Practical AI for the enterprise: Getting past vendors blowing smoke
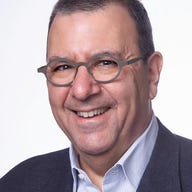
Virtually every enterprise software vendor is pushing AI -- machine learning, cognitive computing, deep learning, and related technologies -- as the ultimate set of technologies to change your business, life, and the world.
Despite this hype, or perhaps because of it, many vendors blow smoke when it comes to their own AI capabilities. Sol Rashidi, chief data and cognitive officer of the cruise line Royal Caribbean, describes her experience trying to buy from AI vendors. I spoke with her on episode 247 of the CXOTalk series of conversations with innovators:
We've got over thirteen-hundred companies who are invested in AI, about $9 billion of research is going into AI. So, we have plenty of options. Our challenge is understanding which vendor to choose, based on their longevity within the industry and their ability to demonstrate and perform and execute. Most companies are still being seed-funded, so longevity is still very key for us. Also, understanding their capabilities and functionalities because unfortunately, of that thirteen-hundred that say they're AI-based, [the only thing] many really offer is a chatbot.
Chatting is not a new technology. Yet, they're putting it under the AI umbrella just because there's a bit more glamor to it. So, we have to sift through, unfortunately, a lot of companies who claim to be AI companies, but aren't.
Unfortunately, this sentiment reflects the harsh reality that many enterprise buyers face today. Frankly, the situation has become ridiculous.
To cut through the hype and address practical issues of deploying AI in the enterprise, I invited Tiger Tyagarajan, president and CEO of Genpact, a professional services company with almost $3 billion in annual revenue, to appear on episode 246 of CXOTalk.
Industry analyst, Phil Fersht, is CEO of HFS Research and one of the world's top authorities on professional services firms. I asked him for context on Genpact's relationship to AI in the enterprise:
Genpact turned the BPO model on its head with its disruptive, virtual captive proposition that significantly challenged the pricing models and ability to integrate offshore capabilities into the old BPO model.
The firm is breaking the mold yet again, by infusing AI into business processes and introducing these concepts to its huge global community of finance leaders. Automation is about digitizing the backoffice, but artificial intelligence marries business processes with clever technology and self-developing algorithms.
Genpact's emerging focus is on embedding smart cognitive applications into process chains and workflows; it's about learning from mistakes and gaining new experiences along the way. This is the emerging "organization neural system," in which real-time, self-learning and intelligent operations support customer needs.
The conversation with Tiger Tyagarajan focuses on practical aspects of deploying AI. As the comments from Phil Fersht make clear, he is eminently qualified to advise us on these matters.
Watch the entire conversation in the video embedded above and read edited excerpts below. You should also check out the complete transcript of our conversation.
How should large organizations approach AI?
Leveraging AI across the business, in its entirety, is too much ocean to boil. That's not necessarily the most effective, efficient way of bringing AI to bear onto a problem.
We have found the most success when a client leverages AI to attack and solve [specific problems]. Let's say, managing receivables or working capital, sending bills out, and all the administrative aspects of that. [When the client] doesn't want to use labor because it's too painful and therefore wants to use a machine to get better and better.
When AI is brought to bear on a specific problem, we see the best answers emerge. When AI attempts to solve the whole company, it's a much, much different journey and often people get disappointed.
Sometimes, the board, CEO, and CXO team say, "We want to use AI in the company. Let's just bring AI into the company." Well, after some time, they realize the better way is artificial narrow intelligence, ANI, narrowing down the problem to the most valuable one we can solve and really attacking it with AI. That's often called "artificial narrow intelligence." We find those projects to be highly successful.
The biggest opportunity is where the value is the highest. You typically try and do that with a proof-of-concept where the problem is big, the opportunity is big, and if solved for, could be a huge benefit, not a small benefit.
It's not that difficult, and it's worth doing it because these are short experiments. The AI-based tool alone does not drive as much value as if you embed it in a workflow.
How can AI help with digital transformation?
There is an incredible role for AI in creating and standing up new business models. To call them innovative business models is an understatement. Most often, these highly disruptive, innovative new business models have, as new technologies like AI as one of their core [components].
Let me give you one interesting example: insurance.
We love our cars and obviously must make sure they are well-insured. Occasionally, we have to file an insurance claim. The good way of doing that, and a lot of companies do very well: I have a minor accident, I stop on the highway, I make my 1-800 call, I then get instructions to what I'm going to do, wait there; pickup truck comes in; someone says, "We'll get back to you with an assessment of what this is going to cost you."
