Reality Engines offers a deep learning tour de force to challenge Amazon et al in Enterprise AI
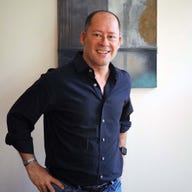

Bindu Reddy, co-founder and chief executive of startup Reality Engines, unveiled a slew of enterprise apps based on cutting-edge deep learning techniques. "Our moat comes both from constantly innovating and in getting more and more practice on key enterprise use-cases," said Reddy, who was formerly head of "AI verticals" at Amazon's AWS cloud service.
Primers
Barely a year old, Reality Engines of San Francisco emerged from stealth mode on Tuesday, announcing a slew of artificial intelligence offerings to perform corporate tasks such as budgeting for cloud services or monitoring corporate networks for break-ins.
Most exciting of all is that the tiny 18-person team has some very novel takes on deep learning forms of AI, the product of seasoned vets in machine learning technology and products. This is no me-too chatbot service, it would appear.
Founders include Bindu Reddy, who was previously head of "AI Verticals" for Amazon's AWS. The company is funded with a single very parsimonious round of $5.25 million from former Alphabet chairman Eric Schmidt, along with Khosla Ventures and others.
The goal, Reddy told ZDNet, is a service that "automatically creates production-ready models from data in the wild," to ease the labor of corporations that don't have massive teams of data scientists and deep learning programmers.
"While other companies talk about offering this service, it is still largely a pipe-dream," wrote Reddy in an email exchange with ZDNet. "We have made significant strides towards this goal," she said.
In fact, the outlines of the Reality Engines service are rather like if one took enterprise AI as currently offered and turned up the volume on the deep learning aspects of the underlying technology.
Offerings for enterprise that use AI from Amazon, Google, Microsoft, IBM, Oracle, and Salesforce have tended to fall into some typical use cases, such as chatbots, sales analysis, text-to-speech, and the like. They make use of a mish-mash of technology such as "AutoML," of varying sophistication and quality.
The Reality Engines offering goes up a level above simple program functions like those to more substantial corporate applications. They include such apps as how to optimize a company's spend on cloud computing services fees; financial metrics forecasting such as revenue and expenses; predictive lead scoring for sales teams; and "affinity scores" for ranking product preferences among customers.
The most interesting part of the story is that all of it is based on a combination of the most recent developments in deep learning.
In particular, the two pillars of the offering are "generative adversarial networks," known as "GANs," and "network architecture search." Those two technologies can dramatically reduce the effort needed to build machine learning for enterprise functions, the company contends.
GANs, of course, are famous for making fake faces by optimizing a competition between two neural networks based on the encoding and decoding of real images. In this case, Reality Engines has built something called a "DAGAN," a GAN that can be used for data augmentation, the practice of making synthetic data sets when not enough data is available to train a neural network in a given domain.
DAGANs were pioneered by Antreas Antoniou of the Institute for Adaptive and Neural Computation at the University of Edinburgh in 2018. The Reality Engines team has gone one better: They built a DAGAN by using network architecture search, or "NAS," in which the computer finds the best architecture for the GAN by trying various combinations of "cells," basic primitives composed of neural network modules.
Here, too, the team innovated. As described in a paper last fall, authored by Reality Engines team members Colin White and Yash Savani, along with Willie Neiswanger, who has an appointment at Carnegie Mellon and with the enterprise AI firm Petuum, the novel approach is called "BANANAS."
BANANAS starts from the traditional approach to NAS, called "Bayesian Optimization," as first outlined by Jasper Snoek and colleagues at the University of Toronto in 2012. The Bayesian approach tries many different network architectures by computing the most probable answers combinations. In the classic approach, the set of the most probable solutions is revealed by the "Gaussian Process," a standard bell curve of a probability distribution.
A schematic to the Reality Engines approach to creating a "DAGAN," a generative adversarial network that is used to augment training data for domains where existing data is scarce. The generator-discriminator pair of the GAN feed new, synthetic training data to a "DenseNet" classifier. As the classifier gets better and better, it feeds back an optimization signal that improves the DAGAN. The DAGAN itself is created by a "neural architecture search" method invented by the company called "BANANAS."
In the Reality Engines approach, White and Savani and Neiswanger replace the Gaussian Process with a "meta" neural network. As they describe it, "we train a meta neural network to predict the accuracy of unseen neural architectures in the search space." They find that the neural-net approach to Bayesian optimization "scales far better than a Gaussian Process model as it avoids the computationally intensive matrix inversion operation."
