3 ways AI is revolutionizing how health organizations serve patients. Can LLMs like ChatGPT help?
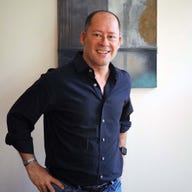
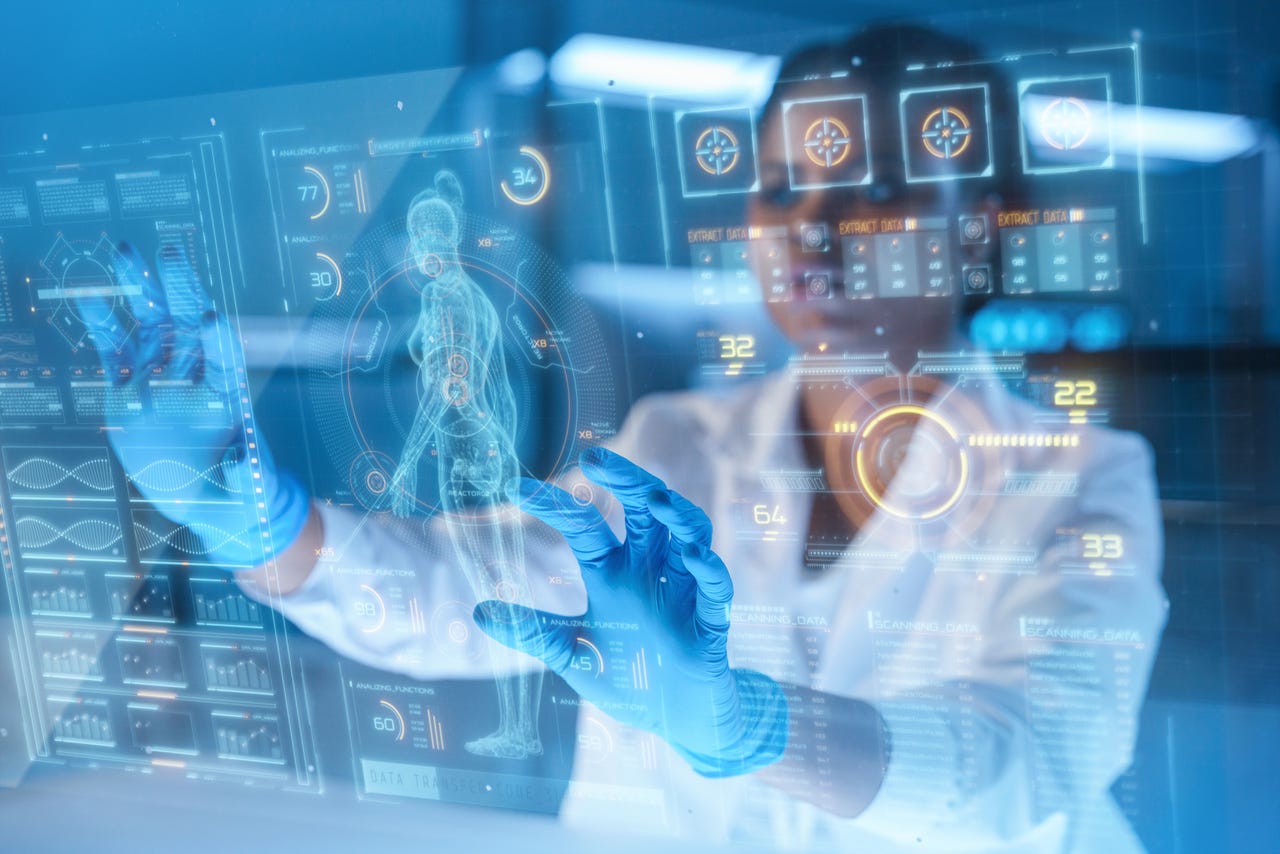
The digitization of health care has been a long time coming, with the practice of healing tied, for obvious reasons, to older, carefully vetted ways of doing things.
But increasingly, artificial intelligence in various forms is creeping into the clinic. Applications include in predictive analytics, smart prostheses, mobile diagnostics, and brain implants. Plus, with the emergence of large language models (LLMs) like ChatGPT, we explore whether that technology can assist in health care today.
While much of the work is in the form of pilot studies, it's clear AI will play a major role in shaping how health care is delivered in decades to come.
"Deep learning AI has finally gotten through this process of the technical infrastructure being in place, and the data being available to train it, after years of really hard work," said Jeremy Howard, co-founder of the AI research and education startup Fast.ai, and founder of the first company to apply deep learning to medicine, Enlitic.
