Graphcore brings new competition to Nvidia in latest MLPerf AI benchmarks
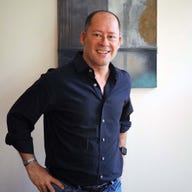
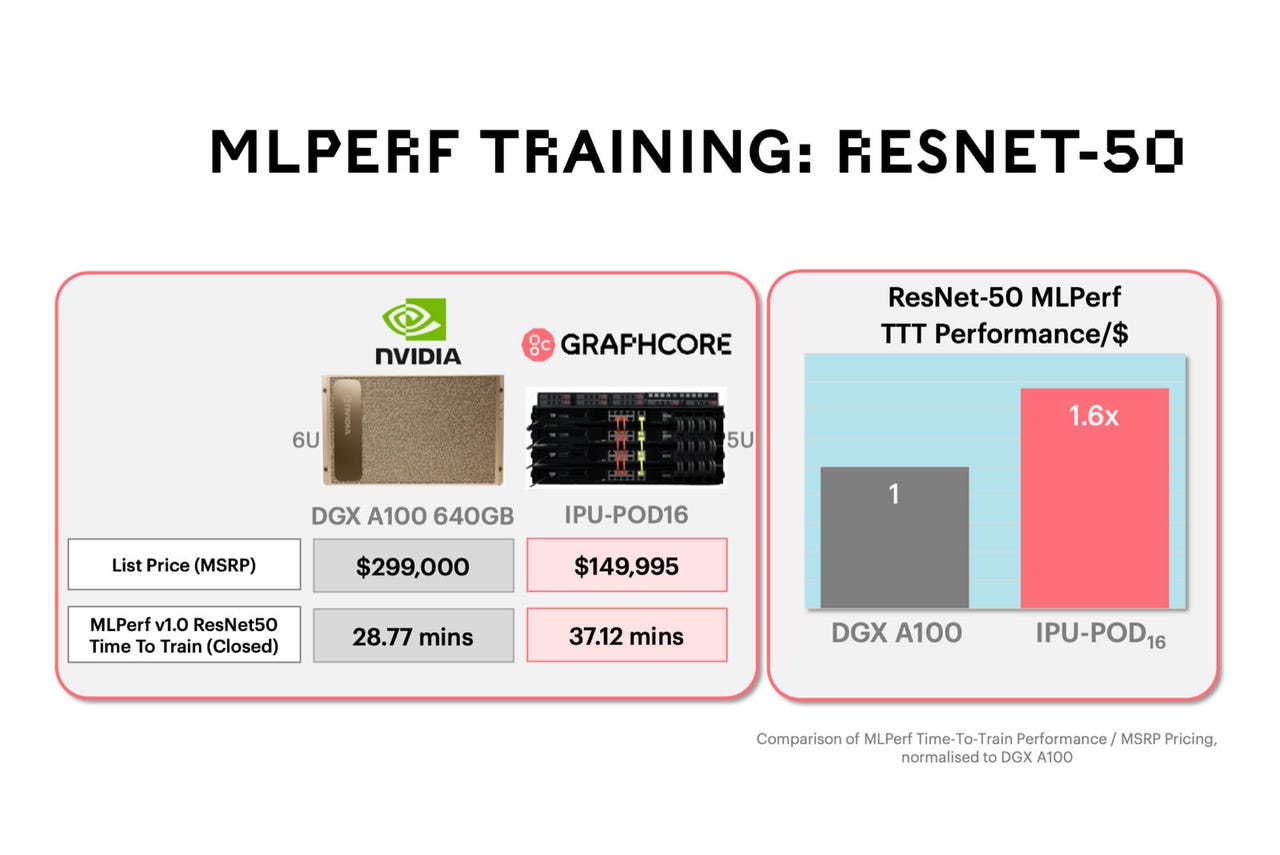
MLPerf, the benchmark suite of tests for how long it takes to train a computer to perform machine learning tasks, has a new contender with the release Wednesday of results showing Graphcore, the Bristol, U.K.-based startup, notching respectable times versus the two consistent heavyweights, Nvidia and Google.
