A startling thing happened when a neural net got to choose its own neurons
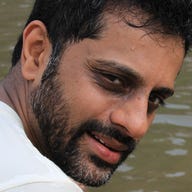

There was a time when purebred dogs were status symbols. Owners would crow about their German Shepherd's or Cocker Spaniel's impeccable pedigrees, sometimes boasting lineages that tread a single line within the same family for many generations.
This attraction to purity was heightened during Hitler's Nazi Germany, when 'contaminated' lineages for German Shepherds were abhorred. However, it was during the Victorian era of the 1800s that people inbred dogs relentlessly to the point where all the breeds we see now -- and their afflictions, such as hip and other joint issues -- are a result of that inbreeding process.
Also: Train AI models with your own data to mitigate risks
Today, the emphasis on purity has shifted and we continue to learn more about genetics, specifically about how inbreeding is the fastest way to acquiring debilitating health issues and unstable temperaments.
Now, the mutt rules. We know the greater the diversity of environments, the greater the diversity of organisms that emerge after adapting to them -- and ultimately the greater the stability of the world we live in.
In the modern world, more so than any period in modern human history, diverse gene pools are sought after, especially as unstable environments and climate change issues will require hardier species. This procecss by itself, as a law of the Earth, is not so hard to understand.
Also: Implementing AI into software engineering? Here's everything you need to know
But what about if a machine exhibits the same ability to perform better under a more diverse engineering setup?
Moreover, what if the machine actually chooses diversity after being allowed to fashion its own insides -- and its choices result in an unparalleled increase in processor speed?
Meta-learning for AI
This question was put to the test by a pathbreaking experiment undertaken by a team of researchers (Anshul Choudhary, Anil Radhakrishnan, John F. Lindner, William Ditto, and Sudeshna Sinha) from a range of institutions (North Carolina State, Indian Institute of Science, Mohali, College of Wooster). Their aim was to test the kind of operational choices advanced AIs, such as neural nets, would make when left to their own devices.
"We created a test system with a non-human intelligence, an artificial intelligence (AI), to see if the AI would choose diversity over the lack of diversity and if its choice would improve the performance of the AI," says paper co-author William Ditto, professor of physics at NC State and director of NC State's Nonlinear Artificial Intelligence Laboratory (NAIL).
Also: AI safety and bias: Untangling the complex chain of AI training
"Our real brains have more than one type of neuron," says Ditto, extrapolating further about the experiment, which is beginning to redefine how we look at neurological diversity in machines and the relationship to performance.
"So we gave our AI the ability to look inward and decide whether it needed to modify the composition of its neural network. Essentially, we gave it the control knob for its own brain. So it can solve the problem, look at the result, and change the type and mixture of artificial neurons until it finds the most advantageous one. It's meta-learning for AI."
Diversity is an evolutionary necessity for survival and peak performance, including in machines.
A neural net -- which is at the heart of most conventional, advanced AIs -- mimics the way our brains work. Just as our brains send and receive electrical impulses that hinge on the strength of their connections, so do neural networks, by adjusting numerical weights and biases when they are getting trained.
As a neural net undergoes training and tries to, for example, identify what buses look like by plowing through a large collection of bus photos, the network adjusts its numerical weights and balances itself as it negotiates right and wrong bus images.
Also: These are my 5 favorite AI tools for work
The strength of the connections between these neurons fluctuates during the training process, but they will essentially remain locked when it comes to their composition.
That's until the scientists gave the neural net the freedom to activate itself -- and then something remarkable happened.
Choosing diversity for peak performance
First, the network selected completely different or heterogeneous, non-linear neuron arrangements.
In other words, the system chose diversity over sameness in a process called 'learned diversity'.
Also: GPT-3.5 vs GPT-4: Is ChatGPT Plus worth its subscription fee?
Then, it turned out that the self-selecting, heteregonous neural net also outperformed the homogeneous one with the same training.
When the team asked AI to perform a 'standard numerical classifying exercise', the self-selecting, diverse AI exercise trounced its homogeneous sibling by 70% to 57% in terms of accuracy.
Ditto says the diverse AI can be as many as 10 times more accurate than a conventional neural net.
"We have shown that if you give an AI the ability to look inward and learn how it learns, it will change its internal structure -- the structure of its artificial neurons -- to embrace diversity and improve its ability to learn and solve problems efficiently and more accurately," says Ditto.
He added that as the problems become more complex and chaotic, the performance of the diverse AI neural net actually improves with time compared to the non-diverse AI.
Also: I spent a weekend with Amazon's free AI courses, and highly recommend you do too
According to the scientists, this learned diversity can even boost the performance of existing physics-informed AIs, such as Hamiltonian neural networks.
As AI gets deployed in almost every application -- many that are involved in life-and-death functions, such as aircrafts and autonomous vehicles -- we will need systems that are far more robust and quick.
A diverse evolutionary trajectory has made humans, animals, plants, and pretty much everything that has survived on Earth until now a success story -- and it also seems to separate the winners from losers in the machine world.