After all that effort, "You should expect to see a hell of a lot more of it in the coming years," meaning, applied AI in medicine, said Howard in an interview with ZDNET.
Right now, the state of the art in applying AI to medicine consists of small-scale studies that employ a variety of very well-established machine learning forms of AI. Those programs have proven their worth over decades. They are finally being taken into the clinic and being applied to a variety of data, from real-time brain activity readings to electronic health records.
It's still a ways off for the newer kinds of machine learning AI, the stuff such as ChatGPT from OpenAI. That technology gets all the attention but it is too new to be reliable in the sensitive realm of the clinic.
Already, the use of machine learning has been transformative for the participating patients. Gert-Jan Oskam, a 40-year-old bicycle accident victim, was given a novel brain-computer interface that let him stand and walk again. He told the prestigious journal Nature the device has been "life-changing."
"Last week, there was something that needed to be painted and there was nobody to help me," said Oskam. "So, I took the walker and the paint, and I did it myself while I was standing."
1. Restoring functionality with AI
Among the most dramatic early wins for AI in health care are the successes, still small in number, with prostheses of various kinds to restore functionality to individuals with serious injuries.
Step aside, Elon Musk: the fabled "brain-computer interface," or BCI that Musk says he'll put into clinical trials has already been achieved by the Stanford team in a stunning example of using carefully developed machine learning forms of AI.
The fabled "brain-computer interface" touted by Elon Musk is already a reality. Researchers in Switzerland made a "digital bridge" between the brain's motion regions and "lumbosacral" region of the spine that translates intention into stimulation of the muscles to make legs move naturally.
The "digital bridge," developed by researchers at Stanford's mechanical engineering department, routes brain signals around a spinal impairment using sensors and wireless technology. The device restores walking to Oskam, who had almost no ability to walk after a spinal cord injury from a bicycle accident a decade prior.
Also: Google's MedPaLM emphasizes human clinicians in medical AI
Researchers Henri Lorach and team described in Nature in May how they implanted a "brain–spinal cord interface" in Oskam. He limited use of his legs following a five-month program of epidural electrical stimulation of the spinal cord, an ability to take some steps with the help of a walker.
Lorach and team implanted two devices, each consisting of 64 electrodes, on top of the parts of either hemisphere of the brain that are known to control movement. Those sensors gathered signals known as electrocorticograph, or, ECoG, that are linked to intentions to move. The ECoG signals were picked up wirelessly via a 3-D-printed headset worn by the patient, which is attached via a USB cable to a "base station" computer worn in a backpack.
The backpack computer decodes the ECoG patterns into commands that are then sent, wirelessly, to a third device, implanted on top of the "lumbosacral" region of the spine -- think of your lower back and tailbone. Called a "pulse generator," it turns the commands into electrical stimulation that "engages the muscles that mediate the intended movements."
The result is that the patient was able to "regain intuitive control over the movements of his legs, giving him the ability to stand, walk, climb stairs and even traverse complex terrains," reported Lorach and team. Videos accompanying the report show the patient getting up from a seated position and walking, in this case with the base station computer set on a walker instead of in a backpack.
It's important to realize this is not just a matter of brain sensor implants. The machine learning algorithms that Lorach and team have pioneered to interpret the brain signals for movement intention are a key element.
Using an approach known as an "online adaptive supervised learning algorithm," the program is tuned as the patient tries repeatedly to move first an on-screen avatar's limbs, and then an exoskeleton.
The software combines several threads of machine learning science, including a "mixture of experts," where different commands control different limbs, and then what's called a "Hidden Markov model," a kind of algorithm in use for decades. The computing of movement intention is all done in real time, as the patient moves. More details on the algorithm can be found in a prior paper from the team from 2022.
Scientists are finding out lots of other ways they can gather signals from the brain and decode them via machine learning to restore functionality.
Also: Amazon AWS rolls out HealthScribe to transcribe doctors' conversations
Last month, researchers Sean Metzger and team at the Department of Neurological Surgery at the University of California at San Francisco related in Nature a speech decoder that generated a voice for a 47-year-old stroke victim rendered mute nearly twenty years earlier.
The so-called multimodal speech decoder also used an implanted ECoG detector to decode "intended sentences" from signals in areas of the brain's sensorimotor cortex. That area is responsible for "attempted movements of the lips, tongue, and jaw." The signals are decoded into what the researchers call "vocal-tract representations" that can then be turned into multiple kinds of output: text on a screen, generated audio of spoken words, and movements of an avatar speaking.
