Neuromorphic computing finds new life in machine learning
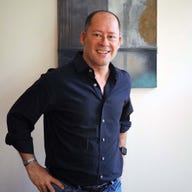
Efforts have been underway for forty years to build computers that might emulate some of the structure of the brain in the way they solve problems. To date, they have shown few practical successes. But hope for so-called neuromorphic computing springs eternal, and lately, the endeavor has gained some surprising champions.
The research lab of Terry Sejnowski at The Salk Institute in La Jolla this year proposed a new way to train "spiking" neurons using standard forms of machine learning, called "recurrent neural networks," or "RNNs."
And Hava Siegelmann, who has been doing pioneering work on alternative computer designs for decades, proposed along with colleagues a system of spiking neurons that would perform what's called "unsupervised" learning.
Neuromorphic computing is an umbrella term given to a variety of efforts to build computation that resembles some aspect of the way the brain is formed. The term goes back to work by legendary computing pioneer Carver Mead in the early 1980s, who was interested in how the increasingly dense collections of transistors on a chip could best communicate. Mead's insight was that the wires between transistors would have to achieve some of the efficiency of the brain's neural wiring.
There have been many projects since then, including work by Winfried Wilcke of IBM's Almaden Research Center in San Jose, as well as The TrueNorth chip effort at IBM; and the Loihi project at Intel, among others. ZDNet's Scott Fulton III had a great roundup earlier this year of some of the most interesting developments in neuromorphic computing.
Also: AI is changing the entire nature of compute
So far, such projects have yielded little practical success, leading to tremendous skepticism. During the International Solid State Circuits Conference in San Francisco, Facebook's head of A.I. research, Yann LeCun, gave a talk on trends in chips for deep learning. He was somewhat dismissive of work on spiking neural nets, prompting a backlash later in the conference by Intel executive Mike Davies, who runs the Loihi project. Davies's riposte to LeCun then prompted LeCun to make another broadside against spiking neurons on his Facebook page.
"AFAIK, there has not been a clear demonstration that networks of spiking neurons (implemented in software or hardware) can learn a complex task," said LeCun. "In fact, I'm not sure any spiking neural net has come even close to state-of-the-art performance from garden-variety neural nets."
But Sejnowski's lab and Siegelmann's team at the Defense Advanced Research Projects Agency's Biologically Inspired Neural and Dynamical Systems Laboratory provide new hope.
Sejnowski, during a conversation with ZDNet at the Salk Institute in April, predicted a major role for spiking neurons in future.
"There's going to be another big shift, which will probably occur within the next five to ten years," said Sejnowski.
"The brain is incredibly efficient, and one of the things that makes it efficient is because it uses spikes," observed Sejnowski. "If anyone can get a model of a spiking neuron to implement these deep nets, the amount of power you need would plummet by a factor of a thousand or more. And then it would get sufficiently cheap that it would be ubiquitous, it would be like sensors in phones."
Hence, Sejnowski thinks spiking neurons can be a big boost to inference, the task of making predictions, on energy-constrained edge computing devices such as mobile phones.

Machine learning pioneer Terry Sejnowski and his team at Salk Institute in La Jolla, California, have developed a way to transfer the parameters from a normal neural network to a network of spiking neurons, to get around the traditional lack of a "learning rule" by which to train such spiking neurons. Sejnowski predicts a big role for such neuromorphic computing in years to come.
The work by Sejnowski's lab, written by Robert Kim, Yinghao Li, and Sejnowski, was published in March. Titled, "Simple Framework for Constructing Functional Spiking Recurrent Neural Networks," the research, posted on the Bioarxiv pre-print server, describes training a standard recurrent neural network, or "RNN," and then transfers those parameters to a spiking neural network. The idea is to get around the fact that spiking neurons currently have no way that they can be trained via gradient descent, the lynchpin of conventional machine learning.
Spiking neurons don't fit with the standard learning rule of deep learning, in other words. The new research is a form of what's called "transfer learning," developing parameters in one place and carrying them over to a new place, to get around that shortcoming of spiking neurons.
Also: What neuromorphic engineering is, and why it's triggered an analog revolution
As the authors explain, "The non-differentiable nature of spike signals prevents the use of gradient descent-based methods to train spiking networks directly."
"Our method involves training a continuous-variable rate RNN (recurrent neural network) using a gradient descent-based method, and transferring the learned dynamics of the rate network along with the constraints to a spiking network model in a one-to-one manner."
Hava Siegelmann and colleagues at DARPA's Biologically Inspired Neural and Dynamical Systems Laboratory claim progress in training spiking neurons using a modified "voting" mechanism that decides between outputs of individual neurons.
"This is taking an already trained network," explained Sejnowski. "The next step is going to be to do the learning on the spiking. We think we can solve that one too, but it's still early days."
As to who will create these circuits for real, that remains to be seen, though Sejnowski vaguely referred to the possibility that a company such as Qualcomm, the dominant vendor of mobile baseband chips, could be a candidate.
The work by Siegelmann's group at DARPA is of a similar nature. Titled, "Locally Connected Spiking Neural Networks for Unsupervised Feature Learning," posted in April on arXiv, the paper is authored by Daniel J. Saunders, Devdhar Patel, and Hananel Hazan, along with Siegelmann, and by Robert Kozma, who holds a dual affiliation with the Center for Large-Scale Intelligent Optimization and Networks at the University of Memphis math department in Memphis, Tennessee.
- What is AI? Everything you need to know
- What is deep learning? Everything you need to know
- What is machine learning? Everything you need to know
- What is cloud computing? Everything you need to know
As with Sejnowski's group, Siegelmann's group observe that the problem is the lack of a proper training procedure or learning rule. "few methods exist for the robust training of SNNs from scratch for general-purpose machine learning ability," they write, "their abilities are highly domain- or dataset-specific, and require much data pre-processing and tweaking of hyper-parameters in order to attain good performance."
To meet the challenge, Siegelmann's team developed last year a Python-based programming package called "BindsNET," which they have used in previous studies to do a kind of transfer learning that sounds similar to what Sejnowski's group did. (BindsNET is posted on Github.)
Using BindsNET, in the current work, Siegelmann's group simulated the construction of shallow artificial neural networks made up of spiking neurons. The shallow network is analogous to a convolutional neural network in conventional machine learning, they write. To resolve the problem of a learning rule, they employ something called "spike-timing-dependent plasticity," or STDP, which acts as a kind of voting mechanism that counts the times that individual neurons fire in response to data, and also the sequence in which they fire. Neurons that are input with patches of image data vote on their candidate for the class of the image, and the pooling of their votes forms an image classifier.
The famous MNIST database of hand-written digits is used as the test, where the neurons are tasked with classifying what digit an image represents.
Siegelmann & Co. report that their neural network architecture proved much more efficient than other approaches with spiking neurons, meaning, it needed fewer passes through the training data to achieve the same or better performance on test data. Within the context of spiking neurons, the big achievement of the paper is to create a more efficient arrangement of neurons that "divide and conquer the input space by learning a distributed representation." That implies spiking neurons can be more efficient going forward by requiring fewer training examples to converge during training.
Both the Sejnowski group and Siegelmann group show their is energy and intelligence active within the spiking neuron corner of neuromorphic computing. The field remains one to watch, even if it hasn't yet swayed the skeptics.