AI: The view from the Chief Data Science Office
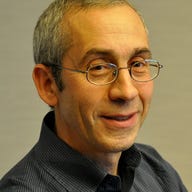
During a briefing with Kimberly Nevala, director of business strategies for SAS this afternoon, we facetiously posed the question of why they were wasting their time engaging with clients about artificial intelligence (AI). The topic of her talk last week at Strata on rationalizing risk with AI and ML struck a chord with us. Navala's message was that understanding what your models can and cannot do is key to the getting AI to succeed in your business, with her presentation outlining how to quantify your confidence levels in AI and ML models.
Featured
One of our most-read posts was the one a few months ago about the importance of not forgetting people and process when running AI projects. Over the past few months, we had the chance to speak at depth with over a dozen senior analytics and data science executives to get a better handle on managing the people and process side of AI projects. In full disclosure, this was a survey cosponsored by Ovum and Dataiku.
Also: Cheat sheet: How to become a data scientist TechRepublic
We targeted early adopter organizations that were well ahead of the curve with dozens to hundreds of projects in production. Given that each organization had dozens of data scientists or more on their staffs, the insights we received reflected over a century of staff years of experience. By far, the brunt of their AI projects was in machine learning.
We wanted to know, what was the impetus for AI, what was the criteria for utilizing AI, how do you staff and manage projects. But probably the most interesting question was how AI projects differed from more traditional data science.
As we've noted, you can't do AI projects without the data science. Not all data science projects require AI. For instance, if a customer segmentation model for a highly stable market, such as home heating oil deliveries, probably doesn't require a lot of machine learning if you have a neighborhood with a stable housing stock and demographics. But if you are trying to stay a step ahead of cyber attacks, machine learning or deep learning models may be necessary because of the constantly morphing threat.
Another core assumption with AI is the central role, not only of models, but data. And because AI models are extremely hungry for data, errors in data set selection or data quality can readily snowball. If getting the data right is important for analytics, it's even righter for AI models.
So should the impetus for AI start from the top down, or is it more effective for ideas to percolate up from the trenches? Given the makeup of the survey group, it wasn't surprising that in most cases, the inspiration for AI came from the C suite. But that doesn't mean that CEO mandates are the only way to go.
Also: The best programming language for data science and machine learning
For instance, the marketing department of a cable television provider whose business morphed through acquisition to include broadcast networks and studio production realized that the relationship to the customer was changing. And so they looked at prescriptive analytics as a means for helping master the new relationships and preserve loyalty as the relationship expanded from connectivity to content provider. They pioneered the use of AI in that organization.
When to employ AI? The answers were not surprising. In most cases, it was for business problems that were either too complex for humans, alone, to get their arms around and/or for problem domains that are constantly moving targets -- such as online gaming market that has been disrupted by a new offering that expands the addressable audience. AI was also the best answer when problem domains are highly dynamic, where relying on people to alter the models would prove too time consuming, lie with the cybersecurity example mentioned above. AI would also be the right solution where prescriptive approaches could provide solutions that were previously elusive such as optimizing maintaining plant equipment; guiding farmers on where, when, and how much to water and fertilize; or what to do to prevent a customer from churning.
What about data science teams? Should they be centrally based or imbedded in the operating units? The verdict was unanimous here. "It is hard for data scientists to understand the business if they live in a bubble," was a common refrain. But this was where the biggest disconnect with reality occurred. With data scientists in scarce supply, they are going to live where the high paying jobs and the talent lives, and that's more likely to be closer to headquarters than out in the boondocks.
That's where the data scientist we interviewed from a regional center of excellence for a global insurer laid out the narrative. The CoE's chief mission was knowledge transfer. We also foresaw a transition where CoEs morph into internal consulting organizations that are more hands-on in taking on projects and are actively training the trainer to either put themselves out of business or make local units more self-reliant.
Also: The AI, machine learning, and data science conundrum: Who will manage the algorithms?
But let's cut to the chase. If AI projects require data science, do they differ from data science projects? The feedback we received was all over the map. Some stated that development tasks are similar but production phase is different, or that AI projects often involve unknown outcomes (but hopefully, that's not the case for self-driving cars).
The biggest differentiator between AI and traditional data science projects is that unlike traditional models, AI models are dynamic. Their appetite for data is constant, and so you have the challenges of model and data drift that can either throw a project off target, or take it in different directions because reality has changed. Everything becomes a moving target, and so you need to manage for change. That's a lot different from change impact management -- the process is too heavyweight to be invoked every time a model changes.
The audit trails for tracking models are currently imperfect, but the best practice we uncovered was time stamping data. Speaking before a gathering of analysts earlier this year, Dr. Jim Goodnight of SAS expressed his concern about AI model accountability. Models cannot yet explain themselves. Maybe at some point we could automate the tracking of models, but for now, people must play front and center in tracking the inputs and outputs against expectations.