Cheap Australian robot uses 7 images to ID Amazon products
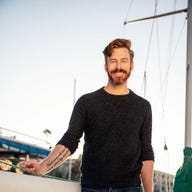
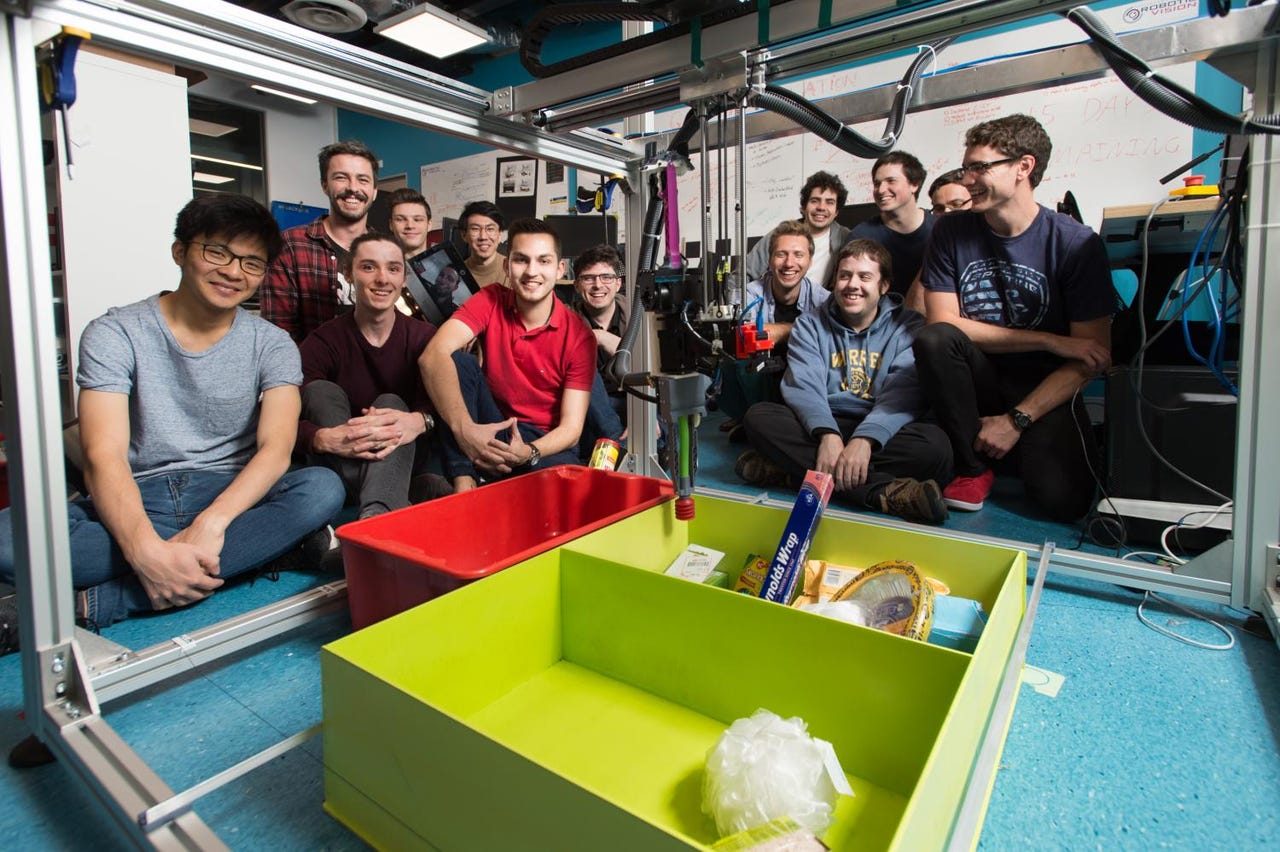
Modern robots can't reliably identify and grasp a wide range of objects from shelves in large fulfillment centers, such as those used by Amazon. That simple fact has preserved tens of thousands of low-wage, low-skill jobs amid widespread automation in the industry.
But those days are numbered. Every year, Amazon holds a challenge for robotics researchers to test the best pick-and-place robots. The Prime company's motivation couldn't be clearer--eliminating human pickers gets Amazon very near the lights-out warehouse its been driving toward since it acquired Kiva Systems, a developer of logistics robots, in 2012.
This year's winner is a bot named Cartman from Australia, and it's garnering attention not just for its adroitness at identifying objects, but for its price tag. The bot is built with largely off-the-shelf components and cost the team around $24,000. That's hardly a song, but for a custom industrial robot it's quite cheap.
Photos of objects the robot contestants were asked to identify
Cartman was created by the Australian Centre for Robotic Vision. Last year, the team used a common collaborative industrial robot called Baxter. This year, befitting the organization's name, the strategy was to invest heavily in the vision-based algorithms while keeping the mechanics as bare-bones as possible.
Unlike its competitors, Cartman is not an actuated robotic arm. It bears a closer resemblance to an arcade grasper, the kind that yanks (or fails to) stuffed animals inside that glass cube.
Attached to the three-dimensional slider are suction cups and a two-fingered gripper, allowing Cartman to grasp a large diversity of small items.
The multi-step test required bots to correctly identify prescribed objects from an array of options and place them correctly.
"We needed seven images of each unseen item for us to be able to detect them," said Anton Milan to Australian reporters.
Deep learning researchers have rapidly improved how quickly machines can identify objects from static images, a necessary skill for many kinds of industrial and, in the near future, home robots.
Google DeepMind researchers created a system that learns from a large image database to correctly identify new objects based on a single image. But the Australian Centre for Robotic Vision's machine is calibrated for real-world use. In a warehouse, objects might be damaged or obscured on shelves, so it's harder for systems to make determinations based on fewer examples.
The Amazon Robotics Challenge began in 2015. This year, 16 teams from 10 countries competed. The victorious Australian team took home an $80,000 prize. No word if Amazon has plans to acquire the technology.