MIT and Tsinghua scholars use DeepMind's AlphaFold approach to boost COVID-19 antibodies
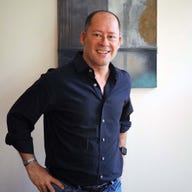
The world of structural biology was stunned in late 2020 by the arrival of AlphaFold 2, a second version of the deep learning neural network developed by the Google artificial intelligence unit DeepMind. AlphaFold solved a decades-old problem of how proteins fold, a key fact governing their function.
Recent research shows the approaches pioneered by AlphaFold are spreading to the broader biology community. In a paper in the journal PNAS this month, "Deep learning guided optimization of human antibody against SARS-CoV-2 variants with broad neutralization," scientists describe modifying a known antibody against COVID-19 in such a way as to boost its efficacy against multiple variants of the disease.
"We enable expanded antibody breadth and improved potency by ∼10- to 600-fold against SARS-CoV-2 variants, including Delta," starting from an antibody that had no effectiveness against the Delta variant, the scientists write. They even find promising signs the approach can work against the Omicron variant.
The research, conducted by Sisi Shan and colleagues at Tsinghua University in Beijing; the University of Illinois at Urbana–Champaign; and Massachusetts Institute of Technology, makes use of deep learning for two very important reasons.
One is to expand what's called the search space, the set of potential solutions to modifying antibodies. Existing approaches, such as random mutagenesis, while valuable "are time consuming and labor intensive." So using deep learning is a way to automate and thereby speed up efforts.
Second, approaches such as random mutagenesis can take away the good parts of an antibody at the same time they confer benefits. The result may be suboptimal. By using a deep learning approach, the authors hope to extend efficacy while also conserving what had been accomplished. They seek not merely to improve but to optimize.
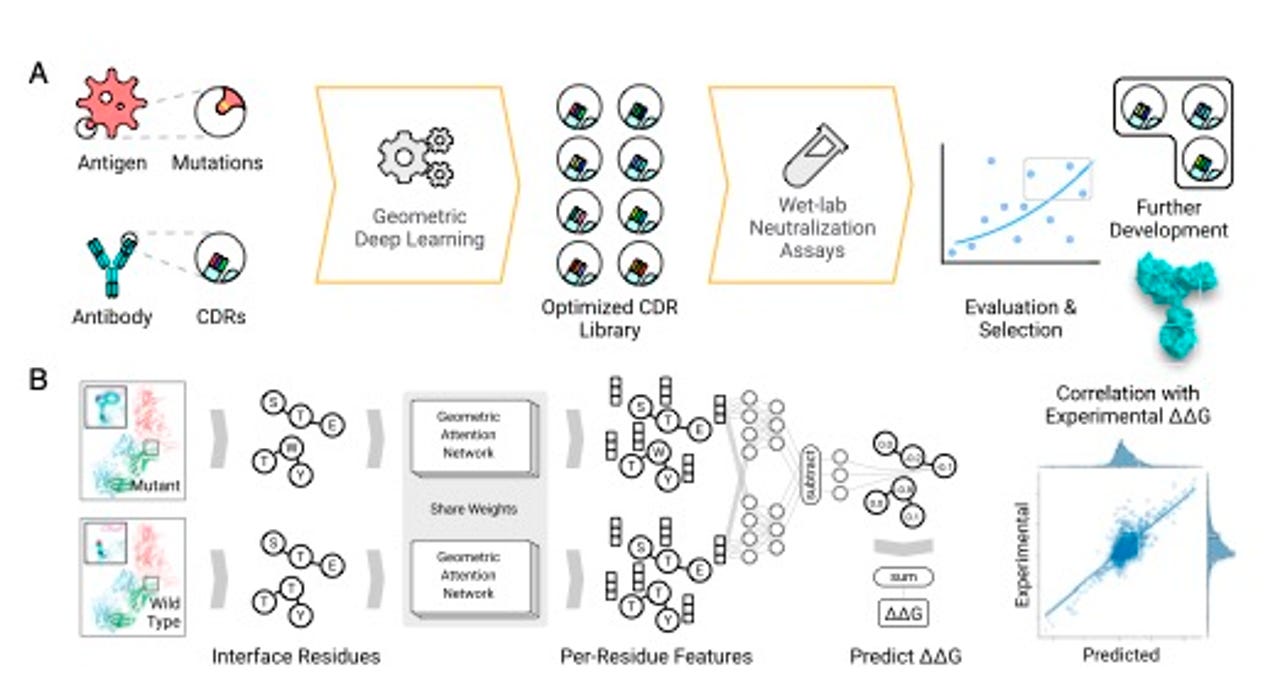
The graph embedding attention procedure for finding pairs of residues that are significant to predict binding affinity.
