Meta's AI guru LeCun: Most of today's AI approaches will never lead to true intelligence
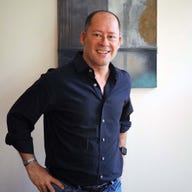

"I think AI systems need to be able to reason," says Yann LeCun, Meta's chief AI scientist. Today's popular AI approaches such as Transformers, many of which build upon his own pioneering work in the field, will not be sufficient. "You have to take a step back and say, Okay, we built this ladder, but we want to go to the moon, and there's no way this ladder is going to get us there," says LeCun.
(Article is updated with rebuttals by Gary Marcus and Jürgen Schmidhuber in context.)
Yann LeCun, chief AI scientist of Meta, owner of Facebook, Instagram, and WhatsApp, is likely to tick off a lot of people in his field.
With the posting in June of a think piece on the Open Review server, LeCun offered a broad overview of an approach he thinks holds promise for achieving human-level intelligence in machines.
Implied if not articulated in the paper is the contention that most of today's big projects in AI will never be able to reach that human-level goal.
In a discussion this month with ZDNET via Zoom, LeCun made clear that he views with great skepticism many of the most successful avenues of research in deep learning at the moment.
"I think they're necessary but not sufficient," the Turing Award winner told ZDNET of his peers' pursuits.
Those include large language models such as the Transformer-based GPT-3 and their ilk. As LeCun characterizes it, the Transformer devotées believe, "We tokenize everything, and train giganticmodels to make discrete predictions, and somehow AI will emerge out of this."
"They're not wrong," he says, "in the sense that that may be a component of a future intelligent system, but I think it's missing essential pieces."
Also: Meta's AI luminary LeCun explores deep learning's energy frontier
It's a startling critique of what appears to work coming from the scholar who perfected the use of convolutional neural networks, a practical technique that has been incredibly productive in deep learning programs.
LeCun sees flaws and limitations in plenty of other highly successful areas of the discipline.
Reinforcement learning will also never be enough, he maintains. Researchers such as David Silver of DeepMind, who developed the AlphaZero program that mastered Chess, Shogi and Go, are focusing on programs that are "very action-based," observes LeCun, but "most of the learning we do, we don't do it by actually taking actions, we do it by observing."
Lecun, 62, from a perspective of decades of achievement, nevertheless expresses an urgency to confront what he thinks are the blind alleys toward which many may be rushing, and to try to coax his field in the direction he thinks things should go.
"We see a lot of claims as to what should we do to push forward towards human-level AI," he says. "And there are ideas which I think are misdirected."
"We're not to the point where our intelligent machines have as much common sense as a cat," observes Lecun. "So, why don't we start there?"
He has abandoned his prior faith in using generative networks in things such as predicting the next frame in a video. "It has been a complete failure," he says.
LeCun decries those he calls the "religious probabilists," who "think probability theory is the only framework that you can use to explain machine learning."
The purely statistical approach is intractable, he says. "It's too much to ask for a world model to be completely probabilistic; we don't know how to do it."
Not just the academics, but industrial AI needs a deep re-think, argues LeCun. The self-driving car crowd, startups such as Wayve, have been "a little too optimistic," he says, by thinking they could "throw data at" large neural networks "and you can learn pretty much anything."
"You know, I think it's entirely possible that we'll have level-five autonomous cars without common sense," he says, referring to the "ADAS," advanced driver assistance system terms for self-driving, "but you're going to have to engineer the hell out of it."
Such over-engineered self-driving tech will be something as creaky and brittle as all the computer vision programs that were made obsolete by deep learning, he believes.
"Ultimately, there's going to be a more satisfying and possibly better solution that involves systems that do a better job of understanding the way the world works."
Along the way, LeCun offers some withering views of his biggest critics, such as NYU professor Gary Marcus — "he has never contributed anything to AI" — and Jürgen Schmidhuber, co-director of the Dalle Molle Institute for Artificial Intelligence Research — "it's very easy to do flag-planting."
Beyond the critiques, the more important point made by LeCun is that certain fundamental problems confront all of AI, in particular, how to measure information.
"You have to take a step back and say, Okay, we built this ladder, but we want to go to the moon, and there's no way this ladder is going to get us there," says LeCun of his desire to prompt a rethinking of basic concepts. "Basically, what I'm writing here is, we need to build rockets, I can't give you the details of how we build rockets, but here are the basic principles."
