NeuroMetrix CEO reflects on the art of AI in the art of healing
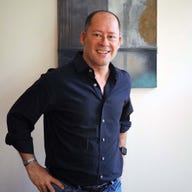
Featured
Identifying cat pictures is a no-brainer for AI, but applying machine learning to healthcare is a delicate matter of making choices about what will help a person versus what is merely interesting research.
That fact is expressed in the approach to artificial intelligence taken by a Waltham, Mass-based company called NeuroMetrix, which sells a novel gadget to ease chronic pain.
CEO Shai Gozani, an M.D., PhD, is enthusiastic about AI, but not religious; in fact, he has a sense of humor about the recent resurgence in popularity of neural networks.
"I was doing computer science in the mid 80s at Berkeley, and AI was big," Gozani recalled during an interview last week with ZDNet. "And then I went to medical school, and two or three years ago, AI was suddenly big again, and I said to myself, Hey, this is the same thing that I heard about at that course I went to back then, between those Bruce Springsteen concerts!"
Also: AI startup People.ai gets $30 million from Andreessen Horowitz to free up workplace drudgery
In all seriousness, NeuroMetrix, which sells a device called "Quell," is finding the value in machine learning applied to large data sets of individuals' pain. The next move may be to apply deep learning forms of computation, such as reinforcement learning, to further enhance the product's features. (The Quell Web site has a bunch of other research papers from the company that can be downloaded.)
NeuroMetrix went public on Nasdaq in January of last year. Although its stock has lost roughly a quarter of its value since the IPO, shares have nearly doubled since their December lows.
The Quell product, which sells for $299 for the just-released second version, and $249 for an older, somewhat bulkier model, is a cuff, made of a combination of spandex, nylon, and polyurethane, that one wears around one's calf. (There is no neoprene or latex in the cuff, the company emphasizes.) The cuff is secured via velcro on the ends, and via some gel pads on the inside that are slightly adhesive. Inside the cuff are electrodes that emit small electrical currents to the skin, on the order of one hundred milli-amps, for fractions of a second at a time. The pulses are administered either automatically or through manual selection from an accompanying smartphone app.
NeuroMetrix CEO Shai Gozani, left, and chief commercial officer Frank McGillin, at the company's booth at the Consumer Electronics Show in Las Vegas, earlier this month.
The goal is to treat chronic pain by sending light electrical currents through the skin. Pain in this case can include things such as lower-back pain. The principle of the electrical pulses is what's called "Transcutaneous electrical nerve stimulation," or TENS. The Quell product uses a particular variant, called "fixed-site high frequency TENS," or "hfTENS," where the stimulation is applied in one area, in this case the calf, regardless of where the pain is. The pulses travel up the nerves to the central nervous system in the brain to affect whatever site on the body has pain.
Also: Analytics startup EDO teases consumer behavior from TV ads with machine learning
For the three years the product has been on the market, it's been all about finding out how the product helps and how people use it, says Gozani, because data, even more than the algorithms, is key.
"The place to start is the data," he says. "If it's not relevant, it's an exercise in futility."
Seventy thousand individuals have contributed data points that have been aggregated in NeuroMetrix's cloud computing facility, Gozani says, over the course of months and in some cases years of use. It is the "richest chronic pain data set in the world," he says.
"What's really most important for machine learning is how many different people do you have, how many chronic pain patients, because each one is unique," he says.
Data is collected in two places, from the device itself and from the companion app. The device can register things such as a person's gait, their style of walking, which can be particularly important, Gozani says, because abnormalities of gait can be precursors to things such as a fall, especially in older individuals.
The app lets a person enter demographic data, such as age and height, and record where and when they feel pain, and attendant phenomena such as mood.
Also: AI startup InsideSales asks if a machine can be taught to sell
The purpose of compiling the data over time is to assemble baselines that can decide for a person how much and how often to emit the pulses. This approach does not require complicated neural networks, Gozani says. Simple linear regression can and has achieved a lot, he says, because the relationships being modeled in the data are not so complicated that they require deep learning.
The use of AI has evolved over time. When Quell first came out, it was designed to employ something like a "best guess" of how much stimulation to apply, Gozani says.
"Seventy thousand patients later, we can say you have this profile, which matches these commonalities: You are such and such height, say, you are a male, you have pain in your legs but not in your shoulder, etc." As a result of patterns deduced about such a population, the device has been able to develop more accurate recommended dosages.
Relating an individual to the growing baseline of pain and care is "the single most important thing," Gozani says. "If you get that right, you can give people a lot more benefit right out of the box."
The value of studying patient data is emphasized by a peer-reviewed paper published this month describing a randomized control trial conducted by NeuroMetrix in partnership with Harvard Medical School, the first-ever RCT of hfTENS.
The Quell cuff on display with its companion smartphone app and the strip of electrodes that make it work, at the company's CES booth.
The use of machine learning for Quell will continue to evolve, says Gozani. The company is actively researching various deep learning methods to see how they may enhance Quell's capabilites. For example, the weather can be an important factor in pain, as understood thousands of years ago by Hippocrates, Gozani notes.
"[Barometric] pressure, for example, can become a factor in some people's pain," he observes. The company already gathers weather data in its cloud operations, and has used some "supervised learning" to see how patients cluster by weather patterns in their geographic area. The next step could be to use deep learning to perform predictive analysis about how weather should influence the administering of the pulses on an individual basis.
"If we monitor the weather for you, we might be able to know we should increase a dosage for you today because the weather is going to be bad," he says, "and that's going to contribute to your having a higher level of pain." That kind of predictive algorithm is a higher-dimensional problem than the baselines for which linear regression is used, and so it might be a natural place where deeper networks are useful, he muses.
Also: IBM, Apple, and Facebook represent new tilt toward business for venerable AI conference
That thought leads naturally to the potential for "reinforcement learning," a branch of machine learning in which the computer decides on which of various actions to take given the shifting nature of a state of affairs and a based on a reward function that is attached to successful outcomes. Gozani says the company is "very interested" in reinforcement learning.
Must read
- 'AI is very, very stupid,' says Google's AI leader CNET
- Baidu creates Kunlun silicon for AI
- Unified Google AI division a clear signal of AI's future TechRepublic
"The user has to make decisions," about administering pulses, he observes, "and a question is: Can something like reinforcement learning help them to make those decisions in a better way?"
Reinforcement learning could be embedded in the device, he says, so that by observing the fact that the user just made a decision, and the decision made things better, something is learned about choices. "We are collecting that data already on patients, but we are not yet learning you, the user, in real time," he says, "that would be the next step."
With reinforcement learning, or deep learning, or any such approach, "AI is a tool," Gozani says. "We are trying to get customers to a good outcome, that's the point. And if we can get them there faster with some tool, then that's of interest to us."
Scary smart tech: 9 real times AI has given us the creeps
Previous and related coverage:
What is AI? Everything you need to know
An executive guide to artificial intelligence, from machine learning and general AI to neural networks.
What is deep learning? Everything you need to know
The lowdown on deep learning: from how it relates to the wider field of machine learning through to how to get started with it.
What is machine learning? Everything you need to know
This guide explains what machine learning is, how it is related to artificial intelligence, how it works and why it matters.
What is cloud computing? Everything you need to know about
An introduction to cloud computing right from the basics up to IaaS and PaaS, hybrid, public, and private cloud.