AI's Minority Report for retail: They know you’ll return it even before you buy it
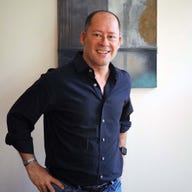
Like something out of the movie "Minority Report," where killers are apprehended before they kill based on a pre-sentiment, practitioners of machine learning are trying to gauge the likelihood you'll return a piece of apparel even before you buy it.
Myntra, the Bangalore-based online fashion retailer owned by Indian e-commerce startup Flipkart (which is backed by Walmart and others), has published new research describing experiments that assess a person's online shopping cart before they click to buy. It's based on patterns of what you've looked at online, but also a guess about your size and fit that even you may not have been aware of.
All of this is meant to enable the computer to decide, in less than 70 milliseconds, just how much of a risk of a return you are. The purpose is to decide whether to treat you differently as a return risk via reward and punishment, with a variety of measures. Those include increasing your shipping charges, as a deterrent, or offering you a coupon as an incentive in return for making the purchase non-returnable.
Myntra's researchers found in tests with real customers that the neural network's predictions, and the rewards and punishments, reduced return rates in measurable ways.
The authors combine several pieces of information into a "deep neural network" to predict product returns, including "latent" vectors of product information, historical return rates, and also a personalized sizing vector that knows that what you're buying may not fit.
The paper, "Early Bird Catches the Worm: Predicting Returns Even Before Purchase in Fashion E-commerce," is posted on the arXiv pre-print server, and is authored by Sajan Kedia, Manchit Madan, and Sumit Borar of Myntra. Borar has since gone to work at Google.
The paper is noteworthy as well for being released last week along with two other papers by Myntra researchers. In one paper, "One Embedding To Do Them All," the authors create a new kind of product listing for retail by combining several sources of information. The third paper, "Fashion Retail: Forecasting Demand for New Items," predicts which new apparel items will do well based on past trends but also based on a model of styles and brands and pricing, a model that can anticipate how brand-new items will do before they go on sale. That last paper is being presented in August at the Knowledge Discovery and Data Mining conference in Anchorage, Alaska.
But it is the "Early Bird" paper that seems to offer the most striking example of how to turn retail into a kind of a game.
Also: Forget Go, Google helps AI learn to book flights on the Web
Kedia and colleagues observed that a trend toward easy returns by online retailers has led to a surge in actual returns, which incurs high "reverse-logistics" costs for those retailers. That includes the cost to ship back, and the cost of missed sales of things while a customer has them, all of which "eats a major share of the profit margin of e-tailers," they write.
The retail industry has tried to forecast return rates, but never by "predicting in real-time, at the cart page, so that preemptive actions can be taken based on the return probability value," the authors write.
To make those real-time predictions, the authors put together a "fully-connected" deep neural network, which is trained on numerous factors about products and customers. That trained model will then produce the instantaneous assessment of the customers' cart to predict the probability of returns.
A "real-time production architecture" assesses your shopping cart in less than 70 milliseconds and decides whether to issue rewards or punishments to curb your expected returns behavior.
The factors used vary from what you'd expect to some novel inventions. Among the things you'd expect, a very obvious factor is counting how many times a given article of clothing has been returned to the store in the past by anyone.
In addition, data such as the rates at which a product listing is clicked by a given user are used to construct what's called "product embeddings" that are specific to that user. That's done by employing "matrix factorization." That process has the purpose of "transforming the user-product interaction matrix into lower dimensional latent vectors which capture the hidden attributes of the products."
How many similar things you've put in your cart is something they watch, such as the same shirt in different colors. It turns out that such doubling-up of items is a leading indicator of higher returns. In fact, the more items in total that a person has in a cart, the more their return rate has been shown to increase, they write.
"Return rates are highly dependent on the cart size," they write. "With cart size more than five products, return rates goes to 72%, whereas cart with one product has return chances of 9%." The authors don't engage in much speculation of causality, but presumably people are doing the virtual dressing-room thing, loading up on multiple versions of something, to try them at home, fully expecting to return the ones they end up not liking.
Also: Facebook enlists AI to tweak web server performance
One striking factor employed, which you might not anticipate, is what's called a "personalized sizing latent feature." The authors noticed that in historical data on returns, when people are asked why they're sending something back, over half the instances are because the item was the wrong size or didn't fit the way the person wanted.
The authors observe that it's hard, online, for a person to even know what their size is because the way sizes are listed and described can vary from item to item or from brand to brand. Therefore, they propose creating a vector that concatenates information "from the lifetime clickstream data" of the user. "Here products are defined in a detailed manner like 'Nike-Men-Shoes-Sports-10', where 10 is the size." In addition to information by individual brand, information for entire categories of apparel is aggregated, including sizing information, "which helps in understanding all size related attributes for a product."
The authors embed all that information using the popular "skip-gram" approach developed by Google's Tomas Mikolov and colleagues in 2013, using the "Word2Vec" algorithm those authors developed.
As a result, when they spy on your cart, and examine what you've got, they can compare your intended purchases to "sizing vectors which explain the user's body shape & fit for different brands & products."
Using all these embeddings, run through the neural network, the program creates a probability score of potential returns. The authors conducted a "live" test in "A/B" fashion, showing some shoppers incentives or penalties based on the analysis, while leaving a control group to experience the normal shopping experience. It was tested on 100,000 users on the production Myntra shopping site, they write.
They suggest they were able to get fairly precise analysis in real time.
Also:
- What is AI? Everything you need to know
- What is deep learning? Everything you need to know
- What is machine learning? Everything you need to know
- What is cloud computing? Everything you need to know
"The dual model first predicts the return probability for a cart and then uses this in a gradient boosted approach to identify the exact number of products that will be returned from that cart." That prediction is fed into a "real-time production architecture" that makes decisions about the rewards and punishments to implement, if any.
The approach got results, they write. When they varied the shipping charge, for example, on a person-by-person basis, orders went down by 1.7%, but returns also went down, by an even higher 3%. When a coupon was offered in return for making items non-returnable, 27% of customers took the offer and returns went down by 4%, they note.
The lesson for Kedia and colleagues is clear: this kind of statistical anticipation improves aspects of the business.
"Experiment results on action items show that accurate prediction of returns can lead to a reduction in the rate of return." They plan to pursue more "action items" in future work, they write.
Meanwhile, the lesson for consumers is clear, too: When you shop online, you're participating in a game, a game whose rules the merchant knows much better than you do. And while you know very little about how they play the game, the merchant, using machine learning, increasingly knows a heck of a lot about how you play.