The paper, and LeCun's thoughts in the interview, can be better understood by reading LeCun's interview earlier this year with ZDNET in which he argues for energy-based self-supervised learning as a path forward for deep learning. Those reflections give a sense of the core approach to what he hopes to build as an alternative to the things he claims will not make it to the finish line.
Robotics
What follows is a lightly edited transcript of the interview.
ZDNET: The subject of our chat is this paper, "A path toward autonomous machine intelligence," of which version 0.9.2 is the extant version, yes?
Yann LeCun: Yeah, I consider this, sort-of, a working document. So, I posted it on Open Review, waiting for people to make comments and suggestions, perhaps additional references, and then I'll produce a revised version.
ZDNET: I see that Juergen Schmidhuber already added some comments to Open Review.
YL: Well, yeah, he always does. I cite one of his papers there in my paper. I think the arguments that he made on social networks that he basically invented all of this in 1991, as he's done in other cases, is just not the case. I mean, it's very easy to doflag-planting, and to, kind-of, write an idea without any experiments, without any theory, just suggest that you could do it this way. But, you know, there's a big difference between just having the idea, and then getting it to work on a toy problem, and then getting it to work on a real problem, and then doing a theory that shows why it works, and then deploying it. There's a whole chain, and his idea of scientific credit is that it's the very first person who just, sort-of, you know, had the idea of that, that should get all the credit. And that's ridiculous.
(Update: Jürgen Schmidhuber replies, "LeCun claims that my 'idea of scientific credit is that it's the very first person who just, sort-of, you know, had the idea of that, that should get all the credit.' In no universe is this true. As I wrote [DLC]: 'the inventor of an important method should get credit for inventing it. She may not always be the one who popularizes it. Then the popularizer should get credit for popularizing it (but not for inventing it).' LeCun, however, apparently isn't satisfied with credit for popularising the inventions of others; he also wants the inventor's credit. He is doubling down on an untenable position that's incompatible with the basic universally-accepted rules of scientific integrity [T22].")
ZDNET: Don't believe everything you hear on social media.
YL: I mean, the main paper that he says I should cite doesn't have any of the main ideas that I talk about in the paper. He's done this also with with GANs and other things, which didn't turn out to be true. It's easy to do flag-planting, it's much harder to make a contribution. And, by the way, in this particular paper, I explicitly said this is not a scientific paper in the usual sense of the term. It's more of a position paper about where this thing should go. And there's a couple of ideas there that might be new, but most of it is not. I'm not claiming any priority on most of what I wrote in that paper, essentially.
(Update: Schmidhuber replies, "LeCun claims about me: '... the main paper that he says I should cite doesn't have any of the main ideas that I talk about in the paper.' This makes no sense.I listed not just one but several relevant papers (including [AC90][UN1][AC02][HRL1] [PLAN4]) that contain most of what LeCun explicitly calls his 'main original contributions' [LEC22a]. LeCun says [LEC22c]: 'I'm not claiming any priority on most of what I wrote in that paper, essentially.' However, he listed his 'main originial contributions' [LEC22a] and I showed they were anything but [LEC]. LeCun claims about me 'He's done this also with with GANs.' This false claim has no justification and no references. My gradient-based generative and adversarial NNs of 1990 [AC90-AC90b] described the (frequently cited and implemented and used) basic principles of the 2014 GAN—whose paper [GAN1] failed to correctly assign credit [T22]. My peer-reviewed publication [AC20] on this remains unchallenged. )
Reinforcement learning will also never be enough, LeCun maintains. Researchers such as David Silver of DeepMind, who developed the AlphaZero program that mastered Chess, Shogi and Go, are "very action-based," observes LeCun, but "most of the learning we do, we don't do it by actually taking actions, we do it by observing."
ZDNET: And that is perhaps a good place to start, because I'm curious why did you pursue this path now? What got you thinking about this? Why did you want to write this?
YL: Well, so, I've been thinking about this for a very long time, about a path towards human-level or animal-level-type intelligence or learning and capabilities. And, in my talks I've been pretty vocal about this whole thing that both supervised learning and reinforcement learning are insufficient to emulate the kind of learning we observe in animals and humans. I have been doing this for something like seven or eight years. So, it's not recent. I had a keynote at NeurIPS many years ago where I made that point, essentially, and various talks, there's recordings. Now, why write a paper now? I've come to the point — [Google Brain researcher] Geoff Hinton had done something similar — I mean, certainly, him more than me, we see time running out. We're not young.
