Has AI passed a new milestone? It's beaten human players at poker, say researchers

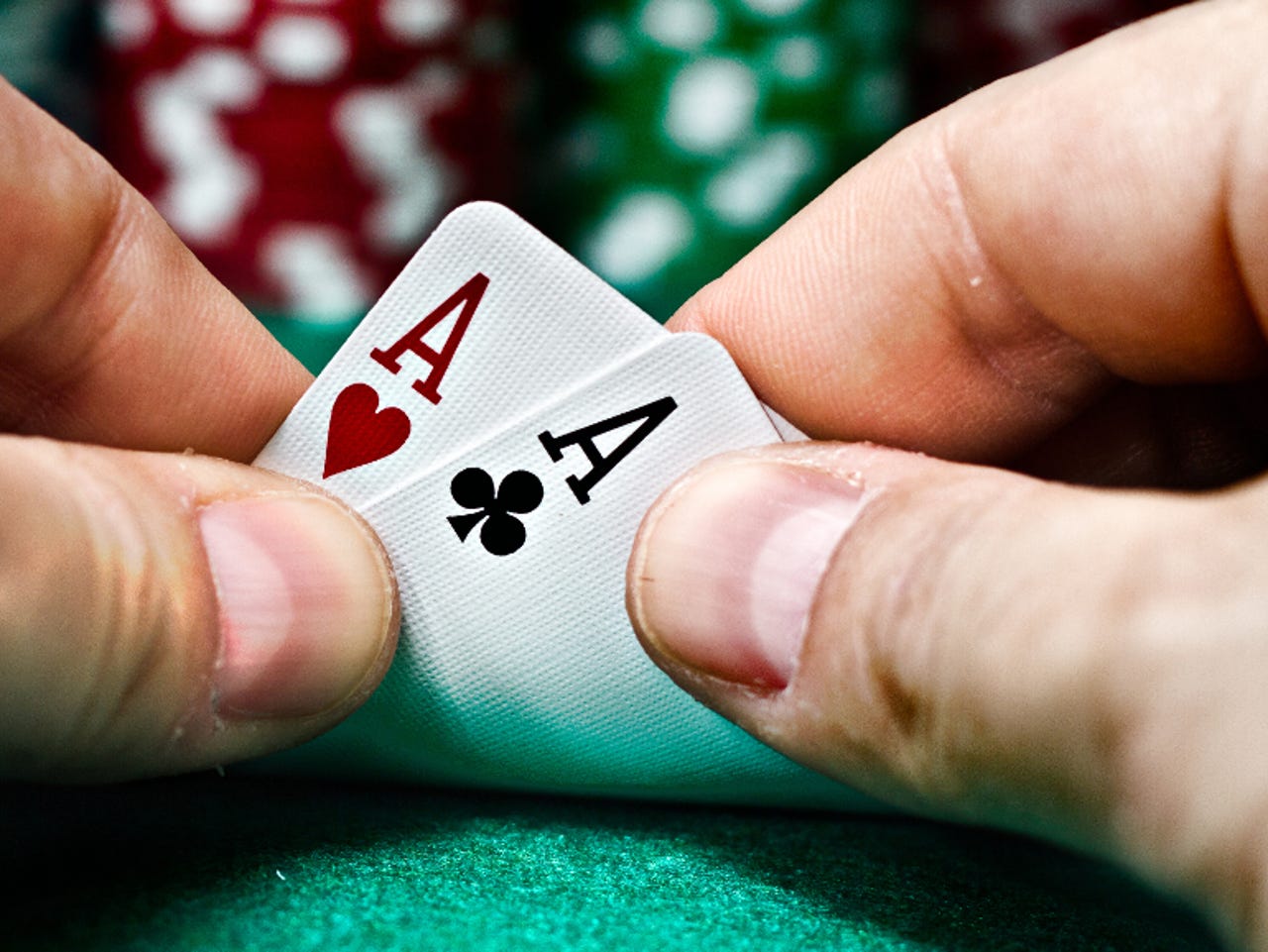
Beating expert poker players differs from past AI successes against human competitors in games such as Jeopardy and Go.
Researchers behind a poker-playing AI system called DeepStack say it's the first algorithm to have ever beaten poker pros in a game of heads-up no-limit Texas hold 'em.
The claim, if verified, would mark a major milestone in the development of artificial-intelligence systems.
Beating expert poker players differs from past AI successes against human competitors in games such as Jeopardy and Go because in poker each player's hand provides only an incomplete picture about the state of play and requires a program to navigate tactics, such as bluffing, based on asymmetrical information.
DeepStack is the work of a collaboration between researchers at the University of Alberta and two Czech universities, who say in a new non-peer reviewed paper that it's the "first computer program to beat professional poker players in heads-up no-limit Texas hold 'em".
The new paper arrived as a rival AI poker team of researcher from Carnegie Mellon University announced a $200,000 match between its system, Libratus, and four poker pros: Jason Les, Dong Kim, Daniel McAulay, and Jimmy Chou. Collectively, the four human pros will play 120,000 hands of heads-up no-limit Texas hold 'em over 20 days against Libratus.
Libratus is Carnegie Mellon University's successor to a program called Claudico, which squared up and lost against the same four players in 2015 over 80,000 hands. Microsoft Research donated the $100,000 prize pool for that competition.
While both Claudico and DeepStack use a technique called "counterfactual regret minimization" to reason through card-play strategy, DeepStack's makers say its system "takes a fundamentally different approach" in how it handles information asymmetry, including simulating a "gut feeling" for which cards to hold on to.
Both Libratus and DeepStack are described as using novel approaches for achieving a Nash equilibrium, which Carnegie Mellon University defines as a "pair of strategies, one per player, where neither player can benefit from changing strategy as long as the other player's strategy remains the same".
"The DeepStack algorithm seeks to compute and play a low-exploitability strategy for the game, ie, solve for an approximate Nash equilibrium. DeepStack computes this strategy during play and only for the states of the public tree that actually arise during play. This local computation lets DeepStack reason in games that are too big for existing algorithms without having to abstract the game's 10 to the power of 160 decision points down to 10 to the power of 14 to make solving tractable," DeepStack's researchers wrote.
DeepStack was evaluated against 33 professional poker players from the International Federation of Poker. Each participant was asked to play a 3,000-game match over a month.
"In total 44,852 games were played by the 33 players with 11 players completing the requested 3,000 games. Over all games played, DeepStack won 492 mbb/g [milli-big-blinds per game]. This is over four standard deviations away from zero, and so highly significant," the DeepStack researchers wrote.
Carnegie Mellon University says Libratus employs a faster method to find a Nash equilibrium as well as developing better endgame strategies, which are powered by the Pittsburgh Supercomputing Center's Bridges supercomputer.
"We're pushing on the supercomputer like crazy," said Sandholm, who said Libratus was build with 15 million core hours of computation compared with the three million used for Claudico.
Carnegie Mellon University's match kicks off today at 11am at the Pittsburgh Rivers Casino and will end around 7pm.