Is LiDAR on its way out? The business case for saying goodbye
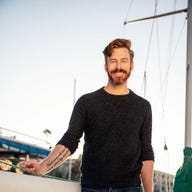

Among the deluge of robotics predictions you're bound to encounter this year, there's one you should pay particular attention to: The way robots "see" is fundamentally changing, and that's going to have a huge impact on the utility cost and proliferation of robotic systems.
Of course, it's a bit of a mischaracterization to talk about robots "seeing," or at least a reductive shorthand for a complex interplay of software and hardware that's allowing robots to do much more sophisticated sensing with much less costly equipment. Machine vision incorporates a variety of technologies and increasingly relies on software in the form of machine learning and AI to interpret and process data from 2D sensors that would have been unachievable even a short time ago.
With this increasing reliance on software comes an interesting shift away from highly specialized sensors like LiDAR, long a staple for robots operating in semi-structured and unstructured environments. Robotics experts marrying the relationship between humans and AI software are coming to find that LiDAR isn't actually necessary. Rather, machine vision is providing higher quality mapping\at a more affordable cost, especially when it comes to indoor robotics and automation.
See also: 2022: A major revolution in robotics.
To learn more about the transformation underway, I connected with Rand Voorhies, CTO & co-founder at inVia Robotics, about machine vision, the future of automation, and whether LiDAR is still going to be a foundational sensor for robots in the years ahead.
GN: Where have the advances come in machine vision, the sensors or the software?
Rand Voorhies: While 2D imaging sensors have indeed seen constant continuous improvement, their resolution/noise/quality has rarely been a limiting factor to the widespread adoption of machine vision. While there have been several interesting sensor improvements in the past decade (such as polarization sensor arrays and plenoptic/light-field cameras), none have really gained traction, as the main strengths of machine vision sensors are their cost and ubiquity. The most groundbreaking advancement has really been along the software front through the advent of deep learning. Modern deep learning machine vision models seem like magic compared to the technology from ten years ago. Any teenager with a GPU can now download and run object recognition libraries that would have blown the top research labs out of the water ten years ago. The fact of the matter is that 2D imaging sensors capture significantly more data than a typical LiDAR sensor - you just have to know how to use it.
While cutting-edge machine vision has been improving in leaps and bounds, other factors have also contributed to the adoption of even simpler machine vision techniques. The continual evolution of battery and motor technology has driven component costs down to the point where robotic systems can be produced that provide a very strong ROI to the end-user. Given a good ROI, customers (in our case, warehouse operators) are happy to annotate their environment with "fiducial" stickers. These stickers are almost like a cheat-code to robotics, as very inexpensive machine vision solutions can detect the position and orientation of a fiducial sticker with ultra-precision. By sticking these fiducials all over a warehouse, robots can easily build a map that allows them to localize themselves.
GN: Can you give a little context on LiDAR adoption? Why has it become such a standardized sensing tool in autonomous mobility applications? What were the early hurdles to machine vision that led developers to LiDAR?
Rand Voorhies: Machine vision has been used to guide robots since before LiDAR existed. LiDAR started gaining significant popularity in the early 2000s due to some groundbreaking academic research from Sebastian Thrun, Daphne Koller, Michael Montemerlo, Ben Wegbreit, and others that made processing data from these sensors feasible. That research and experience led to the dominance of the LiDAR-based Stanley autonomous vehicle in the DARPA Grand Challenge (led by Thrun), as well as to the founding of Velodyne (by David Hall, another Grand Challenge participant), which produces what many now consider to be the de-facto autonomous car sensor. The Challenge showed that LiDAR was finally a viable technology for fast-moving robots to navigate through unknown, cluttered environments at high speeds. Since then, there has been a huge increase in academic interest in improving algorithms for processing LiDAR sensor data, and there have been hundreds of papers published and PhDs minted on the topic. As a result, graduates have been pouring into the commercial space with heaps of academic LiDAR experience under their belt, ready to put theory to practice.
In many cases, LiDAR has proven to be very much the right tool for the job. A dense 3D point cloud has long been the dream of roboticists and can make obstacle avoidance and pathfinding significantly easier, particularly in unknown dynamic environments. However, in some contexts, LiDAR is simply not the right tool for the job and can add unneeded complexity and expense to an otherwise simple solution. Determining when LiDAR is right and when it's not is key to building robotic solutions that don't just work -- they also provide positive ROI to the customer.
At the same time, machine vision has advanced as well. One of the early hurdles in machine vision can be understood with a simple question: "Am I looking at a large object that's far away or a tiny object that's up-close"? With traditional 2D vision, there was simply no way to differentiate. Even our brains can be fooled, as seen in funhouse perspective illusions. Modern approaches to machine vision use a wide range of approaches to overcome this, including:
- Estimating the distance of an object by understanding the larger context of the scene, e.g., I know my camera is 2m off the ground, and I understand that car's tires are 1000 pixels along the street, so it must be 25m away.