Graphcore, which was founded five years ago and has $710 million in financing, didn't take the top score in any of the MLPerf tests, but it reported results that are significant when compared with the other two in terms of number of chips used.
Moreover, when leaving aside Google's submission, which isn't commercially available, Graphcore was the only competitor to enter into the top five commercially available results alongside Nvidia.
"It's called the democratization of AI," said Matt Fyles, the head of software for Graphcore, in a press briefing. Companies that want to use AI, he said, "can get a very respectable result as an alternative to Nvidia, and it only gets better over time, we'll keep pushing our system."
"Nvidia are what we're going after," he said. "We have to be that alternative to Nvidia."
The MLPerf test suite is the creation of the MLCommons, an industry consortium that issues multiple annual benchmark evaluations of computers for the two parts of machine learning, so-called training, where a neural network is built by having its settings refined in multiple experiments; and so-called inference, where the finished neural network makes predictions as it receives new data.
The results released Wednesday were for training.
Graphcore had previously been among a group of startups in AI that includes Cerebras Systems and SambaNova Systems who were refuseniks, sitting out the benchmarks, claiming it was not worth the effort. Cerebras co-founder and CEO, Andrew Feldman, famously told ZDNet in 2019 that the company "did not spend one minute working on MLPerf."
Also: To measure ultra-low power AI, MLPerf gets a TinyML benchmark
For Graphcore, the test has finally become too important to ignore. "We were a little reluctant to contribute," said Fyles. But, he said, the company realized "we have to come out, we have to show our second generation is competitive and plays in all the rules and boxes and spaces as others play in," said Fyles, referring to the company's Mk2 version of its Intelligence Processing Unit chip, or IPU, that is the alternative to Nvidia's GPU.
Also: Cerebras did not spend one minute working on MLPerf, says CEO
"Customers ask us for a comparison to Nvidia, they don't ask us for a comparison to anyone else," said Fyles.
Indeed, the entire benchmark suite of MLPerf stems from a basic unit of comparison that could be called An Nvidia, just like a meter or a Kelvin. The benchmark tasks that the contestants run are selected as those tasks which would take, on average, a week to train on one of Nvidia's older V100 GPUs.
The results are a bit of a David and Goliath situation, as Graphcore trailed gigantic, supercomputer-sized systems from both Nvidia and Google that make use of thousands of chips. Such systems show the absolute bleeding edge in speed that can be achieved by engineered computers from the dominant vendors.
For example, to train the BERT natural language model, a neural network that produces human-like text, the top result took Google's "TPU" chip only 17 seconds to train the program to proficiency. Nvidia's top machine took nineteen seconds. Less time is better, in this benchmark.
At twelve minutes, Graphcore was well down the list. However, the Graphcore system was composed of only two AMD EPYC processors and 64 of Graphcore's IPU chips. Google's two machines were composed of 3,456 of its TPUs plus 1,728 of AMD's EPYC processors, for one, and 2,048 TPUs and 1,024 EPYCs in another.
Nvidia's top results used 4,096 of its latest GPU, the A100, and 1,024 EPYCs in one system, and 1,024 A100s and 256 EPYCs in another. (All machines with special-purpose accelerators also come with a host microprocessor, which is responsible for a variety of things such as dispatching machine learning tasks to the accelerators.)
Graphcore's BERT score was the fastest time for a two-processor AMD system, with the next-closest competitor, an Nvidia-based system, taking a full twenty-one minutes, though that system used only 8 of Nvidia's A100 chips.
You can see all of the results in one big spreadsheet posted by MLCommons.
That fact that Graphcore's system gets by with not just fewer IPU chips but also fewer AMD host processors is meaningful to the company. Graphcore has emphasized that its IPU chip can scale independent of the number of host microprocessors, to place the horsepower where it's needed.
