ExtraHop Addy: analytics at the edge, anomaly detection in the cloud
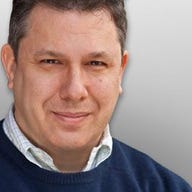
ExtraHop, an analytics startup based in Seattle, has long had a unique approach to analytics: its technology works by collecting data directly from network packets.
When I first became acquainted with ExtraHop, I assumed that packet-level data collection was aimed squarely at machine/operational analytics. But while ExtraHop does indeed excel in that arena, getting data in real time hot off the network, the technology goes well beyond machine-level metrics. ExtraHop uses industry- and use case-specific domain expertise to recognize what kind of data is contained in the packets, and so can perform industry- and scenario-specific analytics as well. This is especially the case with, but not limited to, analytics use cases in the healthcare arena.
Also read: ExtraHop introduces streaming data appliance
But now ExtraHop has taken that a step further, to perform not only descriptive analytics, but also machine learning-based anomaly detection, with a service called Addy. The service, which was announced February 14th, takes ExtraHop's semantic understanding of data a step further to create a highly-optimized solution.
Finding outliers
Addy uses an architecture that combines the existing ExtraHop Discover appliance for data collection, with a cloud service for scoring data against machine learning models, to identify anomalies and alert customers to them. That means that whatever data ExtraHop may be tracking -- whether it be operational data like network operations, or industrial data like healthcare claims processing performance -- machine learning models in the cloud can quickly detect data that seems out of step with the norm.
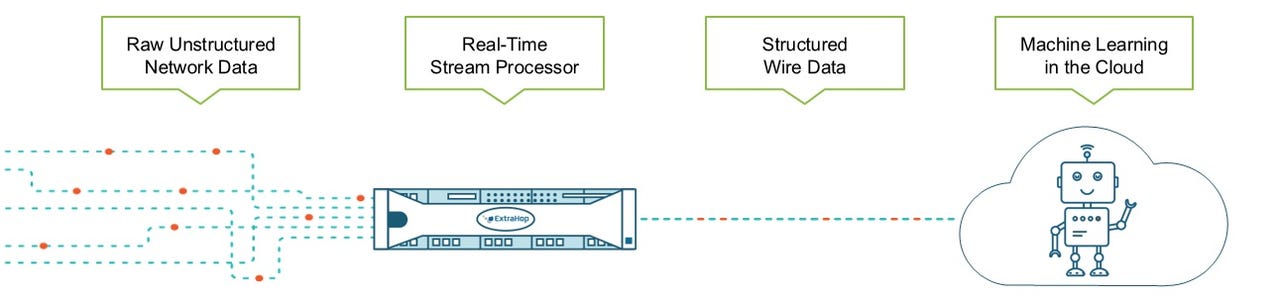
Addy's high-level architecture
While that sounds common-sense enough, and while machine learning technology can make the anomaly detection work relatively easy, there's still a major challenge to getting this to work: the massive amount of data involved due to packet-level data collection, and the resulting "data gravity" costs of moving all that data to the cloud, and scoring it against machine learning models efficiently.
Context-sensitive triage
But ExtraHop seems to have solved this puzzle and done so in a clever and gratifying way. The company has applied its semantic understanding of network packet data, along with some other techniques, to streamline the data payloads it needs to push to the cloud. And it's done this at two different levels.
First, ExtaHop applied the same principal to analytics that underlies columnar databases: that although a broad set of data attributes may be captured together, it's only a subset of those attributes that need to be tracked, aggregated and inspected. And since ExtraHop already understands the semantic structure of the data it collects, that insight can be extended to identify which data within that structure will be relevant to upstream anomaly detection work.
This approach greatly narrows the data, essentially reducing the number of columns that need to be collected and analyzed. And the accuracy of these determinations will keep improving, as customers can "upvote" which particular metrics are significant and important to them. In doing this, ExtraHop has essentially crowd-sourced the so-called feature engineering for its machine learning models, adding in a layer of human intelligence to aid in triage and due diligence on accuracy. This brings data science and common sense together in striking efficiency.
Slimming down
The second principal of analytics that is germane here is that analyzed data begins as a set of individual, granular "fact" data which then needs to be grouped and aggregated. Because of this, the ExtraHop software can and does a great deal of aggregation work at the point of data collection, before the data is sent up to the machine learning infrastructure in the cloud. This approach greatly reduces the number of rows that need to be processed and scored against the machine learning models.
The result: a very clever and optimized approach to collecting massive volumes of time series data right off the network, then mapping and reducing (pun intended) that data into something much more compact. This approach greatly reduces the data gravity involved, making the anomaly detection work much more reasonable and practical. The cloud-based model scoring can then be done in relatively short order and the results can even be round-tripped back to the ExtraHop appliance on-premises, uniting the anomaly detection with the original packet-derived data.
Back to basics
ExtraHop's work here has bearing on a broader set of data analytics scenarios. First off, if you think about it, what the company has done with its domain-specific knowledge is to implement a very aggressive kind of data compression that mitigates data gravity and adds efficacy to a hybrid on-prem/cloud approach to analytics. Certainly, this kind of "compression" is "lossy," but that's very much by design, as analytics is all about getting insight from an important subset of raw data that is collected. With machine learning, this is even more the case.
No matter how fast the Internet gets, no matter how cheap storage, memory and computing power become, new advances and applications will introduce conditions that stress capacity, so optimization never gets old-fashioned. In tech wave after tech wave, the industry seems to forget and rediscover this. ExtraHop has avoided forgetting it in the first place.
Secondly, though, ExtraHop's architecture is instructive for scenarios beyond its own implementation. It illuminates the importance of intelligent "edge" computing, and that has significant ramifications for IoT (the Internet of Things). Most IoT devices are fairly dumb, having just enough power to host sensors and transmit readings from them over the network, back to a much more intelligent hub. This leads to a kind of data "pollution" and to the resultant problem of cleaning it up. Moving all that data around is costly. Filtering that data, down to relevant subsets, and doing that as closely as possible to the point of data collection, is key to enabling machine learning capabilities and making them scalable.
Broader significance
There's irony here, in that a company whose approach is based on collecting the most granular, raw, unprocessed data available is also focused on slimming it down and adding as much value to it as possible, as quickly as possible. That yin and yang approach is what's most intriguing about the Addy product. And that approach needs to become much more widespread if the industry is going to reconcile working with Big Data on the one hand, and generating focused, pithy insights on the other.
This post was updated on March 6th to clarify that ExtraHop's Discover appliance participates in the Addy architecture; originally the article stated that it was ExtraHop's Explore appliance that did so.