Strata 2017 Postmortem: More virtual data lake, more operational machine learning
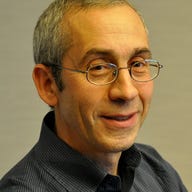
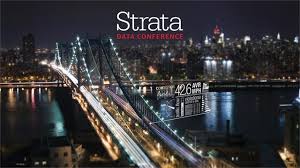
In recounting the news from Strata, the headline from my Big on Data bro Andrew Brust was an admonishment to the Hadoop community that will likely wind up as his epitaph: "Industry to elephant: Go jump in a lake." We could only top that with the addition of a single word: "Go jump in a virtual data lake."
Tech Pro Research
It was more than coincidence that Cloudera, Hortonworks, and SAP each issued announcements this week premised on the notion that data lakes are going the way of enterprise data warehouses before them: data, information, and knowledge will not be contained in a single galactic data store, but instead come from multiple sources.
The brute reality is that, while a key benefit of Big Data analytics is the ability to gain insights from all of the data, it does not always physically or economically make sense to move all of it to one place. For instance, it might be more practical to not move clickstream or log data from an operational database to a Hadoop cluster, unless it's already stored in cloud object storage.
Likewise, if you are conducting Customer 360 analytics, you probably won't move current transaction data to a data lake if you want to analyze that with historical data or log files. It's a similar realization that enterprise data warehouse architects arrived at once they realized that those so-called satellite data marts could more readily accommodate new forms of data before the EDW could, so why bother moving the data and re-structuring the EDW.
Data Virtualization is in, again... sort of
Admittedly, the idea of data virtualization is hardly new. Data integration players like Informatica have featured data virtualization for years, while Denodo has built a company around it. Data virtualization, a.k.a., the virtual data warehouse, suffered bad wraps over the years because the underlying storage and networking infrastructure wasn't fast enough to support interactive querying.
But modern approaches to pushdown query processing are factoring improved bandwidth and in-memory processing. They are processing data where it lives while providing the query optimization and governance from the source. That's the core IP behind Zoomdata and the federated big data query approaches from Oracle and Teradata.
There's yet one more powerful reason that your data lake won't become monolithic: as your organization gets more serious about cloud deployment, it's more likely not to put all of its eggs in one cloud basket. Welcome to the revenge of all those vendors who want to play Switzerland to your data, such as Hadoop providers offering services for governing multi-cluster deployments.
Data platform providers want to get into the act; they will manage and govern your data pipelines with approaches ranging from managing multi-tenanted Hadoop clusters across on premises and the cloud and delivering a data lake in a box; replicating data between clouds to providing universal data discovery services that abstract data from where it is physically stored.
Machine Learning becoming part of the core platform
When it comes to data science and machine learning, there remain serious disconnects in the job market. But either way, the hype around machine learning and AI is materializing in actual products designed to make ML and AI developers more productive.
A takeaway that we got from Strata is that increasingly, data platform providers are viewing data science and machine learning productivity tools as being natural extensions of their platforms; the goal is operationalizing machine learning at the same level that they are according to interactive SQL query, streaming analytics, and data governance.
Cloudera Data Science Workbench and IBM Data Science Experience (DSX) are now featured modules to their own Hadoop platforms. At Strata, IBM announced it is integrating DSX into its new Integrated Analytics System, the successor to its Netezza line that incorporates Db2 BLU acceleration. Earlier in the week at Ignite, Microsoft announced a key upgrade to its Azure Machine Learning service for automating one of the headaches for machine learning: data preparation.
Where there's smoke, there's fire, as any observer of NVidia's GPU business will attest. As big on data bro Andrew reported, efforts to capitalize on GPU performance are materializing through new standards for efficient memory management that will hopefully smooth some of the speed bumps that occur while marshaling data from fast storage to ridiculously scalable processor.
IoT for now? Not so much
The activity around ML overshadowed what has been one of the other hyped domains for big data: IoT. Admittedly, Big Data platform providers have provided hub and edge processing, but that only allows applications to be developed. From our conversations across the Strata expo floor, it became apparent that key hurdles have been that the majority of applications must be custom developed, and there is a lack of either the standards or open source frameworks that could help speed developers along.
While both ML/AI and IoT use cases have been highlighted, for now, enterprises have clearer ideas on how they could incorporate machine learning. In many cases, enterprises don't necessarily need to write new applications to gain the benefits of machine learning: in many cases, they could extend them with analytics that make them smarter.
For IoT, predictive maintenance is by far the clear low hanging fruit; any business with physical assets can benefit from the improved visibility that aggregating and analyzing the new wealth of sensory data could provide. But the relative lack of tools or frameworks noted above, not to mention the lack of standards around device protocols, is not making this low-hanging fruit as ripe for picking as it should be.
Previous and Related Coverage
Strata NYC 2017 to Hadoop: Go jump in a data lake
With more than 30 noteworthy announcements made at the show, this year's Strata Data New York was chock full o' news. Here's a summary, along with a set of trends we can harvest from the event.
Strata+Hadoop World postmortem: Making it real (time)
There was no shortage of AI in the agenda at Strata. Beyond the headlines, there was growing evidence that the big data community is starting to get serious about real time processing.