In a separate version of the BERT benchmark, known as the "Open" submissions, where submitters are allowed to tweak their software code to produce non-standard implementations of the neural network, Graphcore was able to reduce its training time on BERT to just over nine minutes.
Similarly, on an image recognition test called ImageNet, using the standard ResNet-50 neural network, the Graphcore system came in fourth, taking 14.5 minutes to train the system, versus the top-place result of 40 seconds for the Nvidia computer. But the Graphcore machine relied on only 8 AMD CPUs and 64 of Graphcore's IPUs, versus 620 AMD chips and 2,480 of Nvidia's A100 parts.
The competition from Graphcore is made more interesting by the fact that Graphcore's system is currently shipping, the only processor architecture other than the Nvidia systems that is actually for sale. Google's TPU machines are a "preview" of forthcoming technology. Another set of submissions that took top marks on BERT and ImageNet, from the scientific research institute Peng Cheng Laboratory, in Shenzhen, Guangdong, China, is considered a research project, and is not actually available.
Graphcore emphasized to reporters the economic advantage of having respectable results even if they are not the absolute fastest. The company compared the cost of its IPU-POD16, at $149,995 based on one quote, to what it estimates to be the $299,000 of the closest comparable Nvidia submission, an eight-GPU system called a DGX A100.
The Nvidia system scored better on BERT than the IPU system, roughly 21 minutes versus 34 minutes for Graphcore, but to Graphcore, the economic advantage far outweighs the time difference.
"These are systems that are now delivering a much better cost at a very high performance," said Fyles. "When we get to our scale-up submissions we will get to some of these very low numbers," referring to the top scores
Graphcore's largest system at the moment, the IPU-POD64, is composed of 64 separate accelerator chips. The company plans to offer models with 128 and 256 chips this year. The company expects to make those larger systems part of its entries in future. Fyles noted the IPU-POD can have as many as 64,000 IPU chips.
For the moment, "We can bring more accelerators in the same price you pay for a DGXA100, that's our message," said Graphcore's Fyles. The company characterizes that economic equation as a "time to train performance per dollar," arguing it is 1.3x what a person gets with the DGX.
However, Nvidia told ZDNet Graphcore is "cherry picking" its comparisons.
"It's not true," said Paresh Kharya, Nvidia senior director of product management and marketing, regarding Graphcore's economic comparison.
The company pointed to a comparable 8-way A100 system, also with two AMD chips, from Supermicro, that costs only $125,000, but that scored better than the Graphcore IPU-POD16 on both ResNet-50 and BERT. Cost can vary quite a bit across the numerous DGX vendors, Kharya points out.
"This is really a case of apples and oranges," he said of Graphcore. "They are comparing a 16-chip system to our 8-chip system, and even with their 16 chips, they are still slower."
Nvidia, Kharya added, has the advantage of having performance across all eight tests on the MLPerf benchmark, an indication of the breadth of applicability of the machine.
"Customers are not just deploying the infrastructure to do one thing, BERT and ResNet-50; they are deploying to run their infrastructure for five years," said Kharya. "ROI comes from a few things, the ability to run many different things, to have high utilization, and to have the software being highly productive."
"If you can only run a couple of things, you have to price it lower to entice customers to buy," he said.
For Nvidia, the benchmarks confirm a solid lead in the absolute fastest times for commercially available systems. The company noted its speed-up across all eight tasks of MLPerf, emphasizing what it calls the "relative per-chip performance at scale" when the scores of all submissions are divided by the number of chips used.
Nvidia's gains build upon not just gigantic GPU chips such as A100, but many years of refining its software capabilities. Several techniques were highlighted in the benchmark performance, including software that Nvidia wrote for distributing tasks efficiently among chips, such as CUDA Graphs and SHARP.
