Google's MedPaLM emphasizes human clinicians in medical AI
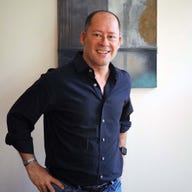

Most applications of artificial intelligence in medicine have failed to make use of language, broadly speaking, a fact that Google and its DeepMind unit addressed in a paper published in the prestigious science journal Nature on Monday.
Their invention, MedPaLM, is a large language model like ChatGPT that is tuned to answer questions from a variety of medical datasets, including a brand new one invented by Google that represents questions consumers ask about health on the Internet. That dataset, HealthSearchQA, consists of "3,173 commonly searched consumer questions" that are "generated by a search engine," such as, "How serious is atrial fibrillation?"
Also: Google follows OpenAI in saying almost nothing about its new PaLM 2 AI program
The researchers used an increasingly important area of AI research, prompt engineering, where the program is given curated examples of desired output in its input.
In case you were wondering, the MedPaLM program follows the recent trend by Google and OpenAI of hiding the technical details of the program, rather than specifying them as is the standard practice in machine learning AI.
Google's MedPaLM builds on top of a version of its PaLM language model, Flan-PALM, with the help of human prompt engineering.
The MedPaLM program saw a big leap when answering the HealthSearchQA questions, as judged by a panel of human clinicians. The percentage of times its predictions were in accord with medical consensus beat the 61.9% score for a variant of Google's PaLM language model, achieving 92.6%, just shy of the human clinician's average, 92.9%.
However, when a group of laypeople with a medical background were asked to rate how well MedPaLM answered the question, meaning, "Does it enable them [consumers] to draw a conclusion," 80,3% of the time MedPaLM was useful, versus 91.1% of the time for human physicians' answers. The researchers take that to mean that "considerable work remains to be done to approximate the quality of outputs provided by human clinicians."
Also: 7 advanced prompt-writing tips you need to know
The paper, "Large language models encode clinical knowledge," by lead author Karan Singhal of Google and colleagues, focuses on using so-called prompt engineering to make MedPaLM better than the other large language models.
MedPaLM is a derivative of PaLM-fed question-and-answer pairs provided by five clinicians in the US and UK. Those question- answer pairs, just 65 examples, were used to train MedPaLM via a series of prompt engineering strategies.
The typical way to refine a large language model such as PaLM, or OpenAI's GPT-3, is to feed it "with large amounts of in-domain data," note Singhal and team, "an approach that is challenging here given the paucity of medical data." Instead, for MedPaLM, they rely on three prompting strategies.
MedPaLM significantly outperforms Flan-PaLM in human evaluations, though it still falls short of human clinicians' abilities.
Prompting is the practice of improving model performance "through a handful of demonstration examples encoded as prompt text in the input context." The three prompting approaches are few-shot prompting, "describing the task through text-based demonstrations"; so-called chain of thought prompting, which involves "augmenting each few-shot example in the prompt with a step-by-step breakdown and a coherent set of intermediate reasoning steps towards the final answer"; and "self-consistency prompting," where several outputs from the program are sampled and a majority vote indicates the right answer.
Also: Six skills you need to become an AI prompt engineer
The heightened score of MedPaLM, they write, shows that "instruction prompt tuning is a data-and parameter-efficient alignment technique that is useful for improving factors related to accuracy, factuality, consistency, safety, harm, and bias, helping to close the gap with clinical experts and bring these models closer to real-world clinical applications."
However, "these models are not at clinician expert level on many clinically important axes," they conclude. Singhal and team suggest expanding the use of expert human participation.
"The number of model responses evaluated and the pool of clinicians and laypeople assessing them were limited, as our results were based on only a single clinician or layperson evaluating each response," they observe. "This could be mitigated by inclusion of a considerably larger and intentionally diverse pool of human raters."
Also: How to write better ChatGPT prompts
Despite the shortfall by MedPaLM, Singhal and team conclude, "Our results suggest that the strong performance in answering medical questions may be an emergent ability of LLMs combined with effective instruction prompt tuning."