Advice for technologists on promoting AI ethics

Ethics looms as a vexing issue when it comes to artificial intelligence (AI). Where does AI bias spring from, especially when it's unintentional? Are companies paying enough attention to it as they plunge full-force into AI development and deployment? Are they doing anything about it? Do they even know what to do about it?
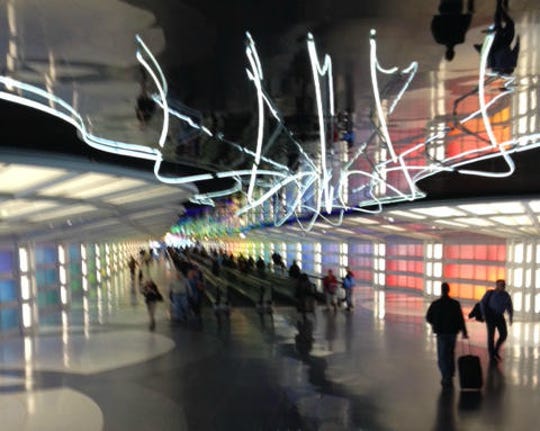
Wringing bias and unintended consequences out of AI is making its way into the job descriptions of technology managers and professionals, especially as business leaders turn to them for guidance and judgement. The drive to ethical AI means an increased role for technologists in the business, as described in a study of 1,580 executives and 4,400 consumers from the Capgemini Research Institute. The survey was able to make direct connections between AI ethics and business growth: if consumers sense a company is employing AI ethically, they'll keep coming back; it they sense unethical AI practices, their business is gone.
Competitive pressure is the reason businesses are pushing AI to its limits and risking crossing ethical lines. "The pressure to implement AI is fueling ethical issues," the Capgemini authors, led by Anne-Laure Thieullent, managing director of Capgemini's Artificial Intelligence & Analytics Group, state. "When we asked executives why ethical issues resulting from AI are an increasing problem, the top-ranked reason was the pressure to implement AI." Thirty-four percent cited this pressure to stay ahead with AI trends.
Another one-third report ethical issues were not considered while constructing AI systems, the survey shows. Another 31% said their main issue was lack of people and resources. This is where IT managers and professionals can make the difference.
The Capgemini team identified the issues with which IT managers and professionals need to deal:
- "Lack of ethical AI code of conduct or ability to assess deviation from it
- Lack of relevant training for developers building AI systems
- Ethical issues were not considered when constructing AI systems
- Pressure to urgently implement AI without adequately addressing ethical issues
- Lack of resources (funds, people, technology) dedicated to ethical AI systems"
Thieullent and her co-authors have advice for IT managers and professionals taking a leadership role in terms of AI ethics:
Empower users with more control and the ability to seek recourse: "This means building policies and processes where users can ask for explanations of AI-based decisions."
Make AI systems transparent and understandable to gain users' trust: "The teams developing the systems should provide the documentation and information to explain, in simple terms, how certain AI-based decisions are reached and how they affect an individual. These teams also need to document processes for data sets as well as the decision-making systems."
Practice good data management and mitigate potential biases in data: "While general management will be
responsible for setting good data management practices, it falls on the data engineering and data science and AI teams to ensure those practices are followed through. These teams should incorporate 'privacy-by-design' principles in the design and build phase and ensure robustness, repeatability, and auditability of the entire data cycle (raw data, training data, test data, etc.)."
As part of this, IT managers need to "check for accuracy, quality, robustness, and potential biases, including detection of under-represented minorities or events/patterns," as well as "build adequate data labeling practices and review periodically, store responsibly, so that it is made available for audits and repeatability assessments."
Keep close scrutiny on datasets: "Focus on ensuring that existing datasets do not create or reinforce existing biases. For example, identifying existing biases in the dataset through use of existing AI tools or through specific checks in statistical patterns of datasets." This also includes "exploring and deploying systems to check for and correct existing biases in the dataset before developing algorithms," and "conducting sufficient pre-release trials and post-release monitoring to identify, regulate, and mitigate any existing biases."
Use technology tools to build ethics in AI: "One of the problems faced by those implementing AI is the black-box nature of deep learning and neural networks. This makes it difficult to build transparency and check for biases." Increasingly, some companies are deploying tech and building platforms which help tackle this. Thieullent and her co-authors point to encouraging developments in the market, such as IBM's AI OpenScale, open source tools, and solutions from AI startups that can provide more transparency and check for biases.
Create ethics governance structures and ensure accountability for AI systems: "Create clear roles and structures, assign ethical AI accountability to key people and teams and empower them." This can be accomplished by "adapting existing governance structures to build accountability within certain teams. For example, the existing ethics lead (e.g., the Chief Ethics Officer) in the organization could be entrusted with the responsibility of also looking into ethical issues in AI."
It's also important to assign "senior leaders who would be held accountable for ethical questions in AI." Thieullent and the Capgemini team also recommends "building internal and external committees responsible for deploying AI ethically, which are independent and therefore under no pressure to rush to AI deployment."
Build diverse teams to ensure sensitivity towards the full spectrum of ethical issues: "It is important to involve diverse teams. For example, organizations not only need to build more diverse data teams (in terms of gender or ethnicity), but also actively create inter-disciplinary teams of sociologists, behavioral scientists and UI/UX designers who can provide additional perspectives during AI design."