ZDNET: Sixty is the new fifty.
YL: That's true, but the point is, we see a lot of claims as to what should we do to push forward towards human-level of AI. And there are ideas which I think are misdirected. So, one idea is, Oh, we should just add symbolic reasoning on top of neural nets. And I don't know how to do this. So, perhaps what I explained in the paper might be one approach that would do the same thing without explicit symbol manipulation. This is the the sort of traditionally Gary Marcuses of the world. Gary Marcus is not an AI person, by the way, he is a psychologist. He has never contributed anything to AI. He's done really good work in experimental psychology but he's never written a peer-reviewed paper on AI. So, there's those people.
(Update: Gary Marcus refutes the claim of lack of peer-reviewed articles. He provided in email to ZDNet the following peer-reviewed articles: Commonsense Reasoning about Containers using Radically Incomplete Information in Artificial Intelligence; Reasoning from Radically Incomplete Information: The Case of Containers in Advances In Cog Sys; The Scope and Limits of Simulation in Automated Reasoning in Artificial Intelligence; Commonsense Reasoning and Commonsense Knowledge in Communications of the ACM; Rethinking eliminative connectionism, Cog Psy)
There is the [DeepMind principle research scientist] David Silvers of the world who say, you know, reward is enough, basically, it's all about reinforcement learning, we just need to make it a little more efficient, okay? And, I think they're not wrong, but I think the necessary steps towards making reinforcement learning more efficient, basically, would relegate reinforcement learning to sort of a cherry on the cake. And the main missing part is learning how the world works, mostly by observation without action. Reinforcement learning is very action-based, you learn things about the world by taking actions and seeing the results.
ZDNET: And it's reward-focused.
YL: It's reward-focused, and it's action-focused as well. So, you have to act in the world to be able to learn something about the world. And the main claim I make in the paper about self-supervised learning is, most of the learning we do, we don't do it by actually taking actions, we do it by observing. And it is very unorthodox, both for reinforcement learning people, particularly, but also for a lot of psychologists and cognitive scientists who think that, you know, action is — I'm not saying action is not essential, it is essential. But I think the bulk of what we learn is mostly about the structure of the world, and involves, of course, interaction and action and play, and things like that, but a lot of it is observational.
ZDNET: You will also manage to tick off the Transformer people, the language-first people, at the same time. How can you build this without language first? You may manage to tick off a lot of people.
YL: Yeah, I'm used to that. So, yeah, there's the language-first people, who say, you know, intelligence is about language, the substrate of intelligence is language, blah, blah, blah. But that, kind-of, dismisses animal intelligence. You know, we're not to the point where our intelligent machines have as much common sense as a cat. So, why don't we start there? What is it that allows a cat to apprehend the surrounding world, do pretty smart things, and plan and stuff like that, and dogs even better?
Then there are all the people who say, Oh, intelligence is a social thing, right? We're intelligent because we talk to each other and we exchange information, and blah, blah, blah. There's all kinds of nonsocial species that never meet their parents that are very smart, like octopus or orangutans.I mean, they [orangutans] certainly are educated by their mother, but they're not social animals.
But the other category of people that I might tick off is people who say scaling is enough. So, basically, we just use gigantic Transformers, we train them on multimodal data that involves, you know, video, text, blah, blah, blah. We, kind-of, petrifyeverything, and tokenize everything, and then train giganticmodels to make discrete predictions, basically, and somehow AI will emerge out of this. They're not wrong, in the sense that that may be a component of a future intelligent system. But I think it's missing essential pieces.
Space
There's another category of people I'm going to tick off with this paper. And it's the probabilists, the religious probabilists. So, the people who think probability theory is the only framework that you can use to explain machine learning. And as I tried to explain in the piece, it's basically too much to ask for a world model to be completely probabilistic. We don't know how to do it. There's the computational intractability. So I'm proposing to drop this entire idea. And of course, you know, this is an enormous pillar of not only machine learning, but all of statistics, which claims to be the normal formalism for machine learning.
