Why isn't AI helping us today with COVID-19?
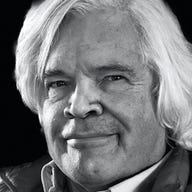
Wouldn't it be great if a medical diagnosis could be automated with machine learning and artificial intelligence? Skip waiting days or weeks for an appointment, then being asked questions with looking and poking. Just go online, get the questions from an AI, and then get a physical appointment if warranted.
That's the goal of medical automatic diagnosis (MAD). But like all ML/AI apps, models need training. Since we're dealing with humans, we can't just train on real doctor-patient interactions or let the AI agent misdiagnose real patients. Yet failing is essential to training.
Thus researchers are looking at developing a patient simulator to train ML models, using real doctor-patient dialogue records. But since the dialogues occur in person, the doctor is observing the patient and making unspoken observations that the dialogue fails to capture. The records don't capture the unasked and unanswered questions that an AI needs to train on.
The training problem
The massive gains in computer vision have been powered by the billions of photos placed online in the last 30 years. Teaching a machine to differentiate cats from dogs is aided by all the pictures of cats and dogs helpfully labeled with captions like "my dog".
But we've already run into training problems with humans when the corpus is not representative of the great diversity among people. Results can only be as good as the corpus an AI agent is trained on.
As AI technology expands to more esoteric realms, the problem of obtaining a sufficient training corpus will grow. A specific problem in medical AI is this: how can we usefully simulate patient symptoms?
Counterfactual inquiry
Simulating patient symptoms seems like it could backfire, but researchers at Sun Yat-sen University and UCLA have proposed a solution: a propensity-based patient simulator (PBPS).
The PBPS is itself a neural network trained to estimate the propensity of a patient to report a particular symptom. The PBPS answer is inserted into the training data to supplement the factual data from clinical records.
This isn't surprising if you think about it. How many times have you gone to the doctor, answered all questions, and NOT received a correct diagnosis? That's why insurance companies pay for second opinions.
The PBPS can use data from later clinical interactions -- a second opinion that includes more subtle symptoms -- to train the diagnostic AI to go deeper into the patient's experience. Unlike humans, an AI doesn't get tired, hurried, or forgetful, and can bring to bear millions of clinical interactions, far more than most physicians will ever have.
There's much more to the research, of course. But the bottom line is that they found this technique gave fast and reliable diagnosis without a burdensome number of questions.
The Storage Bits take
When I first looked at this strategy, it seemed dangerous to rely on synthetic data in the training process. But as I considered that the PBPS relies on a deeper level of clinical data -- going beyond initial interviews -- I saw that it could give much better training to medical AI systems.
I also reflected on the fact that so much AI research is coming out of China today. Partly that reflects their government's focus on becoming the world leader in AI. But it also is an extension of the training problem: the need for a large corpus.
A country with 1.3 billion people has a significantly greater opportunity to amass large data sets for training than a country of 300 million. Given that by 2030 India will have the world's largest population, perhaps we should be looking to that country for future AI leadership.
Scale changes everything, especially in AI.
Comments welcome. I like automated checkouts, and I think I'd like an autodoc even more. What say you?