The state of AI in 2019: Breakthroughs in machine learning, natural language processing, games, and knowledge graphs
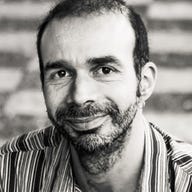
AI is one of the most rapidly growing domains today. Keeping track and taking stock of AI requires not just constant attention, but also the ability to dissect and evaluate across a number of dimensions. This is exactly what Air Street Capital and RAAIS founder Nathan Benaich and AI angel investor, and UCL IIPP Visiting Professor Ian Hogarth have done.
Also: AI created through neuroscienceZDNet YouTube
In the aptly titled State of AI Report 2019 published on June 28, Benaich and Hogarth embark on a 136-slide long journey on all things AI: technology breakthroughs and their capabilities, supply, demand and concentration of talent working in the field, large platforms, financing and areas of application for AI-driven innovation today and tomorrow, special sections on the politics of AI, and AI in China.
Benaich and Hogarth are more than venture capitalists: They both have extensive AI background, having worked on a number of AI initiatives, from research to startups. Furthermore, they draw on the expertise of prominent figures such as Google AI Researcher and lead of Keras Deep Learning framework François Chollet, VC and AI thought leader Kai-Fu Lee, and Facebook AI Researcher Sebastian Riedel.
This collective work is the accumulation of rich expertise, experience and knowledge. Spotting and reading the report, we reached out to Benaich, with whom we had an extensive Q&A session. We distill the report, and Benaich's insights, in a series of two posts, starting with technology breakthroughs and capabilities, and moving to their implications and the politics of AI.
Unpacking AI
If you're into AI, chances are this is not the first AI report you've come across. Many people are familiar with FirstMark's Data and AI landscape, compiled by Matt Turck and Lisa Xu, and The State of AI: Divergence by MMC Ventures. Updates in all three reports were released almost simultaneously. Although this may lead to some confusion, as obviously there is overlap, there also is differentiation in terms of content as well as approach and format.
FirstMark's report is more extensive in terms of listing players ranging from data infrastructure to AI. This is how that report has evolved over time, starting out as Big Data Landscape to become the data and AI landscape. The big data-to-AI evolution is a natural one, as we've pointed out in the past. MMC Ventures has a different point of view, as it's more abstract, potentially making it more suitable to CxOs. The reports have different scopes, and it's not a case of choosing sides -- each one has something to offer.
To begin with, we asked Benaich why they do this: Why do they share what is undoubtedly valuable knowledge, and put in the extra work for this, seemingly for free?
Benaich said they believe that AI will be a force multiplier on technological progress in our increasingly digital, data-driven world. This is because everything around us today, ranging from culture to consumer products, is a product of intelligence:
"We believe there is a growing need for accessible, yet detailed and accurate, information about the state of AI across several vectors (research, industry, talent, politics, and China). The purpose of our report is to drive an informed conversation about AI progress and its implications for the future."
The report lives up to Benaich's goals as set in his reply. The first 40 pages of the report, which comes in the shape of a slide deck, are focused on progress in AI research -- technology breakthroughs and their capabilities. Key areas covered are reinforcement learning, applications in games and future directions, natural language processing breakthroughs, deep learning in medicine, and AutoML.
Also: Is a space alien AI visiting us? ZDNet YouTube
Reinforcement learning, games, and learning in the real world
Reinforcement learning is an area of machine learning that has received lots of attention from researchers over the past decade. Benaich and Hogarth define it as being concerned with "software agents that learn goal-oriented behavior by trial and error in an environment that provides rewards or penalties in response to the agent's actions (called a "policy") towards achieving that goal."
A good chunk of the progress made in RL has to do with training AI to play games, equaling or surpassing human performance. StarCraft II, Quake III Arena and Montezuma's revenge are just some of those games.
More important than the sensationalist aspect of "AI beats humans", however, are the methods through which RL may reach such outcomes: Play driven learning, simulation and real-world combination, and curiosity-driven exploration. Can we train AI by playing games?
As children, we acquire complex skills and behaviors by learning and practicing diverse strategies and behaviors in a low-risk fashion, i.e., play time. Researchers used the concept of supervised play to endow robots with control skills that are more robust to perturbations compared to training using expert skill-supervised demonstrations.
OpenAI used simulation to train a robot to shuffle physical objects with impressive dexterity. The system used computer vision to predict the object pose given three camera images and then used RL to learn the next action based on fingertip positions and the object's pose.