The other thing —
ZDNET: You're on a roll…
YL: — is what's called generative models. So, the idea that you can learn to predict, and you can maybe learn a lot about the world by prediction. So, I give you a piece of video and I ask the system to predict what happens next in the video. And I may ask you to predict actual video frames with all the details. But what I argue about in the paper is that that's actually too much to ask and too complicated. And this is something that I changed my mind about. Up until about two years ago, I used to be an advocate of what I call latent variable generative models, models that predict what's going to happen next or the information that's missing, possibly with the help of a latent variable, if the prediction cannot be deterministic. And I've given up on this. And the reason I've given up on this is based on empirical results, where people have tried to apply, sort-of, prediction or reconstruction-based training of the type that is used in BERTand large language models, they've tried to apply this to images, and it's been a complete failure. And the reason it's a complete failure is, again, because of the constraints of probabilistic models where it's relatively easy to predict discrete tokens like words because we can compute the probability distribution over all words in the dictionary. That's easy. But if we ask the system to produce the probability distribution over all possible video frames, we have no idea how to parameterize it, or we have some idea how to parameterize it, but we don't know how to normalize it. It hits an intractable mathematical problem that we don't know how to solve.
"We're not to the point where our intelligent machines have as much common sense as a cat," observes Lecun. "So, why don't we start there? What is it that allows a cat to apprehend the surrounding world, do pretty smart things, and plan and stuff like that, and dogs even better?"
So, that's why I say let's abandon probability theory or the framework for things like that, the weaker one, energy-based models. I've been advocating for this, also, for decades, so this is not a recent thing. But at the same time, abandoning the idea of generative models because there are a lot of things in the world that are not understandable and not predictable. If you're an engineer, you call it noise. If you're a physicist, you call it heat. And if you are a machine learning person, you call it, you know, irrelevant details or whatever.
So, the example I used in the paper, or I've used in talks, is, you want a world-prediction system that would help in a self-driving car, right? It wants to be able to predict, in advance, the trajectories of all the other cars, what's going to happen to other objects that might move, pedestrians, bicycles, a kid running after a soccer ball, things like that. So, all kinds of things about the world. But bordering the road, there might be trees, and there is wind today, so the leaves are moving in the wind, and behind the trees there is a pond, and there's ripples in the pond. And those are, essentially, largely unpredictable phenomena. And, you don't want your model to spend a significant amount of resources predicting those things that are both hard to predict and irrelevant. So that's why I'm advocating for the joint embedding architecture, those things where the variable you're trying to model, you're not trying to predict it, you're trying to model it, but it runs through an encoder, and that encoder can eliminate a lot of details about the input that are irrelevant or too complicated — basically, equivalent to noise.
ZDNET: We discussed earlier this year energy-based models, the JEPA and H-JEPA. My sense, if I understand you correctly, is you're finding the point of low energy where these two predictions of X and Y embeddings are most similar, which means that if there's a pigeon in a tree in one, and there's something in the background of a scene, those may not be the essential points that make these embeddings close to one another.
YL: Right. So, the JEPA architecture actually tries to find a tradeoff, a compromise, between extracting representations that are maximally informative about the inputs but also predictable from each other with some level of accuracy or reliability. It finds a tradeoff. So, if it has the choice between spending a huge amount of resources including the details of the motion of the leaves, and then modeling the dynamics that will decide how the leaves are moving a second from now, or just dropping that on the floor by just basically running the Y variable through a predictor that eliminates all of those details, it will probably just eliminate it because it's just too hard to model and to capture.
Artificial Intelligence
ZDNET: One thing that's surprising is you had been a great proponent of saying "It works, we'll figure out later the theory of thermodynamics to explain it." Here you've taken an approach of, "I don't know how we're going to necessarily solve this, but I want to put forward some ideas to think about it," and maybe even approaching a theory or a hypothesis, at least. That's interesting because there are a lot of people spending a lot of money working on the car that can see the pedestrian regardless of whether the car has common sense. And I imagine some of those people will be, not ticked off, but they'll say, "That's fine, we don't care if it doesn't have common sense, we've built a simulation, the simulation is amazing, and we're going to keep improving, we're going to keep scaling the simulation."
And so it's interesting that you're in a position to now say, let's take a step back and think about what we're doing. And the industry is saying we're just going to scale, scale, scale, scale, because that crank really works. I mean, the semiconductor crank of GPUs really works.
YL: There's, like, five questions there. So, I mean, scaling is necessary. I'm not criticizing the fact that we should scale. We should scale. Those neural nets get better as they get bigger. There's no question we should scale. And the ones that will have some level of common sense will be big. There's no way around that, I think. So scaling is good, it's necessary, but not sufficient. That's the point I'm making. It's not just scaling. That's the first point.