Graphcore has made progress with its own software, Poplar, as shown in the Open results submitted, though the software platform is still many years younger than Nvidia's, as is the case for all the startups.
For Google, the ultimate bragging rights come in having "continued performance leadership," as the company's Google Cloud researchers phrased it in a blog post. Google's preview of its TPU version 4 claimed the top results in four of the six tests for which Google competed. As with Graphcore, Google focused its comparison on Nvidia's results.
Also: Chip industry is going to need a lot more software to catch Nvidia's lead in AI
The battle will continue this year with more benchmark results, as Graphcore plans to go once more into the breach in the other part of MLPerf, inference, said Fyles.
Aside from Graphcore's entry, the latest MLPerf work is noteworthy on several other fronts.
The test added two new tests, one for speech-to-text tasks, based on the LibriSpeech data set developed in 2015 at Johns Hopkins University, using the widely deployed RNN-T neural network model; and one for what's called image segmentation, picking out the objects in a picture, based on the KiTS19 data set for tumor detection in CT scans, developed last year at the University of Minnesota, Carleton College, and the University of North Dakota.
The test suite also dropped two previous tests, GNMT and Transformer, replacing them with a similar natural language task, Google's BERT.
The new benchmark suite also added seven new submitters, and reported 650 individual results, versus the 138 reported last year.
Also: Nvidia and Google claim bragging rights in MLPerf benchmarks as AI computers get bigger and bigger
MLCommons chairman David Kanter said the benchmark suite is a "barometer for the whole industry," calling it "more exciting than Moore's Law," the historical measure of transistor improvement.
"You can see that since the start of MLPerf training, we've managed to boost performance, at the high side, by 27 times," said Kanter. Accuracy has also gone up at the same time, said Kanter.
"It's important to think about these not just as technical problems but as things that affect people's real lives," said Victor Bittorf, who serves as the chair of the ML Commons working group on ML training.
There appears to be a trend of companies and institutions using the MLPerf suite in their purchase decisions. Nvidia cited Taiwan Semiconductor Manufacturing, the world's biggest contract chip manufacturer, saying the tests are "an important factor in our decision making" when purchasing computers to run AI to use in chip making.
Although the tests are representative, there is an important divide between the benchmarks and the real-world implementations, according to Nvidia's Kharya, Real-world implementations use supercomputers, were the size of small models such as the benchmark ResNet-50 would be trivial.
Instead, those supercomputers are crunching neural networks with a trillion parameters or more, and still take days or weeks to train.
"Scale is really important," said Kharya. "We can train BERT in less than a minute on Selene," the supercomputer built with Intel's A100 GPUs and AMD EYPC microprocessors. "But it would take over two weeks to train a GPT-3 model, even on Selene," said Kharya, referring to the state-of-the-art language model from startup Open-AI.
Kharya's point was that Nvidia's performance gains will be amplified in the real world. A speed-up of three times in the performance of its GPUs on the small BERT model used in MLPerf, he said, will translate to a three-times speed-up in projects that take weeks to months to train.
That means Nvidia's progress can bring substantial reductions in the biggest training projects, he said.
"A difference of one minute versus one hour would look like a lot more in the real world," said Kharya.
The MLCommons in November debuted a separate set of benchmark tests for high-performance computing, or HPC, systems running the machine learning tasks.
Such grand scale is really where Graphcore and Cerebras and the rest are setting their sights.
"There's this whole space where very big companies are looking at very big language models," observed Graphcore's Fyles. "That will transition to other very large models where different types of models are combined, such as a mixture of experts."
For the startups, including Graphcore, the implicit hope is that such complex tasks will ultimately take them beyond merely competing on benchmarks with Nvidia, instead changing the paradigms in AI to their own advantage.
"If we just have a GPU model, if all we're doing is running GPU models, that's all we'll do, is compete with a GPU," said Fyles. "We are working with labs to get them to look at the IPU for a variety of uses, as more than simply in comparison to Nvidia."