The company combined the BANANAS procedure for NAS with the DAGAN, to create what they argue is the first DAGAN ever built by NAS. To do so, they had to do some nifty engineering to create an end-to-end procedure to find the best DAGAN. Every candidate GAN they built was then used to create synthetic data in a novel domain, based on the real data in a source domain, an example of meta-learning and transfer learning. The synthetic data set was then used to train a classifier. And the results of the classifier were used to improve the BANANAS process for building the final DAGAN.
In a preliminary paper authored by the company that was shared with ZDNet in advance of publication, the authors describe how their resulting classifiers -- made up of a "DenseNet," a form of convolutional neural network -- achieves improved accuracy in the new, target domain after being trained on the synthetic data. "The NAS-improved DAGAN improves classification accuracy on the target dataset by as much as 20.5% and can transfer between tasks," they write.
It's a tour-de-force of combined deep learning approaches: a novel NAS approach used to create a novel kind of GAN for data augmentation, which in turn can improve essential tasks such as classification.
According to co-founder Reddy, that pipeline of code is good for much more than classifiers. Deep learning approaches created from NAS can be much more accurate than other machine learning approaches on tasks such as time-series analysis. "The deep learning solution, at least in terms of the AI, will be as good, if not better than any other model," said Reddy. "For example, when it comes to incident detection, we use Variational AutoEncoders, which are generally more robust in detecting anomalies than other approaches."
She points out that "deep learning outperforms or is as-good as classical machine learning" as long as there is sufficient data, something that the DAGAN can help ensure. And deep learning "doesn't require much hand-tuning and featurization," she added, which means that "companies don't have to expend expensive talent that other models will require."
As a result, "the customer saves time and money but also get a quality product," said Reddy. The NAS approach will "create a first pass of the model," she explained, after which a customer's engineers and data scientists can "make any changes they think are appropriate" from there. The service is a pay-per-use model of SaaS, Reddy said, with a cost component for initial training of the network and then pricing for increasing amounts of inference performed in the cloud. That is in line with most a la carte pricing in enterprise cloud AI.
The initial offering of services is so varied that ZDNet asked Reddy if the company risks diluting its appeal by being spread too thin. That's not a problem, insisted Reddy, as there is a natural correspondence between the various enterprise AI tasks.
"The common thread amongst all the use cases is that they all use tabular and columnar data," she said. "Most of the early companies or customers we spoke to had tabular data use cases, which is why we started with them first." Reality Engines has 17 customers already using the service.
On the company's Web site is a demo of the GAN capabilities, with celebrity animations being made to say stuff they never said, a kind of deep fakes-as-marketing approach.
Cheesy demos aside, the company has what appears to be a very solid deep learning offering underlying a straightforward promise of automation: Put your data in the company's cloud and turn the switch, and both network architecture and training data can be set in motion with relative ease.
Additional research papers by the company hint at important future possibilities for the service. For example, White teamed up with colleague Naveen Sundar Govindarajulu last year to outline how first-order logic predicates could be incorporated into deep learning by converting the logic "constraints' into items that can be differentiable, hence, resolved via backpropagation.
Incorporating logic-based constraints into traditional supervised deep learning is a "compelling technique," said Reddy, especially in cases with low amounts of training data. Indeed, one imagines that differentiable "priors" for a neural net may make up for some lack of data.
In general, Reality Engines intends "not just to keep up with research advances but also be at the forefront of these advances by actually doing that research," said Reddy. Certainly, the executive team seems well-equipped for that challenge given the depth of experience. Reality Engines's chief technology officer, Arvind Sundararajan, was a senior engineering leader for Uber's autonomous vehicle program and was also previously the engineering lead for the backend infrastructure used by Google Gmail. The company's research director, Siddartha Naidu, started BigQuery, the cloud database initiative at Google.
Taking on multiple Goliaths, namely, AWS and Google Cloud and Azure and IBM Watson, will certainly require much more capital, and Reddy told ZDNet the company expects to raise more rounds of funding "over the course of the year," adding that "we have had a considerable amount of venture capital interest to-date."
In the meantime, it's the beginning of an intriguing race to stay ahead of the technology but also the shifting nature of the market and customer needs. Technology alone is not enough, as good as it may be, something of which Reddy is well aware.
"Our moat comes both from constantly innovating," she said, "and in getting more and more practice on key enterprise use-cases."