Intel’s neuro guru slams deep learning: ‘it’s not actually learning’
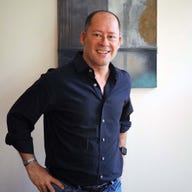
"Backpropogation doesn't correlate to the brain," insists Mike Davies, head of Intel's neuromorphic computing unit, dismissing one of the key tools of the species of A.I. In vogue today, deep learning. "For that reason, "it's really an optimizations procedure, it's not actually learning."
Davies made the comment during a talk on Thursday at the International Solid State Circuits Conference in San Francisco, a prestigious annual gathering of semiconductor designers.
Davies was returning fire after Facebook's Yann LeCun, a leading apostle of deep learning, earlier in the week dismissed Davies's own technology during LeCun's opening keynote for the conference.
"The brain is the one example we have of truly intelligent computation," observed Davies. In contrast, so-called back-prop, invented in the 1980s, is a mathematical technique used to optimize the response of artificial neurons in a deep learning computer program.
Although deep learning has proven "very effective," Davies told a ballroom of attendees, "there is no natural example of back-prop," he said, so it doesn't correspond to what one would consider real learning.
Also: Facebook's Yann LeCun reflects on the enduring appeal of convolutions
Davies then went on to give a talk about "Loihi," his team's computer model of a neural network that uses so-called spiking neurons that activate only when they receive an input sample. The contention of neuromorphic computing advocates is that the approach more closely emulates the actual characteristics of the brain's functioning, such as the great economy with which the brain transmits signals.
He chided LeCun for failing to value the strengths of that approach.
"It's so ironic," said Davies. "Yann bashed spikes but then he said we need to deal with sparsity in hardware, and that is what spikes are about."
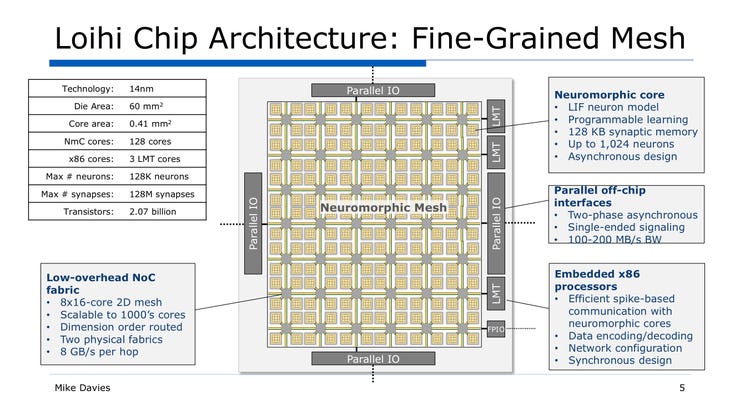
Architectural overview of Intel's "Loihi" neuromorphic computing chip.
The point-counterpoint between the two researchers is not merely academic. Intel is in the business of chips. LeCun told ZDNet in an interview Monday that Facebook has its own "internal activities" ongoing in the development of chips for A.I. Hence, some of Intel's chip business with large customers like Facebook might be in jeopardy if the company faces competition from those very customers because they have a different vision.
The Facebook AI researcher during his talk Monday morning had critiqued spiking neuron work broadly, saying that the technology had not yielded algorithms that could produce practical results. "Yann said, Why build chips for algorithms that don't work; I think these algorithms do work," said Davies.
Still, he conceded that there was at least an element of truth to LeCun's criticism. While the hardware of spiking neurons "is well adequate and provides a lot of tools to map interesting algorithms," said Davies, his department has a "goal to make progress on the algorithmic front, which is what is holding back the field."
But Davies rebuked what he called "this knee-jerk reaction that spikes are inefficient."
Also: Facebook's Yann LeCun says 'internal activity' proceeds on AI chips
Instead, he proposed, "let's look at the data."
Intel says Loihi bests conventional neural network performance on conventional processors, especially in speed at which results are computed.
Davies cited a report put out in December by an organization called Applied Brain Research of Waterloo, Ontario, which compared performance of a speech detection algorithm running on various chips. The software was trained to recognize the keyword "aloha" while also rejecting nonsense phrases. The firm ran the model on a CPU and a GPU, and also made a version that ran on the Loihi chip.
Must read
- 'AI is very, very stupid,' says Google's AI leader (CNET)
- How to get all of Google Assistant's new voices right now (CNET)
- Unified Google AI division a clear signal of AI's future (TechRepublic)
- Top 5: Things to know about AI (TechRepublic)
The Loihi chip seems to come out with much better energy efficiency, as measured by the number of joules required to perform inference on the task. (It's worth noting that the Loihi chip, however, actually delivers lower accuracy, as described in the full version of the Applied Science paper, which is available on the arXiv pre-print server.)
In another example, Davies noted that a basic classifier task performed by Loihi was forty times as fast as a conventional GPU-based deep learning network, with 8% greater accuracy.
Davies told the audience that Loihi gets "much more efficient" as it is tasked with larger and larger networks. He observed that control of robots would be the killer app for neuromorphic chips such as Loihi. "Brains evolved to control limbs" he pointed out, making robotic control a natural.
Wrapping up his talk, Davies said "there's a lot of work left to be done before we can put it out in a commercial form that's applicable to a broad range of problems," but he ended on an optimistic note, saying "We can get algorithms that work that will address Yann's skepticism."
Previous and related coverage:
What is AI? Everything you need to know
An executive guide to artificial intelligence, from machine learning and general AI to neural networks.
What is deep learning? Everything you need to know
The lowdown on deep learning: from how it relates to the wider field of machine learning through to how to get started with it.
What is machine learning? Everything you need to know
This guide explains what machine learning is, how it is related to artificial intelligence, how it works and why it matters.
What is cloud computing? Everything you need to know about
An introduction to cloud computing right from the basics up to IaaS and PaaS, hybrid, public, and private cloud.