We'll also then make sure that the insurance company knows what this is going to cost you, and then this whole process that kicks in, coming back with, "I think the insurance company is going to foot $800.00 for this damage from your insurance claim."
That process takes time, effort, and multiple follow-ups. It's not great customer service despite a great effort by most insurance companies. And, there's angst throughout the whole thing.
You can have a new business model, that many insurance companies are trying -- same accident, except you open your smartphone, open the app, and then just press the button that you had an accident.
Automatically, it triggers someone, for example, an Uber driver, who arrives at your spot in five to seven minutes, takes out his or her iPhone, and starts clicking photographs of everything that he or she can see at that moment, in that car; inside, outside. And, there's a set of instructions that that Uber driver must follow.
Instantaneously, as those photographs are taken, an AI tool looks at them, applies computer vision based on more than ten million images that it already has, and says, "Based on the machine's assessment of damage, these are the kind of repair costs that will be applicable. Therefore, this insurance claim should be paid to the extent of $747.00."
In 20-30 percent of the cases, it kicks off to an expert to decide. In fifteen minutes, the message comes back on the same smartphone, telling the customer that the insurance claim is approved for $747.00, so get your stuff repaired, and that's it.
Think about the customer satisfaction, the retention of that customer. Next car that the customer buys, it's the same insurance company. Think about the value to the insurance company. You can go on and on. That is a disruptive business model. Any insurance company that finds a way to grapple with this, solve it, is going to disrupt the industry. It will change how that industry works.
What is the role of expert knowledge in success with AI?
AI really becomes amazing only if it satisfies two conditions.
Condition number one: you must apply AI to the context of the business and the problem; immerse it in the domain of that problem, in the domain of the industry.
For example, do [the technologists] really know what happens in an accident like that I just described, on an interstate highway? Let's call it a Honda Accord in the state of Michigan, on an interstate highway, etc., etc., etc. If it happens at noon, or midnight, here's what to expect, etc.
In all of that, do you really understand the insurance business? Do you really understand auto insurance in the US, in Michigan? Do you really understand how a customer interacts during or filing of an auto claim? Do you understand how fraud happens in the claims process, in auto claims? Because frauds do happen. The depth of domain knowledge makes a real difference.
The second thing that makes a difference is whether you have enough data. Do you have enough data about past claims? Do you have enough data on all the images and photographs taken in the past? Do you have enough data to create the intelligence that is needed?
Without context of the domain and applying it to data, AI doesn't come alive and doesn't create value. So, we are big believers in AI plus domain, plus data. Without domain and data, there is no value.
How can companies ensure they have both domain knowledge and data?
It is complicated, it is interrelated, and it does take time to solve these problems in a fully industrialized way [at scale]. One could call that the bad news because it's big, and it's hairy, and it's complex.
The good news is that you can break it down into its pieces and its components. You can hone it down to, "Can we start at the proof of concept?" And in that proof of concept can we test it quickly? I'm okay if I don't get the full value on day one. That's the good news. The second good news is that the value is so big when it's fully done that the effort is worth it, which is why it's important to pick the right problem.
So, pick a big problem, hone it down to a proof of concept, and then iterate your way through a series of experiments. It's okay to not solve all the problems that you described on day one. You can solve parts of the problem, knowing full well that you might get only 20-30 percent of the decisions taken by the AI technology. But, that's 20-30 percent, versus zero. Then, you improve. And, in its tenth iteration, it's already got to 85 percent.
The second problem is making sure that people buy into a series of experiments.
And then finally, there's a third problem as you get through the series of experiments and want to scale up. This is where I think AI can fail in solutions and in an enterprise, which also applies to almost all digital technologies. When you scale up, you need a governance layer that watches these technologies.
One of the dangers is an assumption that AI doesn't require governance or being watched: the assumption that it's all well-coded and will work forever. The fact is that business models change, policies change, regulations change, lots of change, change, change. You've got to keep watching all the technologies that you laid out. That needs a governance layer.
You almost need a platform to watch all of that, so when something changes in the business, you can make changes in the technology. We think that's very, very important and a lot of our journeys are around that topic.
CXOTalk brings together the most world's most innovative leaders for in-depth conversations on AI and innovation. Be sure to watch our many episodes!
Thumbnail image credit: Creative Commons photo illustration by Chuck Grimmett.