Second point, whether theory comes first and things like that. So, I think there are concepts that come first that, you have to take a step back and say, okay, we built this ladder, but we want to go to the moon and there's no way this ladder is going to get us there. So, basically, what I'm writing here is, we need to build rockets. I can't give you the details of how we build rockets, but here are the basic principles. And I'm not writing a theory for it or anything, but, it's going to be a rocket, okay? Or a space elevator or whatever. We may not have all the details of all the technology. We're trying to make some of those things work, like I've been working on JEPA. Joint embedding works really well for image recognition, but to use it to train a world model, there's difficulties. We're working on it, we hope we're going to make it work soon, but we might encounter some obstacles there that we can't surmount, possibly.
Then there is a key idea in the paper about reasoning where if we want systems to be able to plan, which you can think of as a simple form of reasoning, they need to have latent variables. In other words, things that are not computed by any neural net but things that are — whose value is inferred so as to minimize some objective function, some cost function. And then you can use this cost function to drive the behavior of the system. And this is not a new idea at all, right? This is very classical optimal control where the basis of this goes back to the late '50s, early '60s. So, not claiming any novelty here. But what I'm saying is that this type of inference has to be part of an intelligent system that's capable of planning, and whose behavior can be specified or controlled not by a hardwired behavior, not by imitation leaning, but by an objective function that drives the behavior — doesn't drive learning, necessarily, but it drives behavior. You know, we have that in our brain, and every animal has intrinsic cost or intrinsic motivations for things. That drives nine-month-old babies to want to stand up. The cost of being happy when you stand up, that term in the cost function is hardwired. But how you stand up is not, that's learning.
"Scaling is good, it's necessary, but not sufficient," says LeCun of giant language models such as the Transformer-based programs of the GPT-3 variety. The Transformer devotées believe, "We tokenize everything, and train giganticmodels to make discrete predictions, and somehow AI will emerge out of this ... but I think it's missing essential pieces."
ZDNET: Just to round out that point, much of the deep learning community seems fine going ahead with something that doesn't have common sense. It seems like you're making a pretty clear argument here that at some point it becomes an impasse. Some people say, We don't need an autonomous car with common sense because scaling will do it. It sounds like you're saying it's not okay to just keep going along that path?
YL: You know, I think it's entirely possible that we'll have level-five autonomous cars without common sense. But the problem with this approach, this is going to be temporary, because you're going to have to engineer the hell out of it. So, you know, map the entire world, hard-wire all kinds of specific corner-case behavior, collect enough data that you have all the, kind-of, strange situations you can encounter on the roads, blah, blah, blah. And my guess is that with enough investment and time, you can just engineer the hell out of it. But ultimately, there's going to be a more satisfying and possibly better solution that involves systems that do a better job of understanding the way the world works, and has, you know, some level of what we would call common sense. It doesn't need to be human-level common sense, but some type of knowledge that the system can acquire by watching, but not watching someone drive, just watching stuff moving around and understanding a lot about the world, building a foundation of background knowledge about how the world works, on top of which you can learn to drive.
Let me take a historical example of this. Classical computer vision was based on a lot of hardwired, engineered modules, on top of which you would have, kind-of, a thin layer of learning. So, the stuff that was beaten by AlexNet in 2012, had basically a first stage, kind-of, handcrafted feature extractions, like SIFTs [Scale-Invariant Feature Transform (SIFT), a classic vision technique to identify salient objects in an image] and HOG [Histogram of Oriented Gradients, another classic technique] and various other things. And then the second layer of, sort-of, middle-level features based on feature kernels and whatever, and some sort of unsupervised method. And then on top of this, you put a support vector machine, or else a relatively simple classifier. And that was, kind-of, the standard pipeline from the mid-2000s to 2012. And that was replaced by end-to-end convolutional nets, where you don't hardwire any of this, you just have a lot of data, and you train the thing from end to end, which is the approach I had been advocating for a long time, but you know, until then, was not practical for large problems.
There's been a similar story in speech recognition where, again, there was a huge amount of detailed engineering for how you pre-process the data, you extract mass-scale cepstrum [an inverse of the Fast Fourier Transform for signal processing], and then you have Hidden Markov Models, with sort-of, pre-set architecture, blah, blah, blah, with Mixture of Gaussians. And so, it's a bit of the same architecture as vision where you have handcrafted front-end, and then a somewhat unsupervised, trained, middle layer, and then a supervised layer on top. And now that has been, basically, wiped out by end-to-end neural nets. So I'm sort of seeing something similar there of trying to learn everything, but you have to have the right prior, the right architecture, the right structure.
