How machines are beating cardiologists in North Central Pennsylvania
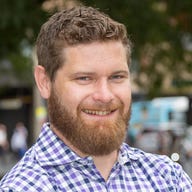
As the world rushes headlong down a path where machine learning (ML) is going to insert itself into myriad tasks, medicine is primed to be one of the early success stories where humans augmented by machines will literally save lives.
In North Central Pennsylvania, integrated health network Geisinger has trained neural networks to examine echocardiograms, and the machines are outperforming its cardiologists. But when it comes to improving the overall medical field, which includes teaching doctors about what the machine has picked up, it all remains a 'black box'.
Speaking at Nvidia GTC in March, Dr Brandon Fornwalt, associate professor and director of Geisinger Department of Imaging Science & Innovation, said that's something the company is trying to look into.
"We haven't been able to figure out [what the model is seeing that the cardiologists cannot]; we're trying different things," he said. "It's very hard to figure that out."
Electronic health records enable machine learning
Geisinger is a health network of 13 hospitals spanning the northern part of Pennsylvania and into New Jersey. It has a wealth of data thanks to an electronic health record (EHR) system introduced in 1996. This contains 1.9 million patients, almost a billion outpatient vital sign measurements, 3.6 billion rows in the dataset, 140,000 whole exomes that have been sequenced, and demographics information indicating that the population is incredibly stable.
"They are born there, they live there, they work there, they grow up, they have kids there -- and so we have an average of 16 years of longitudinal follow on our patients, which allows us to go back in time and grab a snapshot of the patients and then predict the future -- and the future has already happened because we captured that in the retrospective data," Fornwalt said. "So it's a very unique application for predictive modelling and machine learning," he added.
The health provider has also had a team of data analysts and modellers for a over a decade, with the clinical image archive possessing 11 million clinical studies taking up around 2 petabyes of data -- almost 200,000 of the studies have been used in research or innovation pushes, Fornwalt explained.
One area where deep learning has been applied is in intracranial haemorrhaging, where a neural network is used on images from a CAT scanner. When the network detects an abnormality in the patient, the corresponding image is brought to the top of the queue for the radiologist to look at -- the idea is that radiologists can read the most acute findings first.
With over 46,000 head CAT scans consisting of 2 million images collected over a decade, the neural network is trained on data classified as containing or not containing an intracranial haemorrhage.
The system has been operating within Geisinger for two years and during that period the time to diagnosis of outpatient haemorrhages has reduced by 96 percent. For 10 percent of cases where a radiologist had given the all-clear while the machine determined a haemorrhage was present, a second opinion with another human had found a "subtle haemorrhage that may or may not have been clinically significant".
Machine bests humans on cardiac imaging
Another project with neural networks that Geisinger has undertaken is attempting to improve mortality prediction and risk scores.
"Risk is everything in medicine: we give diagnosis because we think it tells us about risk, and we also give treatments because we think we can mitigate risk with treatments," Fornwalt said. "That's really what medicine is about: predicting future events, the risk scores, and risk stratification."
The team used 300,000 echocardiograms from 170,00 patients for the neural network, and it performed much, much better than current clinical metrics. While the result itself was not unexpected, how the network derived the results was.
Fornwalt said that after age, the variable that most affected the result returned by the machine learning network was the TR max velocity variable that measures pulmonary pressure.
"Injection faction is the way that we really look at cardiac function data and the one variable that we use the most -- and yet this other variable that's sort of buried in the report that we never pay attention to came up to the top of the list above all of the [other] metrics," he said.
"This is a way of uncovering the black box and making physicians more comfortable and understanding what these models are doing to predict the future."
Typically during an echocardiogram appointment, the service provider receives 30 videos to make a diagnosis on, Fornwalt explained; using just one video, a neural network can perform better than the current clinical metrics.
"I didn't believe it because that's beating the clinical risk scores ... and that's one of greater than 20 videos and clinical data that we've not yet added to this model -- it's just one video," he said.
The Geisinger team then ran the neural network over its catalogue of 720,000 videos -- which took two weeks on an Nvidia DGX-1 -- and found the accuracy of the model approached 80 percent, while the best human was only hitting 60 percent.
"The cardiologist tended to say that the patient would live ... but that was at the expense of sensitivity -- that is, saying that patients were going to die," Fornwalt said.
"I'm not saying that the machine is better than a cardiologist at what they do, but what I'm saying here is that this is evidence to suggest that machines are going to be able to add predictive value that humans are probably not going to be able to do."
With electrocardiograms (ECGs), Geisinger trained a neural network to predict one-year mortality on 1.8 million ECGs it had collected over 38 years from around 400,000 patients that were linked to outcomes such as death and clinical events -- and once again, accuracy was around the 80 percent mark. But, as Fornwalt described, the interesting result that appeared when the network ran over 300,000 ECGs that were deemed as normal by clinicians, was the network returned the same sort of one-year survival trend as the wider dataset.
"I was kind of shocked at this, because that means that the cardiologist has essentially said 'Hey this is completely normal' but the neural network is finding features in there that are predictive of one-year survival, so how can it be truly normal?," he said.
Going back to the cardiologists, the team returned to see if they could train them to see what the machine was seeing. The cardiologists were shown a pair of ECGs as well the parameters associated with it including age and sex, and told that one of them was predicted by the machine to live, and the other to die. This activity was completed around 400 times, and the result was little better than 50/50 random chance.
So the cardiologists were given another dataset to practice on, and the same test again.
"It didn't change," Fornwalt said. "So we can't even teach them how to see the features that the neural network is picking up on."
"We thought that was a pretty powerful result to say: 'The neural network is doing things that we can't see as humans'."
Disclosure: Chris Duckett travelled to GTC in San Jose as a guest of Nvidia
Related coverage
UPS, Matternet launch drone healthcare delivery service
A drone can make a trip from a clinic to a lab in 3 minutes and 15 seconds, while a car can take up to 30 minutes.
AI vs heart disease: How machine learning could help doctors tackle heart problems before they happen
A system that spun out of Stanford is using AI and machine learning to help doctors visualise patients' arteries and spare them invasive tests.
How machine learning, drones, and robotics will transform the NHS and healthcare
For a health service in the middle of funding squeeze, can new technologies help to improve care and cut costs?
How hospitals can use AI to fight medical device hacking (TechRepublic)
Ben-Gurion University researchers developed a device-focused cybersecurity solution to act as a last line of defense to protect patients.
How a companion robot can help children with chronic illness (TechRepublic)
The companion robot, ikki, is portable and helps children to monitor their health and feel more engaged in the recovery process.
The role of data science and technology in mental health treatment (TechRepublic)
Valera Health uses technology to triage patients to determine which level of care is necessary for patients requiring behavioral and mental health treatment.