MIT explores potential of wearable AI to serve as a "social coach"

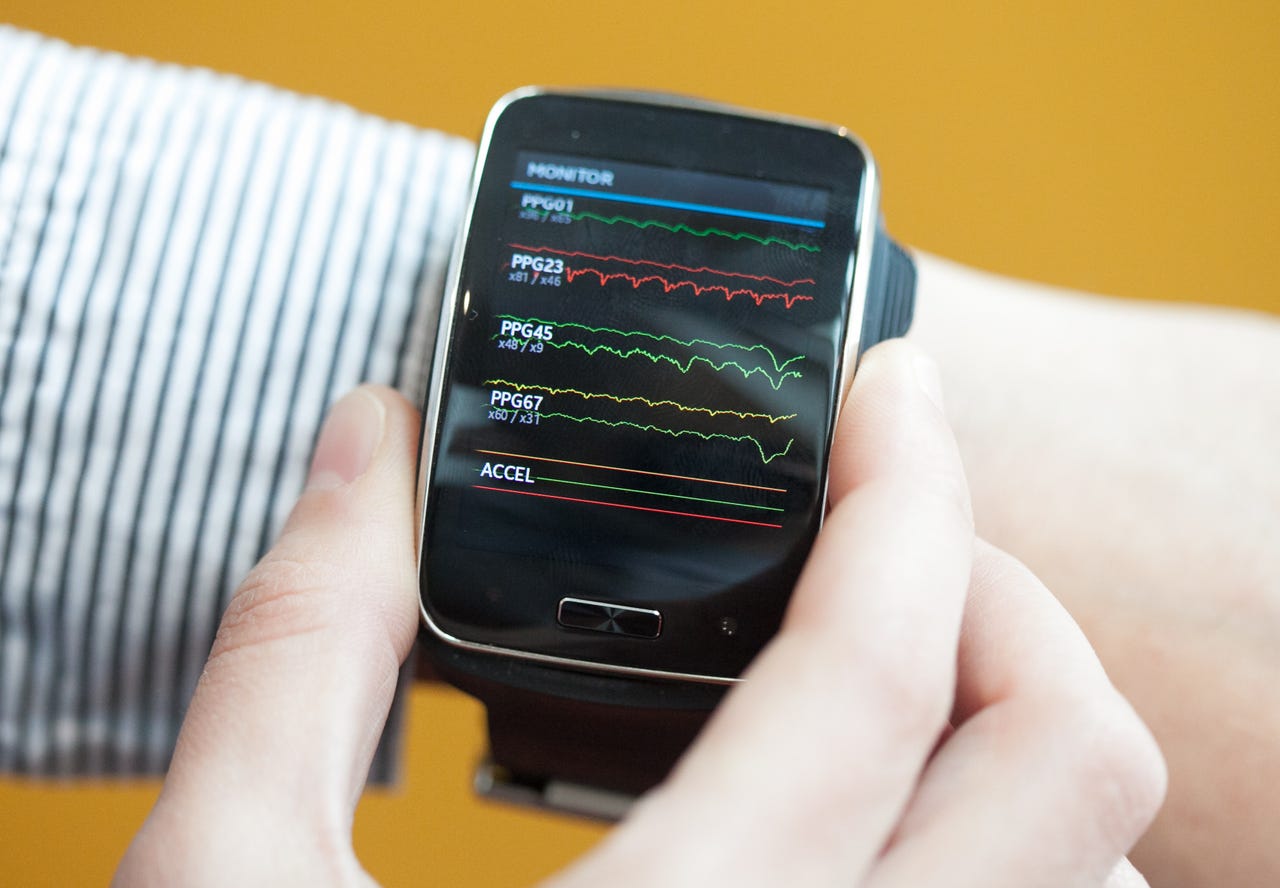
For people with conditions like autism or social anxiety, interpreting everyday social cues like body language or tone of voice can be daunting.
Researchers from MIT's Computer Science and Artificial Intelligence Laboratory (CSAIL) are hoping that one day, their research could give people an AI-powered wearable device to help them pick up on those cues.
Those researchers are presenting the results of their latest study, which used deep learning to determine in real time whether a conversation is happy, sad or neutral based on a person's speech patterns and vital signs. The data was collected with a Samsung Simband, in the hopes that the algorithms developed could one day be used in a wearable device that offers "social coaching" for people with disabilities.
"Imagine if, at the end of a conversation, you could rewind it and see the moments when the people around you felt the most anxious," graduate student Tuka Alhanai, who co-authored a related paper with PhD candidate Mohammad Ghassemi, said in a statement. "Our work is a step in this direction, suggesting that we may not be that far away from a world where people can have an AI social coach right in their pocket."
Alhanai and Ghassemi are presenting their paper at the Association for the Advancement of Artificial Intelligence (AAAI) conference in San Francisco.
The researchers developed their AI system by capturing 31 conversations, each several minutes long. They trained two algorithms on the data -- one to determine whether the overall conversation was happy or sad, and one to classify five-second blocks of each conversation as either positive, negative or neutral.
The subjects wore a Samsung Simband to measure features like movement, heart rate, blood pressure, blood flow and skin temperature,. The system also captured audio data and text transcripts to analyze the speaker's tone, pitch, energy, and vocabulary.
The system associated long pauses and monotonous vocal tones with sadder stories, while more energetic, varied speech patterns were associated with happier ones. Physical cues like fidgeting or increased cardiovascular activity were associated with sad stories. On average, the system could classify the moods of five-second snippets of conversation with an accuracy rate that was 18 percent above chance.
The research fits into fast-moving trends: Sentiment analysis is being applied to a growing range of devices and may soon be available to the maker community via the Raspberry Pi board. And while the wearables market has yet to take off as some have hoped for, there's still plenty of optimism about its growth potential.
The MIT study seems to marry both trends in an impressive way: Ghassemi noted that the experiment is unique in that it allowed its subjects to carry on natural interactions, even as robust physical and speech data was collected. Similar studies have relied on showing participants videos to track emotions, or asking them to act out specific emotions.
Additionally, keeping privacy concerns in mind, the researchers developed their system to run locally on a user's device.
The research team is now aiming to expand their study, potentially with the use of commercial devices like the Apple Watch.
"Our next step is to improve the algorithm's emotional granularity so it can call out boring, tense, and excited moments with greater accuracy instead of just labeling interactions as 'positive' or 'negative'," Alhani said. "Developing technology that can take the pulse of human emotions has the potential to dramatically improve how we communicate with each other."