our permission and our consent to the AI to have our data be used for certain purposes?" For example, it might say, "Okay, well I understand you have a data set served with this platform, this other platform over here, and this platform over here. Are you willing to have that data be brought together to improve your housekeeping?" And you might say "no." He says, "Okay. But would you be willing to do it if your heart rate drops below a certain level and you're in a car accident?" And you might say "yes."
And so, the only way I think we could ever possibly do context is not going down a series of checklists and trying to check all possible scenarios. It is going to have to be a machine that can talk to us and have conversations about what we do and do now want to have done with our data.
What about the risks of creating bias in AI?
Michelle Dennedy: Madeleine Clare Elish wrote a paper called "Moral Crumple Zones," and I just love even the visual of it. If you think about cars and what we know about humans driving cars, they smash into each other in certain known ways. And the way that we've gotten better and lowered fatalities of known car crashes is using physics and geometry to design a cavity in various parts of the car where there's nothing there that's going to explode or catch fire, etc. as an impact crumple zone. So all the force and the energy goes away from the passenger and into the physical crumple zone of the car.
Madeleine is working on exactly what we're talking about. We don't know when it's unconscious or unintentional bias because it's unconscious or unintentional bias. But, we can design-in ethical crumple zones, where we're having things like testing for feeding, just like we do with sandboxing or we do with dummy data before we go live in other types of IT systems. We can decide to use AI technology and add in known issues for retraining that database.
I'll give you Watson as an example. Watson isn't a thing. Watson is a brand. The way that the Watson computer beat Jeopardy contestants is by learning Wikipedia. So, by processing mass quantities of stated data, you know, given whatever levels of authenticity that pattern on.
What Watson cannot do is selectively forget. So, your brain and your neural network are better at forgetting data and ignoring data than it is for processing data. We're trying to make our computer simulate a brain, except that brains are good at forgetting. AI is not good at that, yet. So, you can put the tax code, which would fill three ballrooms if you print it out on paper. You can feed it into an AI type of dataset, and you can train it in what are the known amounts of money someone should pay in a given context?
What you can't do, and what I think would be fascinating if we did do, is if we could wrangle the data of all the cheaters. What are the most common cheats? How do we cheat? And we know the ones that get caught, but more importantly, how do [...] get caught? That's the stuff where I think you need to design in a moral and ethical crumple zone and say, "How do people actively use systems?"
The concept of the ghost in the machine: how do machines that are well-trained with data over time experience degradation? Either they're not pulling from datasets because the equipment is simply ... You know, they're not reading tape drives anymore, or it's not being fed from fresh data, or we're not deleting old data. There are a lot of different techniques here that I think have yet to be deployed at scale that I think we need to consider before we're overly relying [on AI], without human checks and balances, and processed checks and balances.
How do we solve this bias problem?
David Bray: I think it's going to have to be a staged approach. As a starting point, you almost need to have the equivalent of a human ombudsman - a series of people looking at what the machine is doing relative to the data that was fed in.
And you can do this in multiple contexts. It could just be internal to the company, and it's just making sure that what the machine is being fed is not leading it to decisions that are atrocious or erroneous.
Or, if you want to gain public trust, share some of the data, and share some of the outcomes but abstract anything that's associated with any one individual and just say, "These types of people applied for loans. These types of loans were awarded," so can make sure that the machine is not hinging on some bias that we don't know about.
Longer-term, though, you've got to write that ombudsman. We need to be able to engineer an AI to serve as an ombudsman for the AI itself.
So really, what I'd see is not just AI as just one, monolithic system, it may be one that's making the decisions, and then another that's serving as the Jiminy Cricket that says, "This doesn't make sense. These people are cheating," and it's pointing out those flaws in the system as well. So, we need the equivalent of a Jiminy Cricket for AI.
CXOTALK brings you the world's most innovative business leaders, authors, and analysts for in-depth discussion unavailable anywhere else. Enjoy all our episodes and download the podcast from iTunes and Spreaker.