Nvidia and Google claim bragging rights in MLPerf benchmarks as AI computers get bigger and bigger
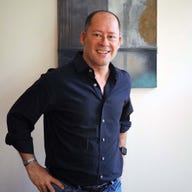
Nvidia and Google on Wednesday each announced that they had aced a series of tests called MLPerf to be the biggest and best in hardware and software to crunch common artificial intelligence tasks.
The devil's in the details, but both companies' achievements show the trend in AI continues to be that of bigger and bigger machine learning endeavors, backed by more-brawny computers.
Benchmark tests are never without controversy, and some upstart competitors of Nvidia and Google, notably Cerebras Systems and Graphcore, continued to avoid the benchmark competition.
In the results announced Wednesday by the MLPerf organization, an industry consortium that administers the tests, Nvidia took top marks across the board for a variety of machine learning "training" tasks, meaning the computing operations required to develop a machine learning neural network from scratch. The full roster of results can be seen in a spreadsheet form.
The second phase of machine learning, when those trained networks are used to make predictions in real time, known as inference, is covered in a separate competition that will be published later this year. Historically, the contest results have been unveiled once every quarter, but the COVID-19 pandemic has played havoc with the usual schedule. The current results were submitted by vendors in June and represent the first time this year the benchmark is being published.
A distinction has to be made with respect to Nvidia's top marks: they were for commercially available systems. Another category of submission is for systems that have the status of being research projects, meaning not available for use by customers. Google's cloud computing service's home-grown chip, the Tensor Processing Unit, or TPU, is one such research project. It blew past Nvidia's results on most tasks in the MLPerf test, if one considers both commercial and research achievements.
The results were the first time that Nvidia has reported metrics by which to assess the relative performance advantage of its newest chip, the A100, which was unveiled in May. Nvidia's senior director of product management, Paresh Kharya, in prepared remarks emphasized that the commercial availability of Nvidia's chip was significant, saying it showed the rapid move from chip debut to shipping systems.
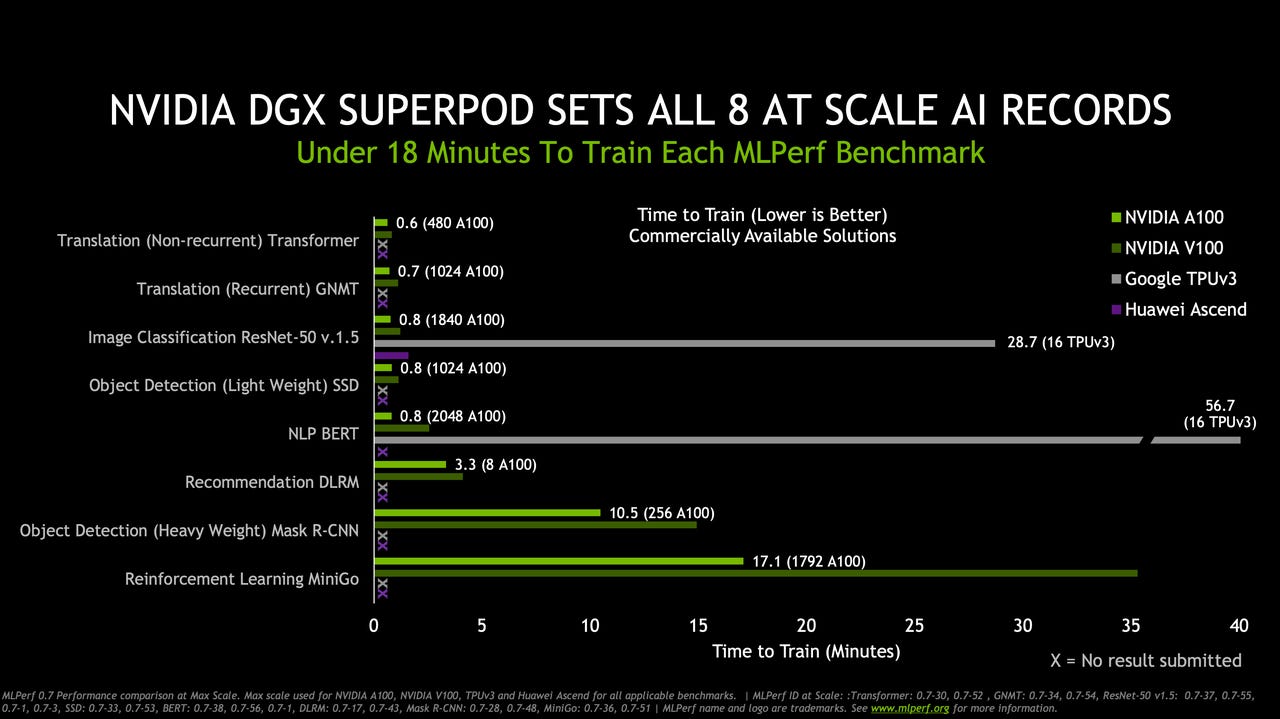
"Commercially available solutions have a very strict requirement that both the hardware and all the software and all the components need to be available to customers with evidence of third-party usage," said Kharya.
