Cerebras CEO talks about the big implications for machine learning in company’s big chip
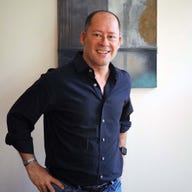
Primers
You may have heard that, on Monday, Silicon Valley startup Cerebras Systems unveiled the world's biggest chip, called the WSE, or "wafer-scale engine," pronounced "wise." It is going to be built into complete computing systems sold by Cerebras.
What you may not know is that the WSE and the systems it makes possible have some fascinating implications for deep learning forms of AI, beyond merely speeding up computations.
Cerebras co-founder and chief executive Andrew Feldman talked with ZDNet a bit about what changes become possible in deep learning.
There are three immediate implications that can be seen in what we know of the WSE so far. First, an important aspect of deep networks, known as "normalization," may get an overhaul. Second, the concept of "sparsity," of dealing with individual data points rather than a group or "batch," may take a more central role in deep learning. And third, as people start to develop with the WSE system in mind, more interesting forms of parallel processing may become a focus than has been the case up until now.
All this represents what Feldman says is the hardware freeing up design choices and experimentation in deep learning.
Cerebras's "wafer-scale engine," left, compared to a top-of-the-line graphics processing unit from Nvidia, the "V100," popular in deep learning training.
"We are proud that we can vastly accelerate the existing, pioneering models of Hinton and Bengio and LeCun," says Feldman, referring to the three deep learning pioneers who won this year's ACM Turing award for their work in deep learning, Geoffrey Hinton, Yoshua Bengio, and Yann LeCun.
"But what's most interesting are the new models yet to be developed," he adds.
"The size of the universe of models that can be trained is very large," observes Feldman, "but the sub-set that work well on a GPU is very small, and that's where things have been focused so far," referring to the graphics processing chips of Nvidia that are the main compute device for deep learning training.
The first sign that something very interesting was happening with Cerebras came in a paper posted on the arXiv pre-print server in May by Vitaliy Chiley and colleagues at Cerebras, titled "Online Normalization for Training Neural Networks." In that paper, the authors propose a change to the way machine learning networks are built, called normalization.
Also: AI is changing the entire nature of compute
Normalization is a technique to deal with a problem faced by all statistical systems: Covariate shift. The data used to train a statistical program is assumed to be essentially similar to data in the real world that a trained statistical model will encounter. Pictures of cats and dogs that a classifier encounters in the wild should be like those encountered in training data. But there are differences between the independent variables in the training, the "covariates," and those found in real data in the wild. That constitutes a shift in the distribution.
Google scientists Sergey Ioffe and Christian Szegedy pointed out in a 2015 paper that covariate shift also happens inside a network. As each training data point exits an activation unit in one layer of the network, the network parameters have transformed that data point from what it was when it entered the network. As a result, the distribution of data is transformed by the successive layers of the network -- so much so that it becomes different from the original statistics of the training data. This can lead to poor training of the network.
Ioffe and Szegedy called this change "internal covariate shift." To remedy it, they proposed what's known as "batch normalization." In batch norm, as it's known, a new layer of processing is inserted into the network. It uses the fact that data samples are processed in what's known as "mini-batches," groupings of several data samples processed by the chip at the same time. The chip takes the statistics of the batch, the mean and variance, specifically, as an approximation of the statistics in the entire data set. It then adjusts the value of the individual data point to be more in accord with those batch statistics, as a way to nudge the sample back into alignment with the "true" distribution of the population.
Batch norm brings advantages in speeding up training time, but it has problems. For one thing, it can dramatically increase the memory used in a computing system. For another, it may introduce biases into the data because the mini-batch of samples used to calculate mean and variance is not necessarily a great approximation of the data distribution in the entire population. That can mean problems when the trained network encounters real-world data, another covariate shift. Lots of follow-on approaches were proposed over the years to improve things, such as "layer normalization," "group normalization," "weight normalization," and even "re-normalization."
Now, Cerebras's team decided to propose their alternative. Instead of using a batch, the Cerebras scientists propose tracking a single sample, and "replace arithmetic averages over the full dataset with exponentially decaying averages of online samples." The process is illustrated in a network graph in the figure below. In tests on ImageNet and the like, the authors contend online normalization "performs competitively with the best normalizers for large-scale networks." (ZDNet reached out to Google's Ioffe for comment, but he declined to comment.)
A diagram of the "online normalization" proposed by Vitaliy Chiley and colleagues at Cerebras as an alternative to batch normalization.
