Google DeepMind gamifies memory with its latest AI work
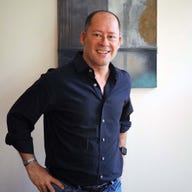
You know when you've done something wrong, like putting a glass too close to the edge of the table, only to accidentally knock it off the table a moment later. Over time, you realize the mistake even before disaster strikes.
Likewise, you know over years when you made the wrong choice, like choosing to become a manager at Best Buy rather than a pro-ball player, the latter of which would have made you so much more fulfilled.
That second problem, how a sense of consequence develops over long stretches, is the subject of recent work by Google's DeepMind unit. They asked how they can create something in software that is like what people do when they figure out the long-term consequences of their choices.
DeepMind's solution is a deep learning program they call "Temporal Value Transport." TVT, for shorthand, is a way to send back lessons from the future, if you will, to the past, to inform actions. In a way, it's "gamifying" actions and consequence, showing that there can be a way to make actions in one moment obey the probability of later developments to score points.
They are not creating memory, per se, and not recreating what happens in the mind. Rather, as they put it, they "offer a mechanistic account of behaviors that may inspire models in neuroscience, psychology, and behavioral economics."
The "Reconstructive Memory Agent" uses multiple objectives to "learn" to store and retrieve a record of past states of affairs as a kind of memory.
The authors of the paper, "Optimizing agent behavior over long time scales by transporting value," which was published November 19th in Nature Magazine's Nature Communications imprint, are Chia-Chun Hung, Timothy Lillicrap, Josh Abramson, Yan Wu, Mehdi Mirza, Federico Carnevale, Arun Ahuja, and Greg Wayne, all with Google's DeepMind unit.
The point of departure for the game is something called "long-term credit assignment," which is the ability of people to figure out the utility of some action they take now based on what may be the consequences of that action long into the future — the Best Buy manager-versus-athlete example. This has a rich tradition in many fields. Economist Paul Samuelson explored the phenomenon of how people make choices with long-term consequences, what he termed the "discounted utility" approach, starting in the 1930s. And Allen Newell and Marvin Minsky, two luminaries of the first wave of AI, both explored it.
Of course, AI programs have a form of action-taking that is based on actions and consequences, called "reinforcement learning," but it has sever limitations, in particular, the fact it can't make correlations over long time scales the way it seems people are doing with long-term credit assignment.
"Humans and animals evidence behaviors that state-of-the-art (model-free) deep RL cannot yet simulate behaviorally," write Hung and colleagues. In particular, "much behavior and learning takes place in the absence of immediate reward or direct feedback" in humans, it appears.
DeepMind's version of reinforcement learning that uses "temporal value transport" to send a signal from reward backward, to shape actions, does better than alternative forms of neural networks. Here, the "TVT" program is compared to "Long-short-term memory," or LSTM, neural networks, with and without memory, and a basic reconstructive memory agent.
DeepMind's scientists have made extensive use of reinforcement learning for their massive AI projects such as the AlphaStar program that is notching up wins at Starcraft II, and the AlphaZero program before it that triumphed at go and chess and shoji. The authors in the new work adapt RL so that it takes signals from far in the future, meaning, several time steps forward in a sequence of operations. It uses those signals to shape actions at the beginning of the funnel, a kind of feedback loop.
Also: Google's StarCraft II victory shows AI improves via diversity, invention, not reflexes
They made a game of it, in other words. They take simulated worlds, maps of rooms like you see in video games such as Quake and Doom, the kind of simulated environment that has become familiar in training of artificial agents. The agent interacts with the environment to, for example, encounter colored squares. Many sequences later, the agent will be rewarded if it can find its way to that same square using a record of the earlier exploration that acts as memory.
How they did it is a fascinating adaption of something created at DeepMind in 2014 by Alex Graves and colleagues called the "neural Turing machine." The NMT was a way to make a computer search memory registers based not on explicit instructions but based simply on gradient descent in a deep learning network — in other words, learning the function by which to store and retrieve specific data.
The authors, Hung and colleagues, now take the approach of the NMT and, in a sense, bolt it onto normal RL. RL in things like AlphaZero searches a space of potential rewards to "learn" via gradient descent a value function, as it's called, a maximal system of payoffs. The value function then informs the construction of a policy that directs the actions the computer takes as it progresses through states of the game.
To that, the authors add an ability for the RL program to retrieve memories, those records of past actions such as encountering the colored square previously. This they call the "Reconstructive Memory Agent." The RMA, as it's called, makes use of that NMT ability to store and retrieve memories by gradient descent. Incidentally, they break new ground here. While other approaches have tried to use memory access to help RL, this is the first time, they write, that the so-called memories of past events are "encoded." They're referring to the way information is encoded in a generative neural network, such as a "variational auto-encoder," a common approach of deep learning that underlies things such as the "GPT2" language model that OpenAI built.
Also: Fear not deep fakes: OpenAI's machine writes as senselessly as a chatbot speaks
"Instead of propagating gradients to shape network representations, in the RMA we have used reconstruction objectives to ensure that relevant information is encoded," is how the authors describe it.
The final piece in the puzzle is that when a task does lead to future rewards, the TVT neural network then sends a signal back to the actions of the past, if you will, shaping how those actions are improved. In this way, the typical RL value function gets trained on the long-term dependency between actions and their future utility.
The results, they show, beat typical approaches to RL that are based on "long-short-term memory," or LSTM networks. Meaning, the DeepMind combo of RMA and TVT beats the LTSMs, even those LSTMs that make use of memory storage.
It's important to remember this is all a game, and not a model of human memory. In the game, DeepMind's RL agent is operating in a system that defies physics, where events in the future that earn a reward send a signal back to the past to improve, or "bootstrap" actions taken previously. It's as if "Future You" could go back to your college-age self and say, Take this route and become a pro-ball player, I'll thank me later."
One approach that might make all this more relevant to human thought, an approach not entertained by the authors, would be to show how TVT can achieve some kind of transfer learning, meaning, can the learning that happens be used in new, unseen tasks of a totally different setting.
The authors end by acknowledging this is a model of a mechanism, and not necessarily representative of human intelligence.
"The complete explanation of how we problem solve and express coherent behaviors over long spans of time remains a profound mystery," they write, "about which our work only provides insight."
And yet they do believe their work may contribute to exploring mechanisms that underly though: "We hope that a cognitive mechanisms approach to understanding inter-temporal choice—where choice preferences are decoupled from a rigid discounting model—will inspire ways forward."