Kharya focused his presentation on comparing how systems built from numerous Nvidia processors were faster than production systems from Google using the TPUs.
For example, it took Nvidia about 49 seconds to train a version of the BERT natural language neural network using 2,048 A100 chips working in tandem, a computer Nvidia calls its SuperPOD. Less time is better in such tests. A Google machine that is commercially available in Google's cloud service, using 16 TPU chips, took almost 57 minutes for the same task, Nvidia pointed out.
However, one of Google's research projects, using 4,096 TPU chips, took the absolute top result to train BERT, only about 23 seconds. Even better performance is possible with the fourth version of Google's TPU, which is not yet in production with customers.
"There are several purposes to MLPerf, and one is to explore the absolute outer limits of performance," Zak Stone, Google's product manager for Cloud TPUs, told ZDNet.
"One of the ways we've done that here is by building the world's fastest training super-computer," referring to the 4,096-TPU system.
Although that system is a research project, some innovations that made possible the system are available to customers of Google's cloud service, he said. For example, an implementation of a popular object detection algorithm, Mask R-CNN, is going to be made available to all customers for use on present systems. That software helped Google cut the time to train object recognition from 35 minutes to just 8 minutes.
Counting its research projects, Google claimed top results in six out of eight tasks overall, Stone pointed out.
Other vendors competing in the benchmark test included Intel, whose Xeon processors were listed in a category called preview. Although the processors are expected to be commercially available, they show up under preview if they weren't shipping in time for the contest entry. Also competing were processors from Chinese telecom giant Huawei, the Ascend 910 chip, which were entered by the Shenzen Institute of Advanced Technology of the Chinese Academy of Sciences.
Nvidia clearly dominated the commercial category, with multiple vendors submitting performance results using the company's A100, including Dell EMC, Chinese search giant Alibaba, Fujitsu, China's Tencent, Chinese cloud service provider Inspur, and even Google itself.
Neither Cerebras, based in Los Altos in Silicon Valley, not far from Nvidia's Santa Clara headquarters, nor Graphcore, of Bristol, U.K., participated in the competition. Both have sat out the bake-off each time. In past, they have told ZDNet the structure of the competition is not reflective of real work their customers are interested in.
"We did not spend one minute working on MLPerf, we spent time working on real customers," Cerebras CEO Andrew Feldman told ZDNet last year. Cerebras last August unveiled the largest computer chip ever made, and followed it up with a dedicated computer system and dedicated software.
Graphcore, which has moved from chips to systems, has expressed similar reservations, saying that MLPerf has tended to be dominated by older AI tests, such as ResNet, making the tests less relevant.
Graphcore told ZDNet this week that it hopes to participate in future when MLPerf takes on a new form under the direction of a steering group known as the ML Commons, a 501C6 industry non-profit.
In response to the critiques from Cerebras and Graphcore, David Kanter, head of MLPerf, remarked to ZDNet that there are bound to be differences of opinion over what should be tested. The benchmark is meant to strike a balance.
