Could a strange new memory chip unlock mysteries of AI?
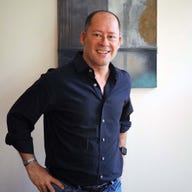
Modern artificial intelligence lacks a strong theoretical basis, and so it's often a shrug of the shoulders why it works at all (or, oftentimes, doesn't entirely work).
One of the deepest mysteries of deep learning is one of its most brilliant successes, what's known as stochastic gradient descent. Stochasticity, the process of randomly picking out examples of data, has yielded breakthroughs in image recognition and other deep learning tasks.
And now, one computer chip company thinks they may have a kind of machine for stochasticity, a chip whose power comes from randomness. It might not lead to a theory of why machine learning works, but it might lead to knew breakthroughs in what stochasticity can achieve.
Eight-year-old Spin Memory, based in Fremont, California, is advancing the state of the art in a form of computer-memory chip known as "MRAM," for "magnetoresistive random access memory." The company has gotten $158 million in three funding rounds to produce a recipe that can be used by chip makers to add more efficient blocks of memory to their parts, what are known in the industry as data caches.
The first chips using Spin Memory's recipe for MRAM data storage may appear at the end of 2021, in the form of replacements for the traditional "Level 3" cache in a processor.
An MRAM cache could be far less power-hungry than the SRAM used by chip makers to make conventional caches, and it could provide several times the amount of storage in the same physical area of a chip. But only if Spin can successfully exploit MRAM's inherent stochasticity.
"There's something highly unusual about MRAM, and it goes to the very nature of AI itself, the stochastic nature of it," says Andy Walker, Spin's vice president of products. "There is a mathematical relationship in its functioning that is directly applicable to AI."
What Walker is referring to is the complementary nature of the electrical operation of MRAM, on the one hand, and the ability of neural networks to function even as their "precision" in ones and zeros is reduced.
Also: AI is changing the entire nature of compute
MRAM stores a one or a zero by aligning the orientation of the magnetic fields of two magnets relative to one another via an electrical current. When an electrical current is passed through the first magnet, the electrons in the current become polarized, meaning that they take on what's called an "angular momentum." When the current is sent through the second magnet, their angular momentum causes torque in the second magnet, and the torque changes the magnetic orientation of the second magnet. That change in orientation represents the flipping of a bit from zero to one, or vice-versa.
That switching function isn't assured, a bit might flip or it might not. It is "a probabilistic value of the voltage," explains Walker. In order to make sure a bit is flipped, to write a one or a zero with certainty, extra amounts of voltage have to be applied. That drives up power consumption of the device, a bad thing, and also reduces the lifetime of MRAM by constantly stressing the device.
"There's something highly unusual about MRAM, and it goes to the very nature of AI itself, the stochastic nature of it," says Andy Walker, Spin's vice president of products.
"The way that the industry has till now worked with MRAM is to pound on it with a long voltage for a long time," says Walker. "They hide this stochastic switch, at the expense of the endurance of the switch."
Instead, Walker and colleagues decided to make a virtue out of that vice. Taking advantage of the ability of neural nets to deal with randomness, they lowered the voltage of MRAM below what would be necessary for reliable storage of ones and zeros. They decided to let the stochastic nature of neural nets make up for the uncertainty of the MRAM.
Spooky action: convolutional neural nets Such as LeNet-4 continued to function at high accuracy even when Spin Memory scientists turned up the "bit error rate" in the system.
"What we have done is we have gone into this area of code design where you understand the physics of the switch, and we incorporated that into the design."
In a paper posted on the arXiv pre-print server in May, Walker and colleagues describe in stunning detail a kind of correspondence of MRAM stochasticity and neural net stochasticity. They took different versions of classic convolutional neural networks, including LeNet-4, and AlexNet, and "binarized" them, meaning, they made the "activation function" of the neural nets either a one or a zero, instead of a numerical value. This approach was first developed in 2016 by AI scholar Yoshua Bengio and colleagues as a way to reduce the compute intensity of neural nets by reducing their numerical precision.
Walker and colleagues find that such binarized networks can still function with a high degree of accuracy even when a large amount of "bit error rate" is injected into the networks. In certain instances, they were able to make as much as a third of the bits incorrect, and still reach conventional levels of accuracy in image recognition tests such as "CIFAR 10."
In Walker's terms, "this unusual stochastic nature of the switch" in MRAM becomes "something spookily relevant to the nature of AI itself."
By tolerating greater error in MRAM, Spin is able to lower the operating voltage, and thereby close the gap between MRAM and SRAM in two important respects, the speed and endurance of the circuits. By eliminating the shortcomings of MRAM, Spin can then exploit inherent advantages that MRAM has over SRAM, namely, feature size and its non-volatile storage capability.
"Longer term, we see a market for standalone memory," says Jeff Lewis, Spin's vice president of business development.
Further technical detail about Spin's part can be found in a patent application published in June.
A lot more memory can be squeezed into the same area of a CMOS chip with MRAM compared to SRAM, on the order of three or four times more.
"In terms of the layout of the [SRAM memory] cell, it's extremely large, six transistors," observes Walker of SRAM. Since the chip industry move to vertical transistors several years back, called "finfet," SRAM is "extremely difficult to scale" in density, so that SRAM has an increasing problem of size in chips whose feature sizes are 10 nanometers or smaller.
A second advantage is that MRAM is non-volatile, so that it can store a one or a zero even when the power is turned off, similar to flash memory. That could be a big energy savings over SRAM because SRAM leaks. It uses energy even when it's not doing any work. As Walker puts it, "AI demands much more memory on the die, and that leads to a huge amount of static leakage, on the order of amps of power being consumed even when the chip is doing nothing."
The focus initially is to replace the so-called "Level 3" cache in processors, says Spin's Vice President of business development, Jeff Lewis. Those caches can be several megabytes of SRAM per chip, and up to tens of megabytes in the case of top-of-the line processors, such as Intel's "Xeon" line.
Spin is working closely with chip equipment maker Applied Materials to make fabrication of MRAM a readily available, predictable manufacturing task for most chip makers.
"Longer term, we see a market for standalone memory," says Lewis, as a replacement for the DRAM chips that serve as main memory for computer systems. "To go into that, we have to have these first improvements, and then make it very high density, so that the costs will be similar to DRAM, on the order of multiple gigabytes at DRAM-like costs."
The first chips from any company using Spin's memory recipe are expected to emerge late next year, or in early 2021. They may be followed by standalone memory parts sometime later in 2021.
Walker is even more enthusiastic about an ongoing fusion of AI with MRAM. Where stochasticity has been an obstacle for MRAM, it could become an asset if it can be harnessed for AI.
"At some point, it turns into a feature, a unique feature of MRAM," he says. To educate people about features such as that is difficult, but the increasing involvement of physicists in AI may help, he offers. "Having physicists getting interested in this area makes it extremely powerful because not many people have that combination of knowledge, to understand across the span of things."