- Building a 3D understanding of the scene by using two or more overlapping cameras (i.e., stereo vision).
- Building a 3D understanding of the scene by "feeling" how the camera has moved, e.g., with an IMU (inertial measurement unit - sort of like a robot's inner ear) and correlating those movements with the changing images from the camera.
Our own brains use all three of these techniques in concert to give us a rich understanding of the world around us that goes beyond simply building a 3D model.
GN: Why is there a better technological case for machine vision over LiDAR for many robotics applications?
Rand Voorhies: LiDAR is well suited for outdoor applications where there are a lot of unknowns and inconsistencies in terrain. That's why it's the best technology for self-driving cars. In indoor environments, machine vision makes the better technological case. As light photons are bouncing off objects within a warehouse, robots can easily get confused under the direction of LiDAR. They have a difficult time differentiating, for example, a box of inventory from a rack of inventory -- both are just objects to them. When the robots are deep in the aisles of large warehouses, they often get lost because they can't differentiate their landmarks. Then they have to be re-mapped.
By using machine vision combined with fiducial markers, our inVia Picker robots know exactly where they are at any point in time. They can "see" and differentiate their landmarks. Nearly all LiDAR-based warehouse/industrial robots require some fiducial markers to operate. Machine vision-based robots require more markers. The latter requires additional time and cost to deploy long rolls of stickers vs fewer individual stickers, but when you factor in the time and cost to perform regular LiDAR mapping, the balance swings far in the favor of pure vision. At the end of the day, 2D machine vision in warehouse settings is cheaper, easier, and more reliable than LiDAR.
If your use of robots does not require very high precision and reliability, then LiDAR may be sufficient. However, for systems that cannot afford any loss in accuracy or uptime, machine vision systems can really show their strengths. Fiducial-based machine vision systems allow operators to put markers exactly where precision is required. With inVia's system that is picking and placing totes off of racking, placing those markers on the totes and the racking provides millimeter level accuracy to ensure that every tote is placed exactly where it's supposed to go without fail. Trying to achieve this with a pure LiDAR system would be cost and time prohibitive for commercial use.
GN: Why is there a better business case?
Rand Voorhies: On the business side, the case is simple as well. Machine vision saves money and time. While LiDAR technology has decreased in cost over the years, it's still expensive. We're committed to finding the most cost-effective technologies and components for our robots in order to make automation accessible to businesses of any size. At inVia we're driven by an ethos of making complex technology simple.
The difference in the time it takes to fulfill orders with machine vision versus with LiDAR and all of its re-mapping requirements is critical. It can mean the difference in getting an order to a customer on time or a day late. Every robot that gets lost due to LiDAR re-mapping reduces that system's ROI.
The hardware itself is also cheaper when using machine vision. Cameras are cheaper than LiDAR, and most LiDAR systems need cameras with fiducials anyway. With machine vision, there's an additional one-time labor cost to apply fiducials. However, applying fiducials one time to totes/racking is extremely cheap labour-wise and results in a more robust system with less downtime and errors.
GN: How will machine vision change the landscape with regards to robotics adoption in sectors such as logistics and fulfillment?
Rand Voorhies: Machine vision is already making an impact in logistics and fulfillment centers by automating rote tasks to increase the productivity of labor. Warehouses that use robots to fulfill orders can supplement a scarce workforce and let their people manage the higher-order tasks that involve decision-making and problem-solving. Machine vision enables fleets of mobile robots to navigate the warehouse, performing key tasks like picking, replenishing, inventory moves, and inventory management. They do this without disruption and with machine-precision accuracy.
Using robotics systems driven by machine vision is also removing barriers to adoption because of their affordability. Small and medium-sized businesses that used to be priced out of the market for traditional automation are able to reap the same benefits of automating repetitive tasks and, therefore, grow their businesses.
GN: How should warehouses go about surveying the landscape of robotics technologies as they look to adopt new systems?
Rand Voorhies: There are a lot of robotic solutions on the market now, and each of them uses very advanced technology to solve a specific problem warehouse operators are facing. So, the most important step is to identify your biggest challenge and find the solution that solves it.
For example, at inVia we have created a solution that specifically tackles a problem that is unique to e-commerce fulfillment. Fulfilling e-commerce orders requires random access to a high number of different SKUs in individual counts. That's very different from retail fulfillment, where you're retrieving bulk quantities of SKUs and shipping them out in cases and/ or pallets. The two operations require very different storage and retrieval setups and plans. We've created proprietary algorithms that specifically create faster paths and processes to retrieve randomly accessed SKUs.
E-commerce is also much more labor-dependent and time-consuming, and, therefore, costly. So, those warehouses want to adopt robotics technologies that can help them reduce the cost of their labor, as well as the time it takes to get orders out the door to customers. They have SLAs (service level agreements) that dictate when orders need to be picked, packed, and shipped. They need to ask vendors how their technology can help them eliminate blocks to meet those SLAs.