SAS is on the brink of generation change
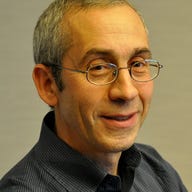
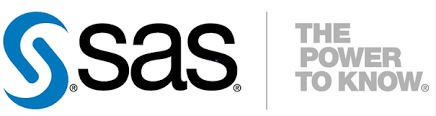
Last year, we provided a Star Trek metaphor describing SAS's evolution as we watched CEO and co-founder Dr. Jim Goodnight demonstrated how to use Alexa to query SAS. A year later, we watched a similar demo using Siri with an iPhone, giving us an idea of the heavy lift that is still required to make conversational interfaces reality.
Clue: You still have to phrase your questions precisely because, as of now, speech recognition systems have not caught up to the autocorrect features that we take for granted when typing Google searches.
But we digress. With the accession of Dr. Oliver Schabenberger from chief of research, product development, and most recently, CTO, to the post of COO (reporting only to Dr. Goodnight), SAS has completed a changing of the guard that began three years ago. With Dr. Schabenberger, SAS has promoted a product researcher with deep academic background (like Dr. Goodnight) to head product development, sales, and operations. SAS characterizes the newly created COO slot as a plan to "expand" the management team. Watch this space.
The challenge for SAS is that a market where it had unquestioned dominance for the lion's share of the last 40 years is now getting quite crowded. With the cloud, you don't need to invest in high-performance compute infrastructure, and with open source, you don't have to pay a lot for this muffler (if that muffler were software). Not surprisingly, an ecosystem of tools for automation, collaboration, orchestration, and deployment for the data science lifecycle has sprouted.
With emergence of Viya over the past couple years, SAS smartly concluded that its value add was not in the SAS programming language, but in the breadth and depth of analytics in its portfolio and the way it manages them. With Viya, SAS chose to coexist with the open source environment. Develop in R or Python, use a Jupyter notebook to compose and share your models, but at runtime, send them to Viya where the models run in SAS's Cloud Analytic Services (CAS) in-memory engine. Or share your algorithms with SAS tools such as Visual Analytics or Visual Investigator.
So SAS has opened the door, but the biggest threats are not necessarily open source, but the ease of access to advanced analytics and machine learning (ML) through the dozens of fit-for-purpose services from each of the major cloud providers. Want to build an ML model in a highly curated environment? Go to Amazon Sagemaker or Azure ML Studio. Want to build some image recognition or speech to text applications? Go to Amazon Rekognition or Google Cloud Natural Language. Want to run some Spark jobs? Go to Amazon EMR or Databricks. As SAS touts its well-rounded environment, these cloud services offer quick onramps to the types of analytics that have been SAS's bread and butter; if you already have your data in cloud storage (which is already your data lake), just give a credit card number.
At this year's analyst conference, we should not have been surprised that the spotlight drifted to artificial intelligence (AI) and ML, as they have pretty much seized the agenda in the analytics world. SAS felt the need to assert that ML and its precursors have always been ingrained into its analytics. Let's just say that SAS came late to the ML hype.
SAS is undergoing the rite of passage that is inevitable with first-movers, as it is challenged to continue demonstrating its relevance in a marketplace it has historically dominated and now shares with numerous upstarts.
SAS has a firm hold on its Global 2000 enterprise base, which isn't going away anytime soon. But it is challenged to compete for the hearts and minds of the next generation of data scientists, data engineers, and business analysts who have become empowered by self-service and drawn in by open source.
There are ways for SAS to get cool again. We recall several years back, flying to a conference (actually, it was SAS), where we found ourselves sitting next to a high school senior who was on his way to UT Austin to check out their data science program. Self-taught, he was already proficient in R and Python, but preferred SAS because of the full-featured analytics environment. That's the scenario that SAS wants to replicate.
Towards that end, SAS has long invested in higher education programs for training the next generations of SAS programmers. But now it must do more: it must meet young practitioners where they live: in the cloud and in the open source community. For them, cloud makes the onramp easy, while open source is synonymous with the place that the community innovates. And by the way, open source provides career portability. Learn a proprietary tool, and you can only go to companies that use that tool; learn open source, and you can go almost anywhere.
Let's start with the cloud. It's still early days for SAS on SaaS. A limited group of SAS customers are using cloud services from its own data centers (near Cary, NC headquarters and Frankfurt) or services that SAS hosts in public clouds. It also offers a managed SAS analytics services on AWS Marketplace, but strictly for higher education. Viya, which is "cloud-friendly" because it runs on elastic CAS infrastructure, is not yet offered as a managed cloud analytics platform-as-a-service. Today, going to the cloud, you would have to deploy it yourself through infrastructure-as-a-service.
That's going to change. SAS is now in the process of re-architecting its analytic tools into containers and microservices that are far more cloud-friendly; we expect that the first containerized SAS analytic services will debut on the Viya platform. The re-architecting is significant, because containers and microservices offer two key advantages.
First, they utilize cloud resources far more efficiently, which means that managed service providers like SAS can price its SaaS offerings more competitively.
Secondly, by refactoring monolithic applications and tools into containers and microservices, SAS can more readily create new cloud-based services, like SAS Visual Investigator that mashes up functions across the SAS portfolio from Visual Statistics, Data Prep, Enterprise Miner, and other tools. That means that SAS can be more responsive to customer needs in the cloud by speeding introduction of new services. By the way, such mashing up fits well with SAS's roadmap for Viya, which is to expose the bulk of capabilities from the core portfolio under a more modern, web-based interface.
As for open source, as mentioned above, SAS interoperates with it, mostly through Viya. However, dealing the lack of perception about SAS and ML, SAS should start contributing to open source.
The logical place for SAS to get proactive with open source is ML and AI for a couple reasons. First, the lion's share of AI/ML innovation is already happening in the open source community. In deep learning, thought leaders Google and Amazon have thrown in TensorFlow and MXNet, respectively; for ML side, there's Spark MlLib, Scikit-Learn in the Python community, and the CRAN libraries of R, among others. And secondly, open source is a good place to get data scientists hooked because it's erased barriers to entry.
This doesn't mean that SAS should become an open source company. Its core differentiator is the underlying platform that manages the analytics lifecycle, not to mention its deep bench of analytic tools and capabilities; that doesn't make sense for open source. But for "gateway drugs," like ML, it provides the logical place for SAS to engage its future customers where they already are.