Lessons learned from Google's application of artificial intelligence to user experience

Google's user experience (UX) proponents have shared how they have been able to apply a potent new tool to promote and embed human-centered design into the site's projects: machine learning. In a recent post, Josh Lovejoy, UX Designer for Google, describes the process he and his team employed to integrate what they call "human centered machine learning" into a recent initiative.
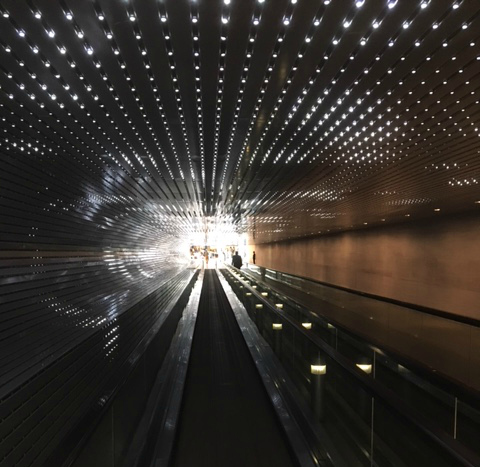
"Our team at Google works across the company to bring UXers up to speed on core [machine learning] concepts, understand how to best integrate machine learning into the UX utility belt, and ensure we're building machine learning and AI in inclusive ways," Lovejoy explains. A great deal of human-centered machine learning went into the development of Google Clips, an intelligent camera that learns and selects photos are meaningful to users. The goal was to help camera users avoid taking countless shots of the same subjects in the hopes of finding one or two standouts.
Machine learning systems were trained to seek out the best photos -- but it required a great deal of training to get the model right, Lovejoy relates. Plus, quite a bit of rethinking was required to reduce the complexity of the user interfaces.
In a previous post, Lovejoy and a colleague, Jess Holbrook, outlined the seven core principles behind human-centered machine learning that were applied to the Google Clips project:
- "Don't expect machine learning to figure out what problems to solve"
- "Ask yourself if machine learning will address the problem in a unique way"
- "Fake it with personal examples and wizards" (Ask participants at user research sessions to test with their own data.)
- "Weigh the costs of false positives and false negatives" (Determine what errors are most impactful to users)
- "Plan for co-learning and adaptation"
- "Teach your algorithm using the right labels" (The system needs to be trained to be able to answer the question "Is there a cat in this photo?")
- "Extend your UX family, machine learning is a creative process" (Machine learning isn't just for engineers, everyone needs to get involved.)
In his latest update, Lovejoy expresses some universal truths the Google teams have learned and now adhere to in the process of using AI to produce superior UX:
UX proponents need to understand machine learning. It's important that software designers, as well as developers, have an understanding of what AI and machine learning will bring to the table. "It'll be essential that they understand certain core ML concepts, unpack preconceptions about AI and its capabilities, and align around best-practices for building and maintaining trust," Lovejoy says.
User requirements are everything. No matter how sophisticated the technology, it alone can't identify and solve business problems or act on business opportunities. Lovejoy relates. "If you aren't aligned with a human need, you're just going to build a very powerful system to address a very small--or perhaps nonexistent--problem," Lovejoy relates.
It's about trust. Many employees -- and executives for that matter -- have a fear of AI. Simply engineering AI into processes and products without their input will only exacerbate those fears.
It's about the enterprise and its corporate culture. As with all important technology developments, an adverse or siloed corporate culture will only lead to resistance and dysfunction. "Every facet of ML is fueled and mediated by human judgement; from the idea to develop a model in the first place, to the sources of data chosen to train from, to the sample data itself and the methods and labels used to describe it, all the way to the success criteria for wrongness and rightness," says Lovejoy.