The self-driving car crowd, startups such as Waymo and Wayve, have been "a little too optimistic," he says, by thinking they could "throw data at it, and you can learn pretty much anything." Self-driving cars at Level 5 of ADAS are possible, "But you're going to have to engineer the hell out of it" and the result will be "brittle" like early computer vision models.
ZDNET: What you're saying is, some people will try to engineer what doesn't currently work with deep learning for applicability, say, in industry, and they're going to start to create something that's the thing that became obsolete in computer vision?
YL: Right. And it's partly why people working on autonomous driving have been a little too optimistic over the last few years, is because, you know, you have these, sort-of, generic things like convolutional nets and Transformers, that you can throw data at it, and it can learn pretty much anything. So, you say, Okay, I have the solution to that problem. The first thing you do is you build a demo where the car drives itself for a few minutes without hurting anyone. And then you realize there's a lot of corner cases, and you try to plot the curve of how much better am I getting as I double the training set, and you realize you are never going to get there because there is all kinds of corner cases. And you need to have a car that will cause a fatal accident less than every 200 million kilometers, right? So, what do you do? Well, you walk in two directions.
The first direction is, how can I reduce the amount of data that is necessary for my system to learn? And that's where self-supervised learning comes in. So, a lot of self-driving car outfits are interested very much in self-supervised learning because that's a way of still using gigantic amounts of supervisory data for imitation learning, but getting better performance by pre-training, essentially. And it hasn't quite panned out yet, but it will. And then there is the other option, which most of the companies that are more advanced at this point have adopted, which is, okay, we can do the end-to-end training, but there's a lot of corner cases that we can't handle, so we're going to just engineer systems that will take care of those corner cases, and, basically, treat them as special cases, and hardwire the control, and then hardwire a lot of basic behavior to handle special situations. And if you have a large enough team of engineers, you might pull it off. But it will take a long time, and in the end, it will still be a little brittle, maybe reliable enough that you can deploy, but with some level of brittleness, which, with a more learning-based approach that might appear in the future, cars will not have because it might have some level of common sense and understanding about how the world works.
In the short term, the, sort-of, engineered approach will win — it already wins. That's the Waymo and Cruise of the world and Wayveand whatever, that's what they do. Then there is the self-supervised learning approach, which probably will help the engineered approach to make progress. But then, in the long run, which may be too long for those companies to wait for, would probably be, kind-of, a more integrated autonomous intelligent driving system.
ZDNET: We say beyond the investment horizon of most investors.
YL: That's right. So, the question is, will people lose patience or run out of money before the performance reaches the desired level.
ZDNET: Is there anything interesting to say about why you chose some of the elements you chose in the model? Because you cite Kenneth Craik [1943,The Nature of Explanation], and you cite Bryson and Ho [1969, Applied optimal control], and I'm curious about why you started with these influences, if you believed especially that these people had it nailed it as far as what they had done. Why did you start there?
YL: Well, I don't think, certainly, they had all the details nailed. So, Bryson and Ho, this is a book I read back in 1987 when I was a postdoc with Geoffrey Hinton in Toronto. But I knew about this line of work beforehand when I was writing my PhD, and made the connection between optimal control and backprop, essentially. If you really wanted to be, you know, another Schmidhuber, you would say that the real inventors of backprop were actually optimal control theorists Henry J. Kelley, Arthur Bryson, and perhaps even Lev Pontryagin, who is a Russian theorist of optimal control back in the late '50s.
So, they figured it out, and in fact, you can actually see the root of this, the mathematics underneath that, is Lagrangian mechanics. So you can go back to Euler and Lagrange, in fact, and kind of find a whiff of this in their definition of Lagrangian classical mechanics, really. So, in the context of optimal control, what these guys were interested in was basically computing rocket trajectories. You know, this was the early space age. And if you have a model of the rocket, it tells you here is the state of the rocket at time t, and here is the action I'm going to take, so, thrust and actuators of various kinds, here is the state of the rocket at time t+1.
ZDNET: A state-action model, a value model.
