McKinsey: AI, jobs, and workforce automation
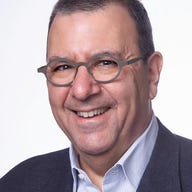
For business people, AI presents a variety of challenges. On a technology level, artificial intelligence and machine learning is complicated to develop and demands rich data sets to produce meaningful results. From a business perspective, many business leaders have difficulty figuring out where to apply AI and even how to start the machine intelligence journey.
Making matters worse, the constant drumbeat of AI hype from every technology vendor has created a continual barrage of noise confuses the market about the real possibilities of AI.
To cut through this noise, I have invited many world-leading practitioners to share their expertise as part of the CXOTALK series of conversations with innovators.
For episode 219 of CXOTALK, I spoke with Michael Chui, a Principal at the McKinsey Global Institute (MGI), and David Bray, an Eisenhower Fellow who is also CIO at the Federal Communications Commission.
The McKinsey Global Institute has released a variety of research reports on topics related to Ai, automation, and jobs. For example, see this article on the fundamentals of workplace automation.
As you can see in the graphic below, Chui and his team examined a variety of industries looking at the impact of automation, including AI, on the workforce.
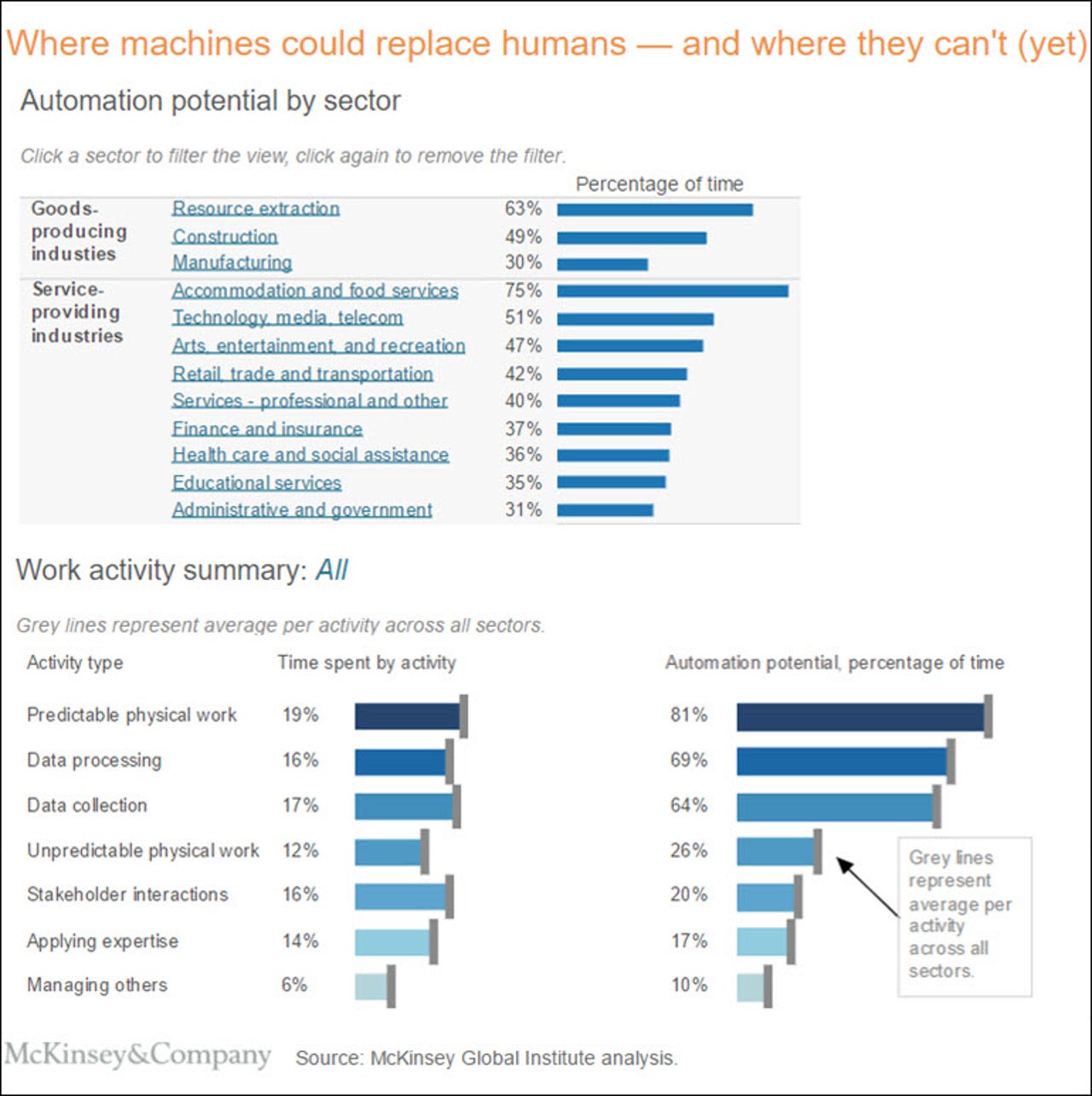
Image from McKinsey Global Institute
Another fascinating graphic showing automation potential and wages for US jobs:
Image from McKinsey Global Institute
The conversation between Michael Chui and David Bray covered key points about the relationship of business and the workforce to automation and AI - including investment, planning, and even ethical considerations.