The so-called multimodal speech decoder also used an implanted ECoG detector to decode "intended sentences" from signals in areas of the brain's sensorimotor cortex, using a tried and true type of machine learning algorithm, known as a "bidirectional recurrent neural network" or "RNN," a program long used for modeling times-series data.
The key, again, is not just sensors but also machine learning algorithms. The signals from the ECoG of vocal tract attempts were fed into another tried-and-true type of machine learning algorithm, known as a "bidirectional recurrent neural network" or "RNN," a program long used for modeling time-series data, data that measures the same variables at different points in time to spot trends. The RNN was first tuned to anticipate several canned sentences the patient was trying to speak (which were shown to the patient on a screen) -- that's a form of correlating the brain activity to a defined set of output.
However, after two weeks of such training, the RNN was producing spontaneous text output from unprompted sentences the patient was trying to utter. The program was able to generate as many as 78 words per minute. That was multiple times faster than the 14 words per minute the patient had been able to produce with their existing assistive device, a head-tracking apparatus where the patient had to nod at words on a screen, similar to what the late physicist Steven Hawking used.
Likewise, the RNN was able to be trained to interpret the ECoG to match a waveform, which could then drive a vocoder to generate speech. After two weeks of tuning the system with the patient, the "speech-neuroprosthetic system" they developed showed such impressive results that "we believe ... these results have surpassed an important threshold of performance, generalizability, and expressivity that could soon have practical benefits to people with speech loss," writes Metzger and team.
2. AI can make medical diagnostics portable
One of the chief stumbling blocks of medical diagnostics is that it requires patients to make a trip to a medical facility where tests can be administered using gigantic equipment and data manually examined by trained experts. But some new attempts at diagnostics are using machine learning forms of AI to take the matter out of the clinic.
Take sleep studies, which usually involve a seven-hour stay at a facility, hooked up to electrodes, and monitored throughout the night by staff. Could it instead be done at home, with a phone and a couple of stick-on patches?
A team at the Georgia Institute of Technology in Atlanta, Georgia, came up with wireless sleep patches made of silicone with embedded flexible circuitry, as reported recently in the prestigious journal Science. The patches make use of machine learning to measure the sleep data rather than having a live technician monitor the patient overnight.
The patches can be attached to the face by the patient at home, one on the forehead, one on the chin. They gather data for electroencephalograms (EEG) and electrooculograms (EOG), two kinds of measurements used to detect sleep apnea. The patches can be used for days at a time, unlike the gel-based electrodes used in a sleep clinic.
The patches transmit the EEG and EOG data via Bluetooth to a mobile device at bedside, and the mobile device uses what's called a "convolutional neural network" or a "CNN," a workhorse of machine learning. With a CNN, data represented as a spectrum of activity can be analyzed to detect sleep apnea.
Silicone face patches for at-home sleep study contain flexible circuitry so they're able to be worn during sleep. They connect wirelessly to a mobile device for data collection and analysis.
Lead author Shinjae Kwon and team found in trials with eight subjects that "the system's performance shows a high accuracy of 88.52%" in detecting obstructive sleep apnea. Moreover, the detection of the home patches and CNN showed "high agreement" with the 82.4% detection produced by the gold standard clinic-based sleep studies known as "polysomnography."
Also: Elon Musk says Neuralink brain implant is nearing human trials
Kwon and team expect to conduct a large-scale study of the system, and they are extending the sensors' capabilities to detect other indicators of sleep apnea, including blood oxygen saturation, carbon dioxide, and motions.
Given that the mobile device is sorting and sifting the CNN data, Kwok and team's effort points to a much larger trend: gathering and analyzing data in the field with AI on a mobile device. By automating the measurement that usually happens manually by skilled technicians, some diagnoses can be extended outside clinic walls.
A 2021 study by a team at Stanford University led by Chayakrit Krittanawong listed over a dozen examples of consumer-grade wearable health cardiac monitoring in addition to the Apple Watch's ECG monitoring. They include glasses from Israel's OrCam Technologies and shoe insoles from MEGAComfort.
Those wearables produce "biosignals," which the team defines as "physiological signals that can be continuously measured and monitored to provide information on electrical, chemical, and mechanical activity." Machine learning is a good candidate to aggregate, analyze, and interpret all that data.
Krittanawong and team relate small-scale studies of 100 subjects that used wearable patches with disposable sensors worn on the subject's chest. The sensor, powered by a disposable battery, detected multiple data from the subject's skin including electrocardiogram waveforms, skin temperature, and the subject's posture. All that data was continuously streamed via Bluetooth wireless to a mobile phone and then uploaded to the cloud for analysis using machine learning.