YL: That's right, the basis of control. So, now you can simulate the shooting of your rocket by imagining a sequence of commands, and then you have some cost function, which is the distance of the rocket to its target, a space station or whatever it is. And then by some sort of gradient descent, you can figure out, how can I update my sequence of action so that my rocket actually gets as close as possible to the target. And that has to come by back-propagating signals backwards in time. And that's back-propagation, gradient back-propagation. Those signals, they're called conjugate variables in Lagrangian mechanics, but in fact, they are gradients. So, they invented backprop, but they didn't realize that this principle could be used to train a multi-stage system that can do pattern recognition or something like that. This was not really realized until maybe the late '70s, early '80s, and then was not actually implemented and made to work until the mid-'80s. Okay, so, this is where backprop really, kind-of, took off because people showed here's a few lines of code that you can train a neural net, end to end, multilayer. And that lifts the limitations of the Perceptron. And, yeah, there's connections with optimal control, but that's okay.
ZDNET: So, that's a long way of saying that these influences that you started out with were going back to backprop, and that was important as a starting point for you?
YL: Yeah, but I think what people forgot a little bit about, there was quite a bit of work on this, you know, back in the '90s, or even the '80s, including by people like Michael Jordan [MIT Dept. of Brain and Cognitive Sciences] and people like that who are not doing neural nets anymore, but the idea that you can use neural nets for control, and you can use classical ideas of optimal control. So, things like what's called model-predictive control, what is now called model-predictive control, this idea that you can simulate or imagine the outcome of a sequence of actions if you have a good model of the system you're trying to control and the environment it's in. And then by gradient descent, essentially — this is not learning, this is inference — you can figure out what's the best sequence of actions that will minimize my objective. So, the use of a cost function with a latent variable for inference is, I think, something that current crops of large-scale neural nets have forgotten about. But it was a very classical component of machine learning for a long time. So, every Bayesian Net or graphical model or probabilistic graphical model used this type of inference. You have a model that captures the dependencies between a bunch of variables, you are told the value of some of the variables, and then you have to infer the most likely value of the rest of the variables. That's the basic principle of inference in graphical models and Bayesian Nets, and things like that. And I think that's basically what reasoning should be about, reasoning and planning.
ZDNET: You're a closet Bayesian.
YL: I am a non-probabilistic Bayesian. I made that joke before. I actually was at NeurIPS a few years ago, I think it was in 2018 or 2019, and I was caught on video by a Bayesian who asked me if I was a Bayesian, and I said, Yep, I am a Bayesian, but I'm a non-probabilistic Bayesian, sort-of, an energy-based Bayesian, if you want.
ZDNET: Which definitely sounds like something from Star Trek. You mentioned in the end of this paper, it's going to take years of really hard work to realize what you envision. Tell me about what some of that work at the moment consists of.
YL: So, I explain how you train and build the JEPA in the paper. And the criterion I am advocating for is having some way of maximizing the information content that the representations that are extracted have about the input. And then the second one is minimizing the prediction error. And if you have a latent variable in the predictor which allows the predictor to be non deterministic, you have to regularize also this latent variable by minimizing its information content. So, you have two issues now, which is how you maximize the information content of the output of some neural net, and the other one is how do you minimize the information content of some latent variable? And if you don't do those two things, the system will collapse. It will not learn anything interesting. It will give zero energy to everything, something like that, which is not a good model of dependency. It's the collapse-prevention problem that I mention.
And I'm saying of all the things that people have ever done, there's only two categories of methods to prevent collapse. One is contrastive methods, and the other one is those regularized methods. So, this idea of maximizing information content of the representations of the two inputs and minimizing the information content of the latent variable, that belongs to regularized methods. But a lot of the work in those joint embedding architectures are using contrastive methods. In fact, they're probably the most popular at the moment. So, the question is exactly how do you measure information content in a way that you can optimize or minimize? And that's where things become complicated because we don't know actually how to measure information content. We can approximate it, we can upper-bound it, we can do things like that. But they don't actually measure information content, which, actually, to some extent is not even well-defined.
ZDNET: It's not Shannon's Law? It's not information theory? You've got a certain amount of entropy, good entropy and bad entropy, and the good entropy is a symbol system that works, bad entropy is noise. Isn't it all solved by Shannon?
YL: You're right, but there is a major flaw behind that. You're right in the sense that if you have data coming at you and you can somehow quantize the data into discrete symbols, and then you measure the probability of each of those symbols, then the maximum amount of information carried by those symbols is the sum over the possible symbols of Pi log Pi, right? Where Pi is the probability of symbol i — that's the Shannon entropy. [Shannon's Law is commonly formulated as H = - ∑ pi log pi.]