You can watch our entire conversation in the video embedded above. An edited partial transcript is available below and you can read the complete transcript at the CXOTALK site.
How should organizations think about investing in AI?
Michael Chiu: More organizations have started to understand the potential of data analytics. Executives are starting to understand that data and analytics are either becoming a basis of competition or a basis for offering the services and products that your customers, citizens, and stakeholders need.
While there are often real technology challenges, we often find the real barrier is the people stuff. How do you get from an interesting experiment to business-relevant insight? We could increase the conversion rate by X percentage if we used this next product to buy an algorithm and this data; we could reduce the maintenance costs, or increase the uptime of this whole good. We could, in fact, bring more people into this public service because we can find them better.
Getting from that insight to capture value at scale is where organizations are either stuck or falling. How do you bag that interesting insight, that thing that you capture, whether in it's in the form of a machine learning algorithm, or other types of analytics, into the practices and processes of an organization, so it changes the way things operate at scale? To use a military metaphor: How do you steer that aircraft carrier? It's as true for freight ships as it is for military ships. They are hard things to turn.
It's the organizational challenge of understanding the mindsets, having the right talent in place, and then changing the practices at scale. That's where we see a big difference between organizations who have just reached awareness and maybe done something interesting and ones who have radically changed their performance in a positive way through data, analytics, and AI.
What are the adoption problems around AI and machine learning?
David Bray: The real secret to success is changing what people do in an organization, that you can't just roll out technology and say, "We've gone digital, but we didn't change any of our business processes," and expect to have any great outcomes. I have seen experiments that are isolated from the rest of public service; and they say, "Well look, we're doing these experiments over here!" but they're never translating to changing how you do the business of public service at scale.
Doing that requires not just technology, but understanding the narrative of how the current processes work, why they're being done that way in an organization, and then what is the to-be state, and how are you going to be that leader that shepherds the change from the as-is to the to-be state? For public service, we probably lack conversations right now about how to deliver results differently and dramatically better to the public.
Artificial intelligence, in some respects, is just a continuation of predictive analytics, a continuation of big data, it is nothing new because technology always changes the art of the possible; this is just a new art of the possible.
I do think there's an interesting thing in which it could offer a reflection of our biases through artificial intelligence. If we're not careful, we'll roll out artificial intelligence, populating it with data from humans, [and] we know humans have biases, and we'll find out that the artificial intelligence itself, the machine learning itself, is biased. I think that's a little bit more unique than just a predictive analytics bias or big data.
Which business areas most suited to AI?
Michael Chiu: When we surveyed about 600 different industry experts, every single one of those problems we identified, at least one expert suggested it was one of the top three problems that machine learning could help improve. And so, what that says is potential is just absolutely huge. There's almost no problem where AI and machine learning potentially couldn't change and improve performance.
A few things that come to mind: One is a lot of the most interesting and recent research has been in this field called "deep learning," and that's particularly suited for certain types of problems with pattern recognition, often images, etc. And so those problems that are like image recognition, pattern recognition, etc. are some of those that are quite amenable and interesting.
So again, regarding very specific types of problems, predictive maintenance is huge. The ability to keep something from breaking; rather than waiting until it breaks and then fixing it, the ability to predict when something's going to break. Not only because it reduces the cost. More important, is the thing doesn't go down. If you bring down a part of an assembly line, you bring down the entire factory or often the entire line.
To a certain extent, that is an example of pattern matching. Sensors are the signals that reflect that something's going to break, informing you to do predictive maintenance. We find that across a huge number of specific industries that have these capital assets, whether it's a generator, a building, an HDC system, or a vehicle, where if you're able to predict ahead of time before something's going to break, you should conduct some maintenance. That is one of the areas in which machine learning can be quite powerful.
Health care is another case of predictive maintenance but on the human capital asset. Then you can start to think, "Well gosh! I have the internet of things." I have sensors on a patient's body. Can I tell before they're going to have a cardiac incident? Can I tell before someone's going to have a diabetic incident? That they should take some actions which could be less expensive, and less invasive, than having it turn into an emergent case where they must go through a very expensive, painful, and urgent care type of situation?
Again, can you use machine learning make predictions? Those are some of the problems things that can potentially be solved better by using AI and machine learning.
David Bray: There are opportunities for artificial intelligence and machine learning to help the public. I think a lot is going to happen first in cities.
We've heard about smart cities. You can easily see better preventive maintenance on roads or power generation and then monitoring to avoid brownouts. I think the real practical, initial, early adoption of AI and machine learning is going to happen first at the city level. Then we've got to figure out how to best use it at the federal level.
CXOTALK brings together the most innovative leaders in the world for in-depth conversations about leadership and innovation. See the complete list of episodes.