AI startup Graphcore says most of the world won't train AI, just distill it
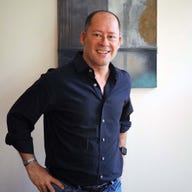
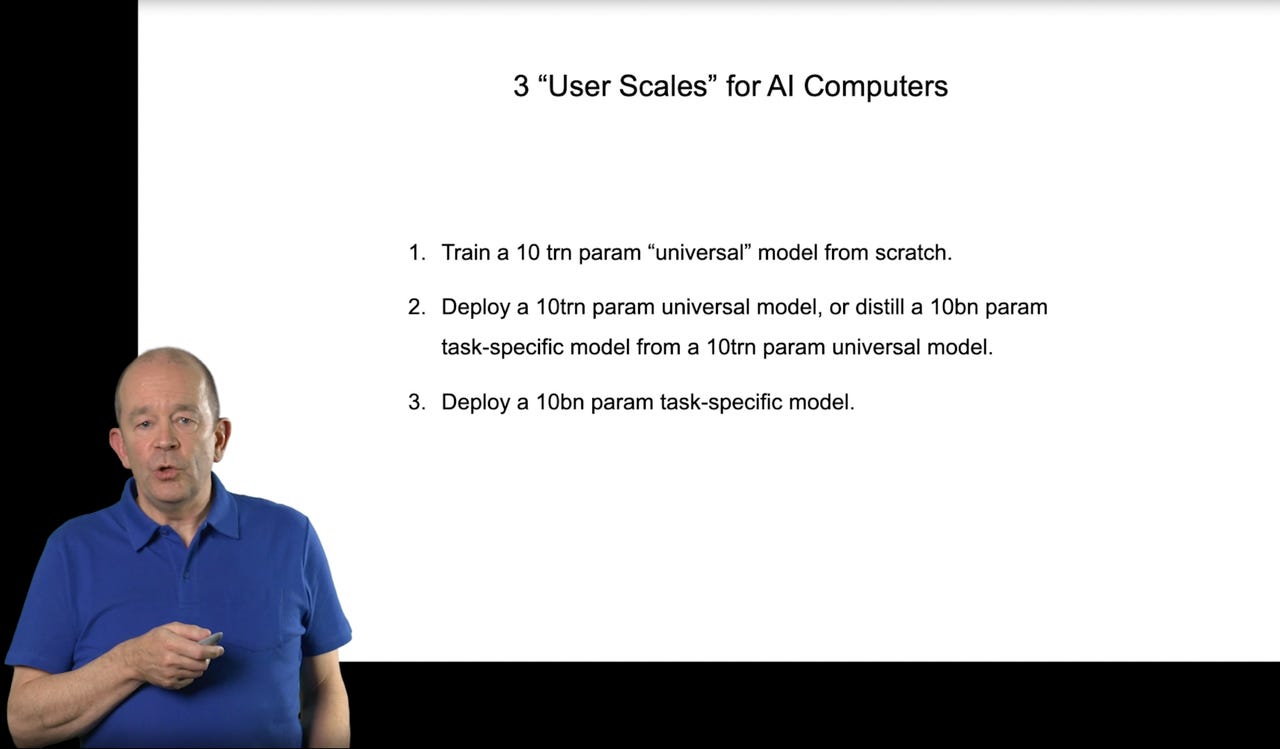
Simon Knowles, chief technology officer of the AI computing startup Graphcore, told a supercomputing conference on Wednesday most of the world won't have the dollars required to train neural network models, which are trending toward a trillion parameters, or weights, apiece. Instead, such huge models will be distilled down by users with less compute power, to make something purposeful and manageable.
Simon Knowles, chief technologist for Bristol, England-based AI computing startup Graphcore, on Wednesday told an audience of supercomputing professionals that the large mass of AI work in years to come will be done by people distilling large deep learning models down to something useable that is more task-specific.
"There are three user scales for people who might want to buy and own, or rent, an AI computer," said Knowles in the talk, a video of which is posted online. "There'll be a very small number of people who will train enormous tera-scale models from scratch," said Knowles, referring to deep learning models that have a trillion parameters, or weights.
"From those universal models, other companies will just rent those and distill from them task-specific models to do valuable things," said Knowles. A third category of users won't train models at all, they simply act as consumers of the distilled models created by the second group, he suggested.
Also: AI chip startup Graphcore enters the system business claiming economics vastly better than Nvidia's
Graphcore is known for building both custom chips to power AI, known as accelerators, and also full computer systems to house those chips, with specialized software.
In Knowles's conception of the pecking order of deep learning, the handful of entities that can afford "thousands of yotta-FLOPS" of computing power -- the number ten raised to the 24th power -- are the ones that will build and train trillion-parameter neural network models that represent "universal" models of human knowledge. He offered the example of huge models that can encompass all of human languages, rather like OpenAI's GPT-3 natural language processing neural network.
"There won't be many of those" kinds of entities, Knowles predicted.
Companies in the market for AI computing equipment are already talking about projects underway to use one trillion parameters in neural networks.
By contrast, the second order of entities, the ones that distill the trillion-parameter models, will require far less computing power to re-train the universal models to something specific to a domain. And the third entities, of course, even less power.
Knowles was speaking to the audience of SC20, a supercomputing conference which takes place in a different city each year, but this year is being held as a virtual event given the COVID-19 pandemic.
The thrust of Knowles's talk to the assembled was to make the case for Graphcore's IPU chip for deep learning, and the accompanying computer system Graphcore has built.
Knowles said many AI computing companies are pursuing the wrong memory technology, high-bandwidth memory, or HBM, consisting of stacked die that cut down on the latency incurred to send and receive data to and from main memory, which resides off of the accelerator chip.
Knowles made the case the industry's propensity to use high-bandwidth memory for AI accelerators is a mistake, given the costliness of the parts. AI needs lots of capacity, not bandwidth, said Knowles.
"In the AI accelerator space recently, everyone has been chasing bandwidth, and I think that's mis-informed," said Knowles. "I think it should be chasing capacity. We don't actually need the bandwidth." Almost all AI products in the market today have high-bandwidth memory, observed Knowles.
"The priority for DRAM should be capacity for the off-chip DRAM, because we need those human-scale models," said Knowles, "because we don't want to only operate human-scale models on computers that are the size of a warehouse."
Anyone who can buy a rack-scale machine, said Knowles, should be able to "at least manipulate a human-scale model and distill smaller models from it."
HBM's high cost relative to typical DDR server memory, said Knowles, would be prohibitive, with HBM costing five times as much per gigabyte as DDR.
"It's very, very expensive," said Knowles of HBM, "partly as a result of the assembly yield, but mostly as a result of margin stacking: in other words, someone who buys HBM has to buy it from the manufacturer of the memory, and then they sell it to you with their margin on top," versus buying DDR from the memory maker directly.
That cost difference means HBM can't achieve the kinds of scale needed to store the very large multi-billion or multi-trillion-parameter memory systems, said Knowles.
Knowles added that HBM doesn't have a path to providing more than 72 gigabytes per chip. That means "only people who could ever afford a warehouse could use it, you couldn't fit it in a rack-scale computer."