Here is the problem, though: What is Pi? It's easy when the number of symbols is small and the symbols are drawn independently. When there are many symbols, and dependencies, it's very hard. So, if you have a sequence of bits and you assume the bits are independent of each other and the probability are equal between one and zero or whatever, then you can easily measure the entropy, no problem. But if the things that come to you are high-dimensional vectors, like, you know, video frames, or something like this, what is Pi? What is the distribution? First you have to quantize that space, which is a high-dimensional, continuous space. You have no idea how to quantize this properly. You can use k-means, etc. This is what people do when they do video compression and image compression. But it's only an approximation. And then you have to make assumptions of independence. So, it's clear that in a video, successive frames are not independent. There are dependencies, and that frame might depend on another frame you saw an hour ago, which was a picture of the same thing. So, you know, you cannot measure Pi. To measure Pi, you have to have a machine learning system that learns to predict. And so you are back to the previous problem. So, you can only approximate the measure of information, essentially.
"The question is exactly how do you measure information content in a way that you can optimize or minimize?" says LeCun. "And that's where things become complicated because we don't know actually how to measure information content." The best that can be done so far is to find a proxy that is "good enough for the task that we want."
Let me take a more concrete example. One of the algorithm that we've been playing with, and I've talked about in the piece, is this thing called VICReg, variance-invariance-covariance regularization. It's in a separate paper that was published at ICLR, and it was put on arXiv about a year before, 2021. And the idea there is to maximize information. And the idea actually came out of an earlier paper by my group called Barlow Twins. You maximize the information content of a vector coming out of a neural net by, basically, assuming that the only dependency between variables is correlation, linear dependency. So, if you assume that the only dependency that is possible between pairs of variables, or between variables in your system, is correlations between pairs of valuables, which is the extremely rough approximation, then you can maximize the information content coming out of your system by making sure all the variables have non-zero variance — let's say, variance one, it doesn't matter what it is — and then back-correlating them, same process that's called whitening, it's not new either. The problem with this is that you can very well have extremely complex dependencies between either groups of variables or even just pairs of variables that are not linear dependencies, and they don't show up in correlations. So, for example, if you have two variables, and all the points of those two variables line up in some sort of spiral, there's a very strong dependency between those two variables, right? But in fact, if you compute the correlation between those two variables, they're not correlated. So, here's an example where the information content of these two variables is actually very small, it's only one quantity because it's your position in the spiral. They are de-correlated, so you think you have a lot of information coming out of those two variables when in fact you don't, you only have, you know, you can predict one of the variables from the other, essentially. So, that shows that we only have very approximate ways to measure information content.
ZDNET: And so that's one of the things that you've got to be working on now with this? This is the larger question of how do we know when we're maximizing and minimizing information content?
YL: Or whether the proxy we're using for this is good enough for the task that we want. In fact, we do this all the time in machine learning. The cost functions we minimize are never the ones that we actually want to minimize. So, for example, you want to do classification, okay? The cost function you want to minimize when you train a classifier is the number of mistakes the classifier is making. But that's a non-differentiable, horrible cost function that you can't minimize because, you know, you're going to change the weights of your neural net, nothing is going to change until one of those samples flipped its decision, and then a jump in the error, positive or negative.
ZDNET: So you have a proxy which is an objective function that you can definitely say, we can definitely flow gradients of this thing.
YL: That's right. So people use this cross-entropy loss, or SOFTMAX, you have several names for it, but it's the same thing. And it basically is a smooth approximation of the number of errors that the system makes, where the smoothing is done by, basically, taking into account the score that the system gives to each of the categories.
ZDNET: Is there anything we haven't covered that you would like to cover?
YL: It's probably emphasizing the main points. I think AI systems need to be able to reason, and the process for this that I'm advocating is minimizing some objective with respect to some latent variable. That allows systems to plan and reason. I think we should abandon the probabilistic framework because it's intractable when we want to do things like capture dependencies between high-dimensional, continuous variables. And I'm advocating to abandon generative models because the system will have to devote too many resources to predicting things that are too difficult to predict and maybe consume too much resources. And that's pretty much it. That's the main messages, if you want. And then the overall architecture. Then there are those speculations about the nature of consciousness and the role of the configurator, but this is really speculation.
ZDNET: We'll get to that next time. I was going to ask you, how do you benchmark this thing? But I guess you're a little further from benchmarking right now?
YL: Not necessarily that far in, sort-of, simplified versions. You can do what everybody does in control or reinforcement learning, which is, you train the thing to play Atari games or something like that or some other game that has some uncertainty in it.
ZDNET: Thanks for your time, Yann.