A day in the data science life: Salesforce's Dr. Shrestha Basu Mallick

If artificial intelligence is going to gain scale, it'll do it by blending into current processes and augmenting human knowhow (at least at first). The real trick will be blending the data science and art behind many processes and business deals.
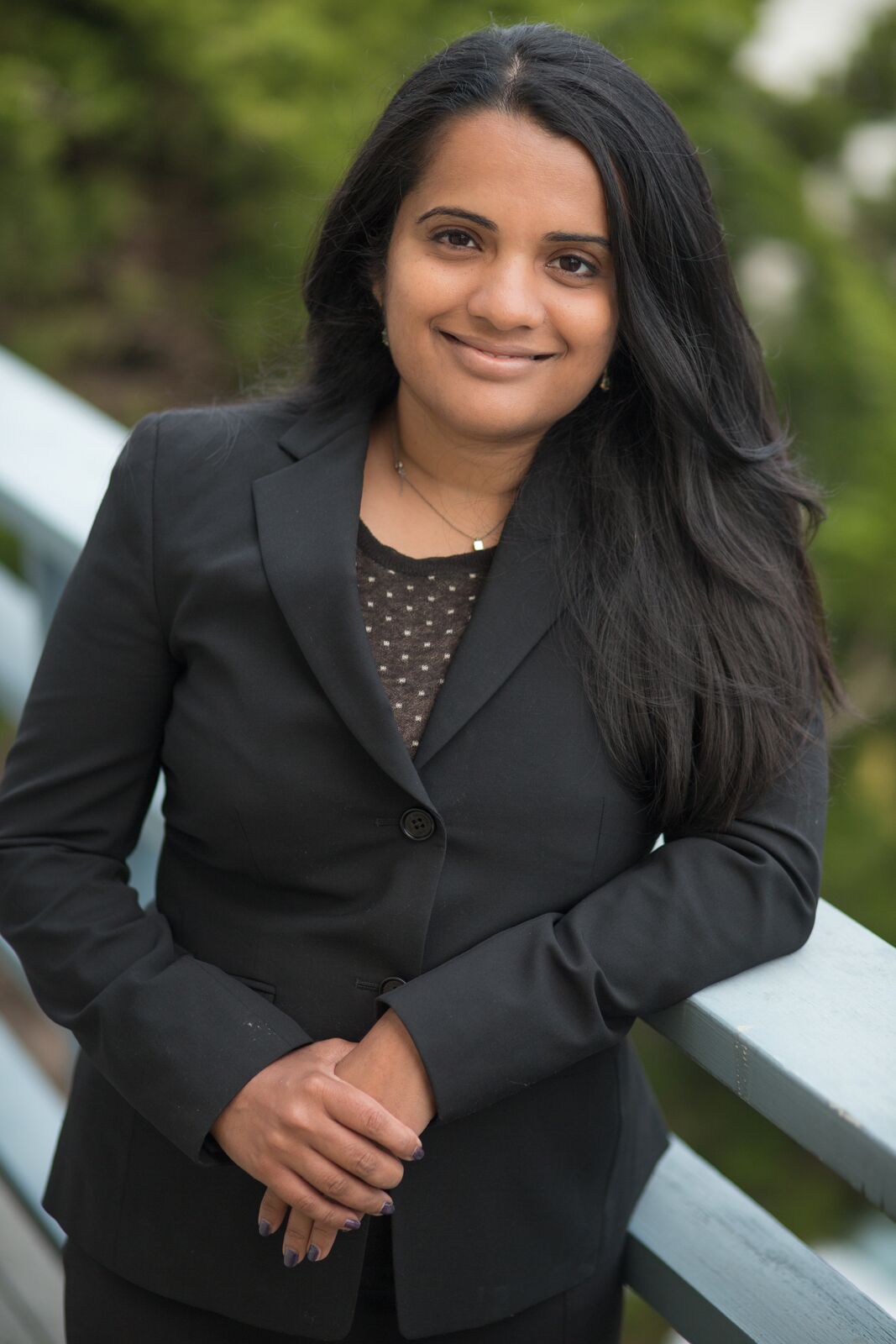
Dr. Shrestha Basu Mallick
With that in mind, ZDNet caught up with Dr. Shrestha Basu Mallick, a senior manager of pricing data science for Salesforce Analytics, to talk about her recent project to optimize pricing in sales deals. The project revolved around combining multiple Salesforce technologies--notably Einstein--to create a price optimization model. Discounting is a fact of sales life so enterprises need to get smarter about it.
Here's a recap of Mallick's project and a day in the life of a data scientist.
What was the gist of the project?
I partnered with my colleague Dan Boren (senior director of pricing and packaging strategy) to craft an AI-driven pricing solution which is designed using existing products: CPQ, Einstein Discovery and Einstein Analytics. Pricing is a complex skill that takes years of experience to master. Historically, pricing has mostly been quantity-based without taking into account all the attributes that make a deal unique. This pricing solution provides both proactive and reactive guidance, and is customized to the specifics of the deal and takes into account 50+ attributes. It also equips managers with pricing performance management dashboards which lets them track how their deals are doing on the pricing front and which of their sales reps need pricing coaching.
What are the challenges with price optimization and delivering discounts at the right time?