The WSE doesn't automatically shut off batch norm; it is a flag that can be set in the processor. The WSE is designed to run any existing neural network created in TensorFlow or PyTorch and other frameworks, and it will accommodate batch norm.
Though merely an option in the WSE chip, online normalization points to a potential move away from what Feldman considers years of gumming up neural networks with tricks to please graphics processors such as those from Nvidia.
"The ways in which problems have always been attacked have gathered around them a whole set of sealing wax and string and little ways to correct for weaknesses," observes Feldman. "They seem practically to require that you do work the way a GPU makes you do work."
Feldman points out batches are an artifact of GPUs' form of parallel processing. "Think about why large batches came about in the first place," he says. "The fundamental math in neural networking is a vector times a matrix." However, "if you do that it leaves a GPU at very low utilization, like, a few percent utilized, and that's really bad."
Also: Google says 'exponential' growth of AI is changing nature of compute
So, batching was proposed to fill up the GPU's pipeline of operations. "What they did is they stacked vectors on top of each other to make a matrix-by-matrix multiply, and the stacking of those vectors is what's called a mini-batch."
All this means that batching is "not driven by machine learning theory, they are driven by the need to achieve some utilization of a GPU; it is a case of us bending our neural net thinking to the needs of a very particular hardware architecture, but that's backward."
"One of the things we are most excited about is that WSE allows you to do deep learning the way deep learning wants to be done, not shoehorned into a particular architecture," declares Feldman.
The WSE is intended for what's called small batch size, or really, "a batch size of one." Instead of jamming lots of samples through every available circuit, the WSE has hard-wired circuitry that only begins to compute when it detects a single sample that has non-zero values.
Cerebras Systems co-founder and CEO Andrew Feldman.
The focus on sparse signals is a rebuke to the "data parallelism" of running multiple samples, which, again, is an anachronism of the GPU, contends Feldman. "Data parallelism means your individual instructions will be applied to multiple pieces of data at the same time, including if they are zeros, which is perfect if they are never zeros, like in graphics.
"But when up to 80% is zero, as in a neural network, it's not smart at all -- it's not wise." He notes that in the average neural network, the "ReLU," the most common kind of activation unit for an artificial neuron, has "80% zeros as an output."
Being able to handle sparse signals looks to be an important direction for deep learning. In a speech to a chip conference in February, the International Solid State Circuits Conference, Facebook's head of AI research, Yann LeCun, noted that "As the size of DL systems grows, the modules' activations will likely become increasingly sparse, with only a subset of variables of a subset of modules being activated at any one time."
That's closer to how the brain works, contends LeCun. "Unfortunately, with current hardware, batching is what allows us to reduce most low-level neural network operations to matrix products, and thereby reduce the memory access-to-computation ratio," he said, echoing Feldman.
"Thus, we will need new hardware architectures that can function efficiently with a batch size of one."
If traditional data parallelism of GPUs is less than optimal, Feldman contends WSE makes possible a kind of renaissance of parallel processing. In particular, the other kind of parallelism can be explored, called "model parallelism," where separate parts of the network graph of deep learning are apportioned to different areas of the chip and run in parallel.
"The more interesting thing would be to divide up work so that some of your 400,000 cores work on one layer, and some on the next layer, and some on the third layer, and so on, so that all layers are being worked on in parallel," he muses. One effect of that is to vastly multiply the size of the parameter state that can be handled for a neural network, he says. With a GPU's data parallelism, any one GPU might be able to handle a million parameters, say. "If you put two GPUs together [in a multi-processing system], you get two machines that can each handle a million parameters," he explains, "but not a machine that can handle 2 million parameters — you don't get a double."
With the single WSE, it's possible to support a four-billion parameter model. Cluster the machines together, he suggests, and "you can now solve an eight-billion or 16-billion parameter network, and so it allows you to solve bigger problems by adding resources."
Feldman sees things like sparsity and model parallelism taking neural nets "beyond what the founding fathers gave us 20 or 30 years ago," meaning, Hinton, Bengio, and Lecun. Modern networks such as Google's "Transformer," he says, Already "contemplate vast compute in their clusters of TPUs," referring to the "Tensor Processing Unit" chip developed by Google.
"The hardware is warping the progress of our industry," is how he sums state of the art. "When limitations of the hardware are keeping us from exploring fertile areas, that's very much what we sought to change; HW should not get in the way of your exploration, it shouldn't drive you to a certain set of techniques like large batch size.
"Hardware should be a platform on which your thinking can take shape."