Can AI predict global pandemics like the coronavirus?
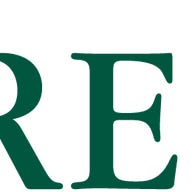
AI is supposed to be the most powerful pattern detection and prediction technology in the world. It, therefore, begs the question: Can we use AI to predict future global pandemicsfar enough in advance to tamp them down or prevent them altogether? It's an enormously consequential question because the answer is not only relevant to sounding the alarm on future pandemics; it also speaks to the potential of AI for businesses.
The short answer is "yes-ish." "Yes" because AI, specifically machine learning (ML), analyzes historical data to find the key variables that are predictive of any event, such as a pandemic. "Ish" because ML's success is dependent on the availability and richness of the data fed to it by data scientists. This is simultaneously the illimitable potential and fundamental truth of AI. As I frequently explain, "Algorithms get all the press, but it is the data that leads to success."
Sparse events are the hardest to predict
This is not the first time I have been asked how AI can be used to predict life-threatening events. I was once asked, "How can we use ML to predict terror events?" My glib answer: "If you want to use ML to predict terror events, then you are going to need a whole lot more terror events." Horrible as even one terrorism event is, machine learning only works if it has enough examples in historical data sets to uncover the key variables that would predict future events. This applies to other sparse events like market crashes, earthquakes, the emergence of a game-changing startup, or any infrequent cultural, political, or natural sea-change events. Global pandemics are thankfully sparse events and therefore would likely also lack the data to predict outright the future of the next one. But that is no reason to give up on ML as a prediction tool.
Don't have the data? Don't give up
Maybe we don't have the direct data to predict the big events like the coronavirus outbreak, but we are very likely to have the data to predict one or more proxy events for an outbreak. By "proxy events," I mean other events that in themselves are predictive of the bigger event. For example, data scientists could attempt to create ML models to predict an increase in sick days, doctors' appointments, "chatter" on social media, or myriad other models that collectively might be predictive of a larger event. This approach should sound familiar: It's exactly what economists, traders, retailers, and many others use to predict GDP, the market direction, and what fashions customers will buy. Some will use ML, but others (perhaps most) will use traditional forecasting, mathematical, or rules-based models to make these predictions. The best results will come from a mix of these approaches.
When black swan events happen, data scientists use AI to help
The best case is to use AI to predict events when there is still time to do something about it. But black swans happen, and they often create an enormous amount of data. You can see that in this current pandemic. Data scientists are using AI today to create predictive models to evaluate potential cures, identify vulnerable populations, and solve many more problems.
AI needs our help to help us
Data is the fuel and machine learning is the engine of AI. A huge prerequisite to successful AI is data. Data, data, data. The world's collection of it is unprecedented! And governments, organizations, and enterprises must continue to invest. Likewise, automation in machine learning (AutoML) solutions make data scientists more productive. We need more of both: data and AutoML. With more data and AutoML, AI will help humanity in no less consequential ways as the invention of the vaccine, electricity, and the internet.
This post was written by VP, Principal Analyst Mike Gualtieri, and it originally appeared here.