Analytics helps hospitals avoid billing losses
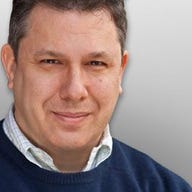
Hospital software systems can be very siloed. While various systems are are to some degree standards, they tend to be so only within specific medical specialties. Integrating systems between specialties gets tricky, making it tough to get a "360 degree" view of a hospital's clinical environment.
And It's not just that clinical systems have trouble talking to each other, but they also have trouble talking to administrative billing systems. Because of this, the hospital bills that go out to insurance companies and, on occasion, to individuals, can be missing important charges. Given how many hospitals run at or below break-even, these lost billings can sometimes make the difference between the hospital having operational viability and not.
What if technology could actually be used to fix these problems with...technology?
Fixing the problem Systems do exist to correct these data errors, but they tend to be rule-based, involving just a straight evaluation of conditions for error, and then flagging of records for which the conditions are true. This allows nurse auditors to look though the set of data records that have been flagged, so as to determine whether they truly contain billing errors. Why the second evaluation by humans? Because rule-based systems can miss nuance and judgements that cause them to identify certain bills erroneously, or to make a very big deal out of what might be a trivial matter, monetarily.
Rule-based systems don't triage and they don't prioritize, nor do they take into account contextual problems and circumstances. Rules-based systems don't "learn," but rather do as they're told, sometimes rather blindly.
This is a job for machine learning In response, Opera Solutions is today announcing its Revenue Cycle Management solution for hospitals. Opera Solutions is an analytics specialist, so it comes as no surprise that this product uses pattern-based algorithms to analyze billing and clinical records intelligently. Bigger discrepancies get prioritized, as do those with a high probability of under-billing, where that probability is determined by patterns gleaned from the prior analysis of records.
Opera's technology understands the clinical context too, so it can avoid "false positives." For example, the technology can filter out records that look generically wrong but in reality make sense, based on how doctors work and how insurance companies pay.
The product is already live in about 60 hospitals, providing a substantial knowledge base that allows Opera to improve the algorithms, and the algorithms to improve themselves. Such a learning, and self-improving solution works nicely and wards off rote application of rules-based bill auditing. Other industries have benefited from such solutions before, but in health care, I am told, this is relatively new.
Data should be smart, not just Big Big Data is great, but some of its major value lies in the ability to analyze it and garner predictive patterns, for follow-on analyses. Opera's business model is all about that, and this new health care solution which prevents "financial leakage" shows why they are doing so well.