Their approach employs the essential techniques of AlphaFold 2: a graph network, and a treatment of variables called "attention."
Also: DeepMind's AlphaFold 2 reveal: Convolutions are out, attention is in
A graph network is when some collection of things can be assessed in terms of their relatedness, such as people in a social network. AlphaFold 2 used information about proteins to construct a graph of how near to one another different amino acids are. Those graphs were then manipulated by the attention mechanism to compute how much each amino acid relates to another amino acid.
Shan and colleagues take the same approach, and they apply it to the amino acids of both the virus, the antigen, and also the amino acids of the antibody. They compare so-called wild types with mutated forms of both, to determine how binding of the antibody to the antigen changes as the amino acid pairs of both change between wild type and mutated version.
To train a deep neural network to do that, they set a goal, what's known in machine learning as the objective function, the target that the neural network tries to replicate. The objective function in this case is the free energy change, the change in energy of proteins from wild type to mutant, symbolized by the Greek letters delta-delta, and G, or ΔΔG. Given a target free energy, the neural network is developed to a point where it can reliably predict which amino acid pair changes will come closest to the target free energy change.
As Shan and colleagues describe their approach,
To estimate the effect of mutation(s), we first predict the structure of mutated protein complexes by repacking side- chains around mutation sites and encode both wild-type (WT) and mutated complexes using the network to obtain both WT and mutant embeddings. Then, additional neural network layers compare the two embeddings to predict the effect of mutation measured by ΔΔG.
Although Shan and team refer to AlphaFold 2, and they employ the approach of AlphaFold 2, they did not use DeepMind's code. The new work, referred to as the ΔΔG Predictor, was written entirely from scratch, co-author Bonnie Berger of MIT told ZDNet in an email exchange.
Because both ΔΔG Predictor and AlphaFold 2 are open source, you can examine them for yourself and see how the code compares. The ΔΔG Predictor code can be seen on its GitHub page, and AlphaFold 2 can be seen on its page.
After training the neural net to predict both significant antibody and antigen mutations, the authors work backward from evidence of where the antibody has, in fact, been successful, in the case of the Alpha, Beta, and Gamma versions of COVID-19. They use that data to predict which mutated antibodies will extend efficacy.
As the authors put it,
Our method generated an in silico mutation library of antibody CDRs, ranked by trained geometric neutral networks in such a way that they should not only improve antibody binding to the Delta RBD but also maintain binding to the RBD of other var- iants of concern (VOC).
The CDR, or complementarity-determining region, is the part or an antibody that binds to an antigen. The RBD, the receptor binding domain, is the main target on the virus.
The researchers derive double, triple and even quadruple-mutated antibodies. Testing them in a lab against synthesized virus, they find increasing strength in reducing the antigen concentration as the mutations compound. They conclude something is "binding" better between the mutated antibody and the virus. "Antibodies HX001-020, HX001-024, HX001-033, and HX001-034 with three or four mutations were also stronger than HX001-013 with only two mutations," they write. "The increase in binding affinity may contribute to the increased neutralizing activity of these antibodies against SARS-CoV-2 WT and variants."
Among the provocative findings is that it may be enough for a mutated antibody to avoid a problematic mutation in the virus in order to be more effective. In a structural analysis, they find that one part of the original antibody is brushing up against a particular part of the antigen, and the two are repelling each other.
"Since both [antibody particle] R103 and [antigen particle] R346 have very long sidechains and carry positive charges, proximity between the two may introduce strong repulsion which may greatly reduce the binding affinity between the antibody and the antigen," they observe.
The scientists substitute for the normal antibody particle and, "we no longer observe a direct interaction with R346 on the Delta RBD [receptor binding domain]. This factor might explain the greatly improved neutralization against the Delta variant."
The research is made all the more interesting by the fact that the antibody the authors work on is one that was introduced last year by Shan and colleagues. Called "P36-5D2," the antibody was obtained by being isolated from the blood serum of a recovering patient infected with COVID-19. It was determined through animal model studies by Shan and team to be a "broad, potent, and protective antibody."
Hence, the new work marks what could be a milestone in AI: extending conventional wet-laboratory methods in infectious disease treatment by refining a traditional biological product with novel computer-driven methods.