"Many of these benchmarks are constructed to be as representative as possible, but being representative also means that it's not an exact match," said Kanter. "If you want it to be general, it's probably not going to be exactly what you do or I do," he said, referring to different workloads.
"MLPerf overall does a really good job of driving improvements across all platforms, across a range of workloads that track real workloads that are representative of industry and academia," said Google's Stone.
The benchmark suite continues to evolve, MLPerf's Kanter noted. The results announced today were the first time the BERT neural network for natural language was included as a test. Another test that was added is a recommendations engine for online commerce called DLRM, which stands for Deep Learning Recommendation Model. An existing test, based on reinforcement learning, called Mini-Go, was expanded to be more challenging. It now tests performance on a full-size, 19 by 19-square board of the ancient strategy game Go.
An important new step this time around, said Kanter, was that MLPerf drew upon the advice of an advisory board from industry and from academia to chose to add DLRM to the tests.
"We had people participating from all over, giving us the advice that this is approximately what you want, while other stuff is too far out, people don't use it in production," he said.
Google's Stone lauded the inclusion of DLRM for the first time. "I think it's representative of where the core algorithms to many online businesses are going."
Some of the contention over what MLPerf should be pertains to the complexity of the benchmark. It is not just a measure of chips, but a measure of how many elements come together to form a computer system.
"MLPerf has a very significant software component," Kanter told ZDNet.
"A typical MLPerf solution will have a compiler, it will have a math library, it will have a linear algebra library, you may have a library for clustering, your accelerator driver, a framework — and that's not an exhaustive list!"
MLPerf is finding the real relevant things to measure along all those avenues of innovation, said Kanter.
"We're all realists and we understand that people will really optimize benchmarks as a point of pride and as a point of marketing, but we want those optimizations to be valuable to the broader world," he said.
One interesting area left out of the measurements are the cost and energy factors of different chips and computers. Is a machine, for example, that accomplishes a task with 1,024 chips necessarily more costly, or more power-hungry, than a system with half as many chips?
But MLPerf is designed to record raw performance, it says nothing about the energy consumed by any vendor's system, nor the cost of the product. So economics and environmental advantage can't be compared.
"Energy efficiency is a very important consideration for customers," Nvidia's Kharya told ZDNet. "We look forward to working with MLPerf to incorporate some of these metrics into the benchmarks going forward."
"I think it would be fantastic to have authoritative comparisons of cost to the user as we do in performance," said Google's Stone. "I don't want to speak on behalf of the community; the community process needs to sort through the appropriate guidelines."
Measuring energy efficiency, such as cycles of instructions per watt of energy consumed, is complicated by the very scale of different systems entered into the competition, Kanter told ZDNet. Comparing the economics of different systems is even more challenging, he said.
"The way that things are priced in cloud and on premise is very different," he observed. "That really presents a challenge" to comparing effective cost.
A definite trend across vendors is that bigger and bigger hardware will be the norm for the foreseeable future. Things that once took days, weeks, years, can be done in the blink of an eye. It took just 28 seconds for Google's machine with 4,096 TPUs to train the venerable ResNet neural network to classify images on the common ImageNet task. That's down from 77 seconds a year ago for the best result.
More to the point, much bigger machines can handle much bigger, more ambitious machine learning software models, things like OpenAI's GPT-3 language model, which comes closer than ever to forming realistic natural language phrases.
"I think we can't ignore the huge trend in the research community toward very large models," said Google's Stone.
"I am not claiming that blind increases in scale are all that's necessary," he added. "But the broader capability of larger and larger-scale supercomputers is the prerequisite for these brilliant teams of researchers to even explore hypotheses; it's the new baseline."
"It is certainly a dimension you can push along," said MLPerf's Kanter of building bigger systems.
"From a pure technical tour de force, it's incredibly headline-grabbing, but you have to look at the entire universe of machine learning systems," he said. "Some users will say, I've got four accelerators by my desk and that's what I can afford."
"We would like to be of equal service to both those corners of the landscape."