Pricing is an incredibly complex science and art that takes years to master -- there are hundreds of variables to take into consideration and today's sales process moves faster than ever before. The best price does not always mean the highest price -- it means finding the right price point at which the customer feels that they are getting a good value while capturing that value for the vendor as well.
Sales reps are always juggling at least three priorities on any given deal: the customer's satisfaction in the value they're getting; the speed in which they close the deal; and the best price for the company. No human can effectively balance these priorities on their own keeping in mind the different variables -- and its especially difficult for newer sales reps who are just starting off their sales careers. Very few tools drive these pricing conversations effectively. It's equally difficult for sales managers, who have very little data-driven insight into the pricing stages of deals. They often end up focusing their efforts on the largest deals or the reps that ask for help, not the reps that need coaching.
What data is needed--third party and internal--to best optimize price?
The starting point should be internal data, including the number of existing seats the customer has, customer size, competitive factors, and segmentation such as region or industry. Special focus should be given to deals that have closed at good price points -- all else being same -- because these are the deals you want your AI-driven model to learn from. Once a model is built with internal CRM data, external data sets, such as macroeconomic variables and weather patterns,that are relevant to the pricing model can be layered in.
How do you decide what's the best use for AI vs. another technology?
For companies just getting into price optimization, starting with a rules-based model can yield results right away. These models would provide guidance based on pre-set rules derived from business constraints and best practices. A rules-based algorithm might determine whenever product = Sales Cloud and Competitor = X, target discount = 10 percent. A model created without pre-set rules would look at all the historical data for Sales Cloud and a specific competitor, figure out the patterns inherent in the data and then recommend a target discount.
The biggest factor in using AI would be the amount and quality of data available. In order to consider an AI-driven solution, a company needs to have the means of collecting well-structured data that captures all the relevant signals from the negotiation process, which can be used to train the models. Other factors to consider include: the speed at which business conditions change, the discounting spread on product lines, the enablement needed to make sales reps trust and use the AI solution, and the complexity of the sales process.
What are the variables to consider when creating a good model?
All the variables that could contribute to deal price should be considered. These include but are not limited to:
- Customer variables -- Examples include customer size, industry, and customer satisfaction
- Product variables -- Examples include product type, the weight that product carries in a larger deal, and the total number of other products involved in the deal
- Deal variables -- Examples include region, competitors and activities or actions that have been taken as part of the deal (meetings with c-suite, industry or company events, etc.)
Special emphasis must be made to include all of the actionable variables. These are actual levers that the sales rep can control and use in negotiation, such as quantity, contract length and payment terms. For example, a sales rep may be able to offer a higher discount if the contract extends from 12 months to 24 months.
There is clearly a shortage of data scientists. How does that shortage impact the ability to democratize AI usage?
There are simply not enough data scientists in the world to deliver on the projects required for every company. At Salesforce, we want to deliver artificial intelligence at scale, and make it accessible to everyone. We have done the heavy lifting that would normally require a number of data scientists, and removed the complexity in order to deliver seamless and scalable AI to Salesforce customers of all sizes.
TechRepublic: Cheat sheet: How to become a data scientist | Job description: Data scientist (Tech Pro Research) | How to build a data science team | Here come all the AI deployments; Now how do we manage AI?
How does AI account for the art involved with sales and pricing? How did you account for that art in your model?
The art of pricing comes from the human aspect of the sales reps' behavior. When my colleague Dan and I worked on building this pricing solution, we would often find that two deals, which were very similar in attributes, would have widely different pricing. We were able to see that the difference was coming not from the customer and product variables, but from the human beings involved on the deals -- the sales representative and the sales manager.
We discovered that layering on the psychographic and behavioral attributes -- such as the experience level of the sales rep, the pricing expertise of their manager, the sales reps' quota attainment -- enabled us to explain some of the variation. In other words, we found that certain sales reps were more adept at the art of pricing. Our challenge was how to capture this elusive trait and bring it into our AI-driven model.
One thing we did was to learn from the expert pricers who had a high win rate while still getting good margins. We interviewed these expert pricers and incorporated their pricing best practices into the model. We developed our AI model in such a way that when it provides guidance, it provides an "expert" target and a "good" target. The "expert" target is derived from the pricing patterns of the expert pricers -- the top 25th percentile. The "good" target is likely more attainable, a good benchmark for less experienced sales reps, and still a win for both the customer and the vendor.
We also learned that salespeople best at pricing used creative deal structuring to win deals as opposed to outright discounting; hence our model provides negotiation levers. It provides recommendations on how sales reps can use payment terms and contract duration creatively to give customers appropriate discounting while preserving deal margins.
For us this is the tip of the iceberg. We are exploring other techniques, such as marking confidence intervals that will tell sales reps how confident the model is in the recommendation. This will invite the sales team to use their judgment. We want this to be an augmented intelligence effort -- the AI and the sales rep need to work together to come to the best price.
More:
- The great data science hope: Machine learning can cure your terrible data hygiene
- Science-based healthcare: How IoT and AI can help us make health decisions based on data not opinion
- Match Group's Tinder emerges as cash cow courtesy of data science, dynamic pricing, paying off tech debt
- IBM enhances Watson Data Platform, with an eye towards AI