SAS wants to spread its footprint
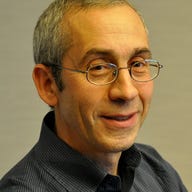

When BI emerged back in the 1990s, SAS was already a mature player that catered to a narrow elite of data miners and quants. You may have used Business Objects or MicroStrategy to generate sales by region dashboards, but when it came to crunching deep analytic models, SAS had few rivals. For SAS, the overriding theme in recent years has been broadening its footprint in a landscape where AI (mostly machine learning) is reshaping BI and driving the need to treat modeling, not as a one-off but as a lifecycle that integrates with operational systems both on-premises and in the public cloud. Both developments are making the landscape where SAS competes increasingly crowded, from the self-service visualization tools of business analysts, and the data science collaboration tools that are coming from an expanding array of venture-backed startups.
Then there's open source, the elephant in the room, which SAS no longer treats as a mortal threat but a heterogenous system; SAS's contribution is applying process and governance that picks up where open source leaves off. When it introduced SAS Viya three years ago, SAS opened a path for data scientists to write models using Python, and later, Java, Python, Lua, and R, then invoke SAS routines in Viya on the back end. But until recently, SAS had a perception issue with Viya, as many prospective customers had the impression that it was merely a Tableau-style pretty face instead of a new analytics platform tackling the full lifecycle. But SAS is finally starting to get the Viya story out. Given that SAS's growth has hovered around the 1 – 2 % range for the past five years, perceptions are critical for the company to spark renewed growth.
2018 was where Viya finally took off, with sales roughly doubling. Meanwhile, SAS's cloud business grew just over 30%, primarily from the Results as a Service offering, where you feed your data to SAS-managed models.
Since launching, Viya has added to its portfolio. The highlight at launch, SAS Visual Investigator, pieced together capabilities from a variety of separate SAS tools, ranging from Visual Analytics to Decision Manager, Enterprise Miner to Visual Statistics and others. The Viya portfolio has since expanded considerably, encompassing data preparation, econometrics, model lifecycle management, optimization, data mining and machine learning, text analytics, computer vision and natural language interaction.
Near term, SAS's goals include engaging a wider audience within its client base. Part of it comes through more flexible user-based licensing so customers do not have to make hard commitments to any individual products or modules from the stack or worry about changing compute images or data volumes. The other prong is through end-to-end capabilities supporting business analysts and data scientists alike, plus new more industry-specific vertical solutions such as credit fraud detection. It's also introducing more flexible pricing to make it easier to license SAS without having to make hard commitments to any individual module or product from the portfolio.
To those in the peanut gallery who want to get updated on what we saw at this year's SAS analyst event, the focus was not on the product roadmap per se, but the end-to-end journey. The message is that, beyond the large portfolio of SAS analytics functions are tools that support each step of the analytic lifecycle where the appeal is being broadened beyond SAS's usual constituency and into enterprise operations when analytics becomes key.
The trip spanned from data management to model management, data discovery, and deployment featuring deep dives into selected use cases. Examples included showing how SAS ESP ingests and transforms real-time streaming data in a data pipeline that also enriches with data at rest to support cancer research. From that pipeline, you can use SAS or third-party analytic tools. There was the phase of data discovery, using an example with credit fraud prevention that provided GUI-driven environments for the business user and model building, competition, and training for the data scientist. Then there was the focus on deployment, providing the metrics on deployment and lineage (of data and the model), and last-mile applications such as SAS Intelligent Decisioning being applied to customer next-best offer scenarios.
Clearly, SAS has plenty of company in different parts of the analytics lifecycle, from modeling and model lifecycle management to analytics, reporting, and self-service. SAS's big draw has always been the breadth and depth of its analytics functions. As one customer put it, open source frameworks or libraries will typically take the 80/20 rule, such in classification or regression analysis, while SAS will venture beyond with the more esoteric stuff or analytic functions that are shaped for specific industry sectors.
But the functional gulf between SAS and the open source world is a battle for time that has a generational spin to it. In 40 years, SAS has had the opportunity to develop a deep and wide portfolio of analytic functions. With the enthusiasm for open source, especially among incoming data scientists and data engineers, there is a strong push in the community to expand the portfolio in the open source wild. We've also seen how sectors that traditionally kept development to themselves are increasingly embracing open source, either through open source-first strategies (use open source technology where it is viable) or building their own open source projects. A decade ago, tech meetups were practically non-existent in New York. Today, a week rarely goes by without somebody from Wall Street or the media world demonstrating their new open source contributions.
As noted above, SAS has taken the first steps by meeting the open source community halfway by making SAS analytics on Viya accessible to non-SAS languages. We've gone on record previously that SAS should take the next step and start contributing to open source as well. At the end of the day, SAS's core value-add will not just be its rich analytics functionality, but also how it helps you manage and implement analytics, and how SAS delivers it.
Consider what SAS has already delivered with its Viya portfolio for repackaging, and its Results as a Service for delivery, Now imagine once SAS containerizes its portfolio in cloud-native format; that could help SAS accelerate the refactoring the analytics portfolio as cloud services. Then add a broad third-party partner ecosystem (a discipline that is new to SAS), and SAS can compete as the analytics destination.
What about innovation? While it may be a matter of time before the open source world gets within hailing distance of the richness of SAS's analytic function portfolio, there still is plenty of opportunity for SAS to differentiate through innovation. Last year, Dr. Jim Goodnight, SAS's CEO, called for explainability as the missing ingredient with AI models. This year, we saw SAS demonstrate its current and future capabilities in model explainability that includes natural language feature in Viya model management and across the rest of the Viya Portfolio. SAS can also document the lineage of the model and data, and the intent of the model. SAS is not the only player that is tackling this problem. Just a few weeks back, we saw H2O demonstrate its approach to model explainability. But take our word for it. On this round, SAS's machine learning model explanations were far easier to understand.