AI chip startup Graphcore enters the system business claiming economics vastly better than Nvidia’s
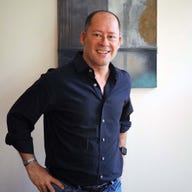
In what appears to be a trend in the world of artificial intelligence hardware and software, AI chip designer Graphcore on Wednesday morning said that its latest very large chip for AI will be sold in a four-chip server computer that sits in a rack, putting Graphcore into the expanding market for dedicated AI server computers.
Graphcore, based in Bristol, U.K., which has received over $300 million in venture capital, unveiled what it calls the Mk2 GC200, or Mark-2, as the company refers to it, its latest processor dedicated to handling machine learning operations of neural networks. It also said that it will begin selling a four-chip computer called the M2000 that is housed in a standard 1U pizza box chassis.
"This is really what we've been working on since we started the company," Nigel Toon, chief executive of Graphcore, told ZDNet in an interview via Zoom.
Building its own computer is a departure for Graphcore from its existing business model, whereby the company sells a plug-in PCIe card that is meant to live inside someone else's server computer. Previously, Graphcore has partnered with Dell to develop systems from those cards.
But the increasing need to cluster machines meant that someone had to solve the scaling of chips into very large systems, said Toon.
"The key is that you know people want to do really big models," said Toon, referring to neural network computer models in artificial intelligence. Increasingly, programs from Google and others are so compute-intensive that they can require dozens or hundreds of chips working on parallel. Toon has previously told ZDNet such models are better-suited by Graphcore's approach to processing.
Also: AI is changing the entire nature of compute
"They want to build out these large computer systems, and they want to train really, really fast, and so what we're doing here is we're building more efficient computers."
"You want to be able to scale systems out, so how do you connect these boxes together?" he asked, rhetorically. "You need a dedicated AI [networking] fabric, so we've got that in this IPU machine."
Graphcore's pivot to systems echoes moves by several chip vendors of AI silicon in the last year or so.
Nvidia, the dominant force in AI chips, in May talked up its new DGX100 server running multiple A100 processors designed for AI, emphasizing the complexity of its systems engineering as embodied in its motherboard. Startup Cerebras Systems last fall unveiled a system that is akin to a super-computer in a box the size of a dorm fridge, running the world's largest chip. Rodrigo Liang, head of AI startup SambaNova, expressed the emerging worldview when he told ZDNet earlier this year, "you have to build the entire system."
Graphcore's Pod-64 is a 16-way 1U server cabinet containing 64 individual Mk2 chips. Such cabinets can ve clustered together via the company's IPU-Fabric technology to build a datacenter of up to 64,000 chips.
At the heart of Graphcore's computer is the Mk2 GC200 processor chip, which is even bigger than the already gigantic Mk1, the company's first product. The company moved from a 16-nanometer process technology at Taiwan Semiconductor to a 7-nanometer process, resulting in a 59.4-billion transistor die measuring 823 square millimeters. That's more than double the 23.6 billion transistors in the Mk1 but only slightly bigger than the Mk1's 815 square millimeters.
The processor's individual computing cores, which crunch numbers in parallel, have increased in number to 1,472 from 1,280, and the fast on-chip SRAM memory has been tripled to 900 megabytes. The chip can perform the equivalent of running 9,000 separate programs in parallel, noted Toon.
All that yields some immediate large speed-ups, such as a 9.3-times increase in speed in training the BERT-Large natural language neural network from Google.
Direct comparisons in performance with Nvidia are not yet available, the most immediate reason being Graphcore has yet to get its hands on an Nvidia DGX A100 to run against, though Toon said it intends to benchmark directly in coming months.
But Toon made the case that raw power and connectivity makes the M2000 a vastly more economical machine purchase. The base 4-way server costs $32,450. A purchase of eight of the machines, at $259,600, yields specs that at least on paper are far above what one would get by buying 8 DGX machines at a comparable price of $199,000: two petaflops of compute capable of handling 32-bit floating point precision math versus only 156 teraflops for the DGX; 3.6 terabytes of memory versus 320 gigs in the DGX.
"To get the same kind of throughput you'd need to spend three million dollars on Nvidia kit versus less than $300,000 on the IPU machines," said Toon.
The idea gets carried farther by assembling multiple machines into a cluster, said Toon. Sixteen of the four-chip servers can be connected in a cabinet the company calls a Pod-64, for a total of 64 chips. And 1,024 of those pods can be networked together for a total of 64,000 processors working in parallel. That allows for a total of 16 exaflops of compute, and 3.2 petabits per second of bandwidth.
With clustered systems, workloads can be dynamically assigned by Graphcore's software to any of those 64,000 processors so that the mix of processors working on different jobs can change during the day.
"Maybe you're doing inference during the day, and then you're retraining your models at night," offered Toon. "Or maybe you've got a group that are a research team and then they switch to some different models that need different configurations of IPUs connected together, so it creates a completely seamless configuration."
To enable clustering, the company invented its own clustering connectivity technology, called "IPU-Fabric." The technology, either as a direct connection between M2000s or via ethernet, supports bandwidth of up to 2.8 terabits per second in what the company states is low latency. The company says IPU-Fabric is optimized for operations such as all-reduce and other data movement needed to support AI workloads across multiple machines.
Toon took the opportunity to poke fun at Nvidia, which in April closed on the $7 billion acquisition of Mellanox Technologies in order to get high-speed interconnections for its DGX systems.
"We've had this team of 100 people working in Oslo for over three years now, building this thing from scratch for AI, and Nvidia has gone off and spent seven billion dollars to buy Mellanox, just to keep up with us, maybe."
Graphcore's chip that powers its computers, the Mk2 GC200, contains 59.4 billion individual transistors in a piece of silicon measuring 823 square millimeters.
Aside from the benefits of scaling, Toon said the server offering will remove the need for others to build custom servers, which is an expensive process for original equipment manufacturers such as Dell. "What we're doing is we're saying, look, you can use the the servers that your customers already want to go and buy."
"You just plug our IPU machine in the rack next to that, put in as many as you need, and you've added AI processing into your system." A similar argument was made by Nvidia CEO Jensen Huang in introducing the DGX machine in June — easing the burden of Nvidia's hardware partners.
Asked by ZDNet what to do about large companies that just want to buy PCIe cards, Toon noted that in fact most customers don't swap out old cards for new ones because new cards require more power than was delivered to the old cards. Hence the appeal of a card versus a closed box is less than it would seem.
"What we're doing here is we're giving people a platform through the management system so that you can just change and set power to give you the performance that you need on the box."
Also: Nvidia Ampere, plus the world's most complex motherboard, will fuel gigantic AI models
Graphcore has internally developed a PCIe card, Toon noted, and "we could go and build that product," he said. "We're looking at it, we can go down that route," said Toon. "I think at this point, we think there's a lot of advantages in the in the IPU machine," he said, adding, "if customers really turn around and say, No no we just want a card to plug in, then we can turn on that."
For the time being, early reviews seem fairly enthusiastic. Graphcore offered quotes from customers for the M2000, including Professor Andrew Briggs of the Department of Materials at the University of Oxford, who is using the machine to speed up quantum computing work. He said the Department is "tremendously excited" about the new technology and how it will "propel us further and faster into the future of quantum computing."
Similar glowing remarks came from Laurence Berkeley National Laboratory, Oxford Nanopore, and Chinese AI company EspresoMedia. Graphcore said JP Morgan Chase said it is evaluating the system to "see if our solution can accelerate their advances in AI, specifically, in the NLP and speech recognition arenas."