The patches were used as an alternative to implantable heart monitoring devices to predict the risk of hospitalization for heart failure. With cloud analysis, the patches and mobile devices performed with a level of sensitivity and specificity that matched traditional medical-grade implantable monitors.
3. Better predictive analytics for patient safety
Among the most widely deployed uses of AI to date in actual clinical settings is the use of predictive algorithms -- programs that can do things such as predict a patient's chance of hospital re-admission. They often use data from electronic health records (EHRs), since such records are a readily available store of vast amounts of data.
A study this year by the University of Utah School of Medicine found "broad adoption of AI in current clinical operations" based on EHR data, according to lead author David Classen and team.
However, those predictive tools have had questionable accuracy. A 2021 study of the Epic software for sepsis by the University of Michigan Medical School found extremely low rates of accuracy in the tool's prediction of sepsis, raising doubts about its utility in practice.
That suggests that researchers need better ways to deduce what an EHR is telling them with all that data.
In an example of the cutting edge of predictive analytics, scientists at the Stanford University School of Medicine gathered EHRs for 22,104 pairs of mothers and their newborns, linked the records, and came up with what they describe as greater ability to predict mortality in premature births, the leading cause of death of children under 5.
The work by Davide de Francesco and team, published February in Science Translational Medicine, used features contained in the mother's health records to predict neonatal outcomes. The main objective is to get a more precise picture of infant mortality than the handful of features typically used, known as the "Appearance, Pulse, Grimace, Activity, and Respiration," or Apgar, score at the time of birth/delivery.
Instead, the researchers fed multiple data points from multiple visits by the mother prior to delivery into another time-tested type of machine learning algorithm, called a "long-short-term memory" or "LSTM," used, like RNNs, to piece together time-series data. The LSTM was trained to correlate codes for things such as maternal procedures, medications, and observations leading up to birth with the conditions in the infant's neonatal medical record, such as hypotension or sepsis.
The authors found a significant increase in the ability to predict the reported outcomes of the infant versus the traditional risk assessment.
Specifically, the LSTM program could generalize heightened risk from some maternal conditions. "Among these codes strongly associated with neonatal outcomes were […] opioid dependence in remission, fetal-maternal hemorrhage, various congenital heart diseases," they relate.
What's more, they could also assert some things that protect a premature newborn in the weeks and months that follow birth.
"Notable laboratory measurements that suggest a protective association against neonatal outcomes include serum albumin, serum protein, platelets, basophils, lymphocytes, and eosinophils," they write. "These data suggest that there is interplay between the maternal immune system at one week before delivery and the relative health of the fetus that carries forward into the neonatal period and beyond."
The Stanford work suggests that as more sophisticated deep learning models take over from relatively primitive predictive systems, there's lots more information just sitting there, waiting to be decrypted.
Large language models (LLMs) in health care?
The machine learning AI methods discussed above -- RNNs, CNNs, LSTMs, and Hidden Markov models -- are all fairly well-established AI approaches that have been around for decades. The novelty is that they are being deployed now with new, greater levels of sophistication, and with new data.
But what about the really new algorithms in deep learning, the "generative AI" that is all the rage, such as OpenAI's ChatGPT?
It's very, very early days for generative AI in medicine and health care. AI in the form of large language models is only gradually entering the field in pilot studies, held back by concerns over things such as the "hallucinations" of language models, meaning their propensity to assert false information.
In fact, a study by Weill Cornell Medicine in August reported that "LLMs could be susceptible to generating factually inconsistent summaries and making overly convincing or uncertain statements, leading to potential harm due to misinformation."
ChatGPT's creator, OpenAI, has in fact told the journal Nature Medicine, that "its models should not be used for medical diagnostics, to triage or to manage life-threatening issues."
The risks and the ethical issues of generative AI mean many regulatory hurdles lie ahead.
In July, Scientists Bertalan Meskó of Hungary's The Medical Futurist Institute, and Eric Topol of the Scripps Research Translational Institute, wrote an overview paper in which they observed that "LLMs offer tremendous promise for the future of healthcare, but their use also entails risks and ethical challenges."
Meskó and Topol predict that regulators will "create a new regulatory category for LLMs as those are distinctively different from AI-based medical technologies that have gone through regulation already."
"It certainly appears to be extremely useful," says Jeremy Howard of generative AI, "but very difficult to deploy in a rigorous way that fits into the current constraints and processes in the US medical system."
Howard predicts that despite shortcomings, generative AI may have value in filling the medical skills gap.
"Most of the population of the world does not have access to nearly enough doctors," he said. "It may come down to, Would you like a community health worker with six months of training in effectively utilizing this AI system versus nothing at all?"