In RL, agents learn tasks by trial and error. They must balance exploration (trying new behaviors) with exploitation (repeating behaviors that work). In the real world, rewards are difficult to explicitly encode. A promising solution is to: Store an RL agent's observations of its environment in memory; and reward it for reaching observations that are "not in memory".
The above, cited from the report, seem like equally good and natural ideas. Could leveraging those be the way forward for AI? Benaich noted that games are a fertile sandbox for training, evaluating and improving upon various learning algorithms, but also offered some words of skepticism:
"Data that is generated in a virtual environment is often less expensive and more widely available, which is great for experimentation. What's more, game environments can be made more or less complex depending on the goals of the experiment in model development.
However, the majority of games do not accurately mimic the real world and its plentiful nuances. This means that they're a great place to start, but not an end in themselves."
Also: Understanding AI in supply chain ZDNet YouTube
Natural language processing and common sense reasoning
As Benaich and Hogarth note, it's been a big year in natural language processing (NLP): Google AI's BERT and Transformer; Allen Institute's ELMo; OpenAI's Transformer, Ruder and Howard's ULMFiT, and Microsoft's MT-DNN demonstrated that pre-trained language models can substantially improve performance on a variety of NLP tasks.
Pretraining models to learn high- and low-level features has been transformative in computer vision, largely via ImageNet. ImageNet is a dataset that contains more than 20,000 categories. A typical category, such as "balloon" or "strawberry," consists of several hundred annotated images.
Since 2010, the ImageNet project runs an annual software contest, the ImageNet Large Scale Visual Recognition Challenge (ILSVRC), where software programs compete to correctly classify and detect objects and scenes. The challenge uses a "trimmed" list of one thousand non-overlapping classes and has been a driving force for the gradual refinement in computer vision.
ImageNet is a curated set of training data for computer vision which has helped progress the state of the art. Image: Nvidia
In the last year there have been similar empirical breakthroughs in pre-training language models on large text corpora to learn high- and low-level language features. Unlike ImageNet, these language models are typically trained on very large amounts of publicly available, i.e. unlabeled text from the web.
This method could be further scaled up to generate gains in NLP tasks and unlock many new commercial applications in the same way that transfer learning from ImageNet has driven more industrial uses of computer vision.
Benaich and Hogarth highlight the GLUE competition, which provides a single benchmark for evaluating NLP systems at a range of tasks spanning logic, common sense understanding, and lexical semantics.
As a demonstration of how quickly progress is being made in NLP, they go on to add, the state-of-the art has increased from a GLUE score of 69 to 88 over 13 months. The human baseline level is 87. Progress was so much faster than anticipated that a new benchmark SuperGLUE has already been introduced.
Language, however, is special when it comes to human cognition. It is associated with common sense reasoning. Progress in common sense reasoning has been made, too. We have seen how recent research from Salesforce advanced the state of the art by 10%.
Researchers from NYU have shown that by generatively training on a dataset's inferential knowledge, neural models can acquire simple common sense capabilities and reason about previously unseen events. This approach extends work such as the Cyc knowledge base project that began in the 80s and is called the world's longest AI project.
Also: Trends within machine learning and AIZDNet YouTube
The way forward: Combining deep learning and domain knowledge?
We asked Benaich for his take on approaches combining deep learning and domain knowledge for NLP, as this is something experts such as Yandex's David Talbot think is a promising direction. Benaich concurred that combining deep learning and domain knowledge is a fruitful avenue of exploration:
"Especially when the goal of an AI project is to solve a real-world problem vs. building a general intelligence agent that should learn to solve a talk tabula rasa. Domain knowledge can effectively help a deep learning system bootstrap its knowledge of the problem by encoding primitives instead of forcing the model to learn these from scratch using (potentially expensive and scarce) data."
Benaich also noted the importance of knowledge graphs for common sense reasoning on NLP tasks. Cyc is a well-known knowledge graph, or knowledge base, as the original terminology went. He also went on to add, however, that common sense reasoning is unlikely to be solved from text as the only modality.
Other highlights included in the report are federated learning, advances in data privacy by TensorFlow Privacy from Google and TF-Encrypted from Dropout Labs, and a number of use cases for deep learning in medicine. These include science-fiction-like feats such as decoding thoughts from brain waves and restoring limb control for the disabled.
It would take a very deep dive to unpack everything included in the report, such as progress in AutoML, GANs, and deep fakes for speech synthesis -- something which we predicted a few years back. Just scanning through the report takes a while, but has a lot to offer.
We will, however, continue with part two of the Q&A with Benaich, including AI chips, robotic process automation, autonomous vehicles, and the geopolitics of AI.