Artificial Intelligence in healthcare is racist
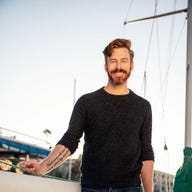
AI in healthcare has a bias problem. Last year, it came to light that six algorithms used on an estimated 60-100 million patients nationwide were prioritizing care coordination for white patients over black patients for the same level of illness.
The reason? The algorithm was trained on costs in insurance claims data, predicting which patients would be expensive in the future based on who was expensive in the past. Historically, less is spent on black patients than white patients, so the algorithm ended up perpetuating existing bias in healthcare.
Therein lies the danger of using narrow datasets in Artificial Intelligence: If the data is biased, the AI will be biased. That doesn't mean we should (or, now that the genie is out of the bottle, can) abandon AI. Which leads to an obvious question: Can using broader datasets, including socioeconomic data, reduce the influence of bias in clinical AI and correct systemic bias that persists in vital institutions like healthcare, education, and law enforcement?
Dr. John Frownfelter, Chief Medical Information Officer at Jvion, is one of the people advancing the broader dataset approach. Jvion's AI analyzes over 4,500 factors per patient so bias in any one dataset doesn't compromise the integrity of the AI's output. Jvion's AI also actively counters existing bias by flagging socioeconomic barriers to care that drive bias in the first place.
I caught up with Dr. Frownfelter to understand how developers and organizations can get the inevitable shift to AI to drive healthcare delivery right.
GN: How pervasive is AI becoming in fields that impact patient health and outcomes? Can you give us a sense of the trajectory of adoption over the past few years?
Dr. Frownfelter: The market for AI in healthcare is growing exponentially, from $600M to $6B in the last few years. Gartner predicts that by 2021, 75% of provider organizations will have invested in AI to either improve operational performance or clinical outcomes. Our own AI at Jvion is now in use at over 300 hospitals and 40 health systems, with a database encompassing 30M+ patients. So far, radiology is furthest ahead for AI in healthcare, where machine learning models are being used to detect malignancies and other abnormalities in MRIs, X-rays and other scans.
It's getting to the point now where AI will be critical to hospitals' survival in the near future, particularly as the pandemic bleeds providers of revenue, and more providers shift to value-based care models that tie their financial outcomes to improving patient outcomes. With clinical AI, it becomes possible to leverage patient data to make more informed clinical decisions that ultimately improve patient outcomes.
GN: What are the dangers of using narrow datasets in AI that affect patients? Can you point to specific examples of bias?
Dr. Frownfelter: This comes down to the training data set that is used. If there are gaps in the data used to train the AI, then these gaps will manifest in the output of the AI. For example, there is a case where facial recognition software was trained only on white people. Well, when it was put to use, it recognized people of all races, but only as white people. So the training data set will skew the output if it is not representative of the population upon which it is being used.
Using narrow datasets can also expose AI to any bias inherent to the data. In the case of racially-biased AI that was widely reported last year, the problem was that the AI conflated the patient's health risk with the amount spent on their care in previous insurance claims. Well historically, black patients are underserved by healthcare, so they have less insurance claims. That doesn't mean they have less risk, in fact, because they are underserved they have greater risk. The assumption the AI was based on was wrong, but that assumption was baked into the AI's output.
Narrow datasets, such as those that focus only on insurance claims data, can also leave out important risk factors, particularly the socioeconomic determinants of health (SDOH) that drive disparities in health outcomes in the first place. Incorporating data on these factors into AI models enables the AI to detect hidden risks that would be missed by AI models trained only on clinical data or surface-level demographics. But even with socioeconomic data, you run the risk of overgeneralizing the features of a population if your data set isn't specific enough. For example, there can be a wide variation in income, or access to nutritional food, within a zip code. To more accurately assess patients' socioeconomic or environmental risk factors, you need to look at these factors at the level of the US Census tract, or better yet, the level of US Census blocks or block groups.
GN: What are the blind spots that developers may have that lead them to use inadequate datasets? How can those be corrected for?
Dr. Frownfelter: Recent analysis showed many digital image AI algorithms are trained with data from academic institutions in just three states. This is a huge blind spot. To fix these blind spots, AI developers should use training data that reflects the population the algorithm will be applied against. Using more complete and representative data, rather than cherry picking select data points, will reduce the bias in the output. Our AI at Jvion is trained on the patient data from our customer hospitals, incorporating 30M patients in almost every state, so it's inherently representative.
GN: How can we test AI for bias? What incentive will end users have to perform such tests?
Dr. Frownfelter: We can test for bias in AI by evaluating the differences in predictions for different groups. The common areas to test are for race, age and gender. Based upon the findings, if there are differences in the AI's predictions for outcomes in different groups, the deeper question is whether those differences accurately reflect existing differences in the population, or whether those differences reflect bias within the AI model. It may be the case that the model is designed to reduce the recognition of these existing disparities between populations, but it does not necessarily mean that those differences do not exist.
If the algorithm uses social determinants of health data, it's important to validate that the weighting of different factors used in making predictions are appropriate. That means comparing the predicted distribution of outcomes with the actual distribution of outcomes relative to the risk factor in question. For example, and these are made up numbers, if an algorithm predicts patients living in an area with poor air quality will have a 20% chance of developing Chronic Obstructive Pulmonary Disease (COPD), when in reality the rate of COPD in areas with poor air quality is 35%, then air quality should be weighted more heavily in the algorithm.
Ultimately, end users, in other words clinicians, both want and need to trust that there isn't bias in the AI they use. The onus is on AI developers to demonstrate the data used to train their algorithms and assure users the data is comprehensive of risk factors and representative of the population.
GN: How is Jvion's approach different from what else is out there in the market?
Dr. Frownfelter: Jvion's approach uses broad data sets that account for thousands of risk factors and, with data from 30M patients across over 300 hospitals, are representative of the populations that we serve. We are able to map new patients over 99% of the time, regardless of race, gender or age and dozens of other characteristics as well.
Another difference is that our AI doesn't just predict which patients are at risk of experiencing an adverse outcome. It provides recommendations for how to change patients' risk trajectory toward a better outcome. By taking into account the patients' clinical, behavioral, socioeconomic and environmental circumstances, these recommendations are tailored to each patient's unique situation so that they will have a greater chance of averting negative outcomes.
Jvion's approach is also different from other solutions on the market because we allow the data to speak for itself. By that I mean that we leverage the power of machine learning to understand new associations and correlations within the data that otherwise would not be apparent. An example of this is that what appears to be differences in risk due to age are actually due to the increasing number of underlying clinical conditions that accompany us as we get older. Age is not the risk factor — it is simply a marker correlated with the real risk factors. Our approach looks at the actual risk drivers, not the markers for risk.
Finally, we also consistently validate our AI with performance modeling and that is followed by the ultimate test of measuring outcomes. On average, hospitals using our AI report average reductions in preventable harm incidents of roughly 30%, which translates to annual savings of millions of dollars.